VPiP: Values Packing in Paillier for Communication Efficient Oblivious Linear Computations.
IEEE Trans. Inf. Forensics Secur.(2023)
摘要
The technique of packing multiple values into one message without losing homomorphic computation properties is the main workhorse that drives many exciting advances in applying lattice-based homomorphic encryption schemes to privacy-preserving Machine-Learning-as-a-Service (MLaaS). However, this technique does not directly work for the classic Paillier homomorphic encryption scheme, limiting the use of the Paillier scheme in the privacy-preserving MLaaS. To enrich the applications of Paillier in privacy-preserving MLaaS, we present a set of new methods for efficient linear computations over packed values under the Paillier scheme, such as vector multiplication, matrix multiplication, and convolutional calculation between ciphertexts and plaintexts. Different from the packing methods of lattice-based schemes, the Paillier packing method naturally allows higher packing capability for values in lower bitlength. This property can significantly benefit privacy-preserving MLaaS, as the values of user inputs and parameters of machine learning models are often quantized into low bits (e.g., 1-8 bits). We conduct comparisons based on different linear computation tasks, the proposed methods under the Paillier scheme clearly outperform the state-of-the-art in terms of communication and computational efficiency, especially in realistic scenarios. For example, compared to one of the recent arts CrypTFlow2 (Rathee et al., 2020), the communication cost of our solution can be 21.7x smaller at best. Thanks to the reduction of communication cost, the runtime can be 2.46x faster than CrypTFlow2 at the mediancountry-speed of current global mobile broadband.
更多查看译文
关键词
paillier,communication efficient
AI 理解论文
溯源树
样例
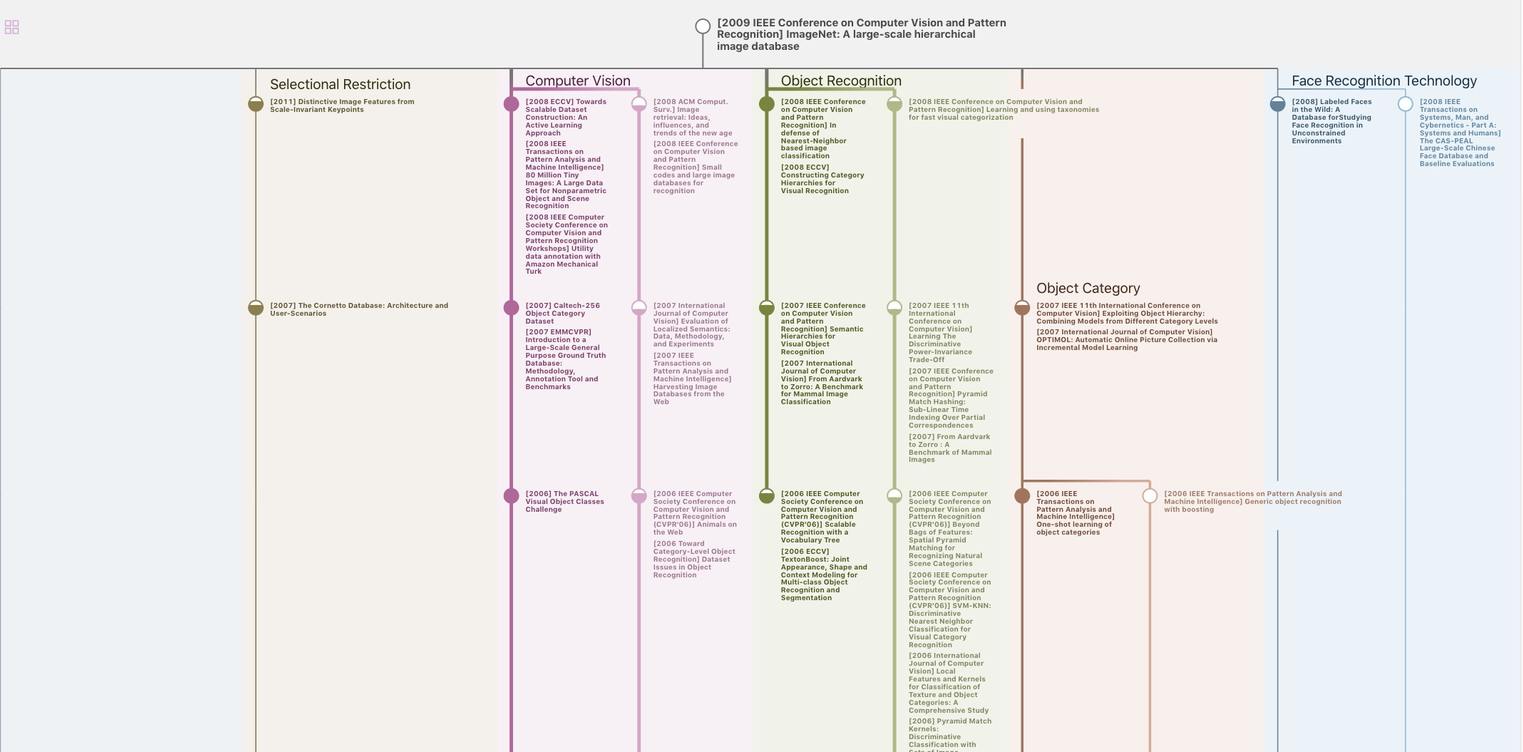
生成溯源树,研究论文发展脉络
Chat Paper
正在生成论文摘要