AdvDDoS: Zero-Query Adversarial Attacks Against Commercial Speech Recognition Systems.
IEEE Trans. Inf. Forensics Secur.(2023)
摘要
Automatic speech recognition (ASR) has been widely and commercially employed in health care, autonomous vehicles, and finance. Yet, recent studies have shown that universal adversarial perturbations (UAPs) pose a serious threat to white-box ASR systems when the adversary has access to the target model. Until now, the impacts of such a threat on commercial systems are still open since their models are not publicly available. To understand the security weakness in the practical black-box setting, this paper introduces the first zero-query UAP attacks, called AdvDDoS, with black-box access to ASR systems: we do not need to pay any query expense to estimate UAPs. Specifically, we craft targeted UAPs under a popular feature extractor and a local ASR model by reversing the robust target-category features, in which adversarial perturbations containing robust features are believed to have better transferability. Compared with vanilla UAPs, our UAPs incorporated with target-category features lead to better attacks against commercial ASR systems. We validate the efficacy of our AdvDDoS by launching attacks against a range of commercial ASR systems, i.e., three API services (Alibaba, Tencent, and Baidu), and three personal assistants (Apple Siri, iFlytek, and Google). Extensive experimental results demonstrate the superiority of AdvDDoS. For example, AdvDDoS achieves 83.26% word error rate (WER) and 53.25% success rates of attacks (SRoA) for the universal attack against Tencent ASR API, which outperforms the vanilla UAPs by up to 61.56% on WER and 11.6% on SRoA. The success of our attack sheds light on zero-query UAP attacks against commercial ASR systems.
更多查看译文
关键词
speech recognition,zero-query
AI 理解论文
溯源树
样例
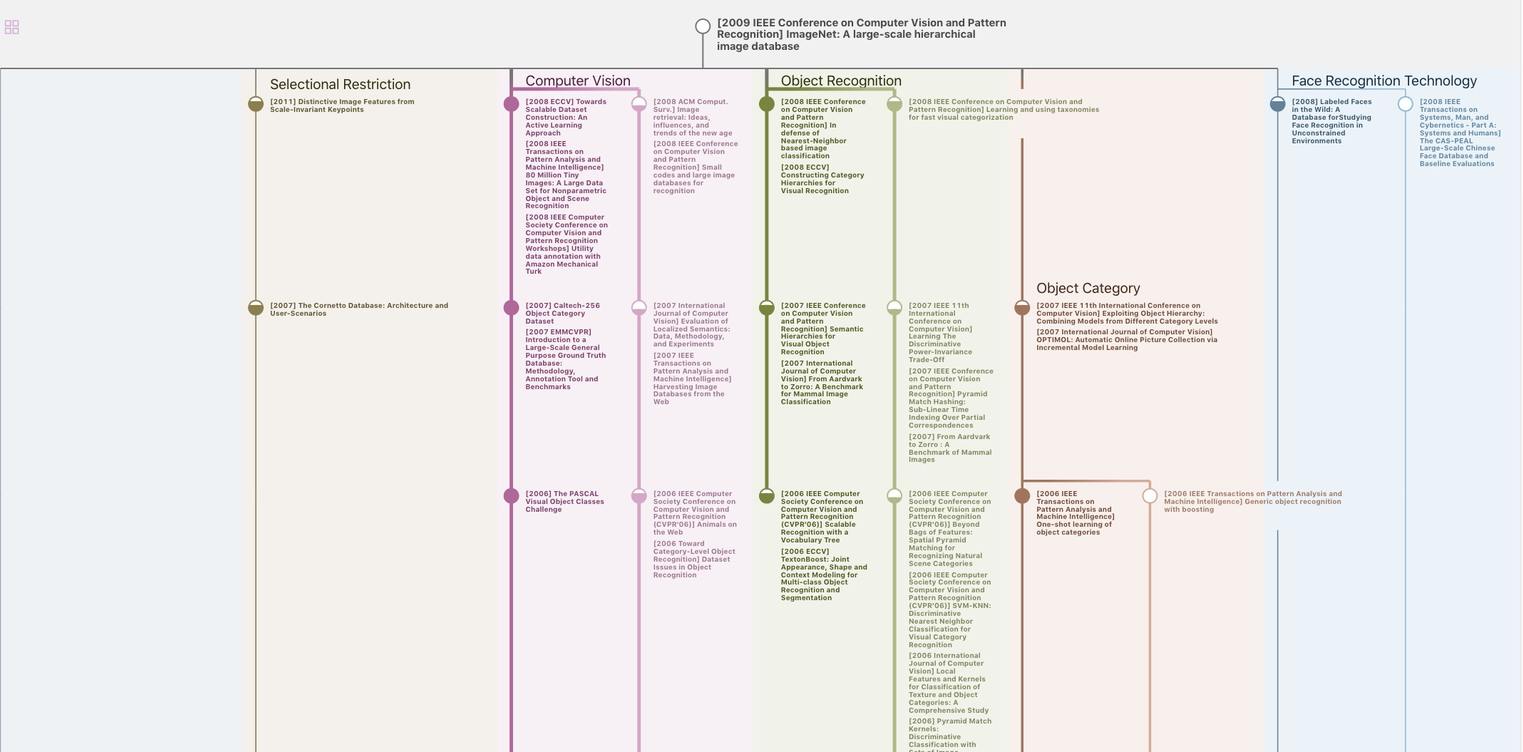
生成溯源树,研究论文发展脉络
Chat Paper
正在生成论文摘要