Approximate Optimal Indirect Regulation of an Unknown Agent With a Lyapunov-Based Deep Neural Network.
IEEE Control. Syst. Lett.(2023)
摘要
An approximate optimal policy is developed for a pursuing agent to indirectly regulate an evading agent coupled by an unknown interaction dynamic. Approximate dynamic programming is used to design a controller for the pursuing agent to optimally influence the evading agent to a goal location. Since the interaction dynamic between the agents is unknown, integral concurrent learning is used to update a Lyapunov-based deep neural network to facilitate sustained learning and system identification. A Lyapunov-based stability analysis is used to show uniformly ultimately bounded convergence. Simulation results demonstrate the performance of the developed method.
更多查看译文
关键词
Deep neural networks,reinforcement learning,adaptive control,Lyapunov methods,nonlinear control systems
AI 理解论文
溯源树
样例
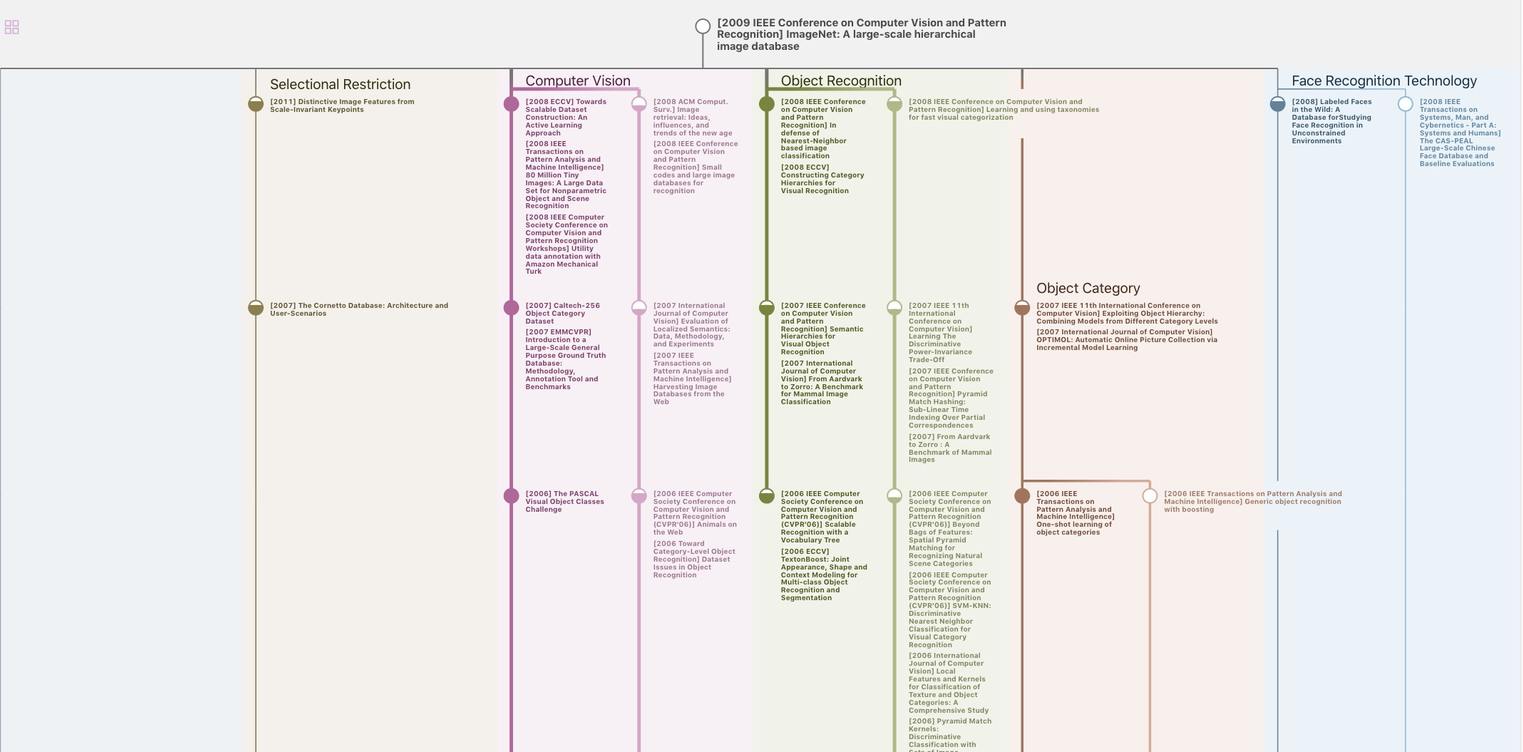
生成溯源树,研究论文发展脉络
Chat Paper
正在生成论文摘要