Landslide Risk Evaluation in Shenzhen Based on Stacking Ensemble Learning and InSAR
IEEE J. Sel. Top. Appl. Earth Obs. Remote. Sens.(2023)
摘要
Construction activities of accelerated urbanization in Shenzhen have increased the landslide risk area, which has intensified the potential threat to human and natural environment. However, the risk of landslides in Shenzhen is poorly evaluated. In this article, a landslide risk evaluation (LRE) model is constructed using landslide susceptibility map (LSM) and landslide vulnerability. In the experiment, a stacking ensemble learning (SEL) model is constructed based on convolutional neural network (CNN), multilayer perceptron, gated recurrent unit (GRU), and support vector machine regression to generate LSM by using topography, geology, human engineering activities, time-series precipitation, and time-series normalized difference vegetation index. Road network, building distribution density and annual average precipitation data are used to evaluate landslide vulnerability based on entropy weight method. In this article, multiple statistical indicators are used to evaluate the performance of the LSM model, and Interferometric Synthetic Aperture Radar (InSAR) deformation data are utilized to verify the LRE results in Shenzhen. The results show that the SEL method has more refined results for LSM, with a best overall evaluation accuracy, especially in the receiver operating characteristic curve, where the accuracy is improved by nearly 8%. In LRE of Shenzhen, very high, high, moderate, low, and very low risk areas account for 0.283%, 0.451%, 0.859%, 36.890%, and 61.517%, respectively. In most of very high-risk area, InSAR deformation results show a clear concentrated deformation trend with a large deformation rate. Research results can provide technical and data support for landslide disaster prevention in Shenzhen.
更多查看译文
关键词
Terrain factors,Precipitation,Hazards,Spatial resolution,Convolutional neural networks,Soil,Monitoring,Convolutional neural network (CNN),gated recurrent unit (GRU),landslide susceptibility,Shenzhen city,stacking ensemble learning
AI 理解论文
溯源树
样例
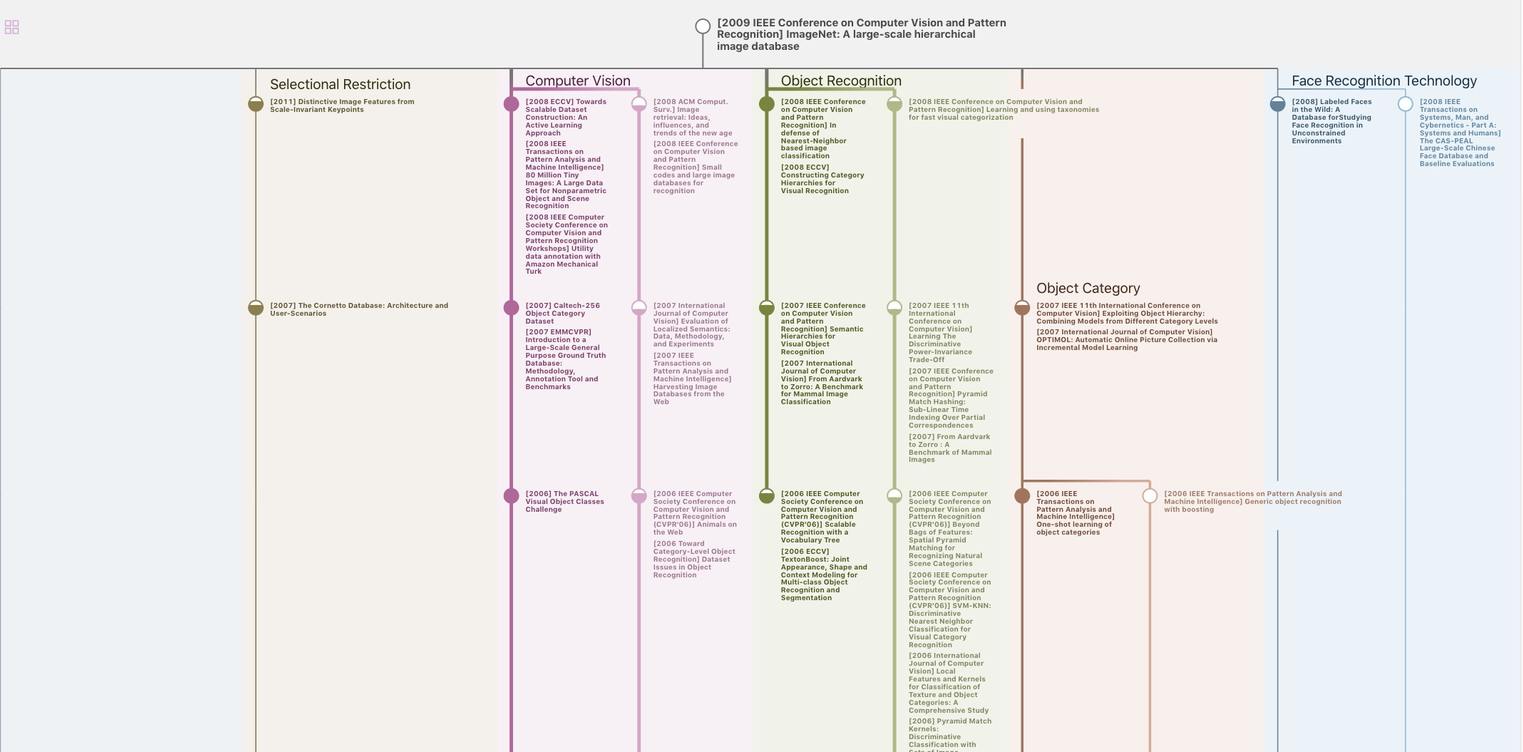
生成溯源树,研究论文发展脉络
Chat Paper
正在生成论文摘要