MVFSIGM: Multi-Variant Feature-Based Synthesis Image Generation Model for Improved Stability Using Generative Adversarial Network.
SOFT COMPUTING(2023)
摘要
Image synthesis has recently been identified as a sensitive issue due to the enormous volume of data required to function deep learning models. Deep learning models are used for various issues to support medical imaging, speech synthesis and text-to-speech and text-to-image conversion. Several machine-learning approaches exist but suffer to achieve higher accuracy and stability. To handle this issue, an efficient multi-variant feature synthesis image generation model is presented. The model works with the generative adversarial network (GAN) structure, which generates synthesis images according to different features like shape, color and object. The generator uses such features to generate multiple variations of images according to various compositions. The discriminator, in turn, classifies the image and returns values to the same, which is iterated a number of times till the negative classification becomes less. The generator is an inverse convolution neural network model, and the discriminator is a CNN classifier with probability. The method has been designed with the neurons to compute shape transition measure, color transition measure and object transition measure. Using each of these techniques, the method calculates the value of image synthesis support to produce multiple results from the generator. Similarly, the discriminator is designed to measure multi-variant feature synthesis support to identify the class of image. The classification accuracy of the model is 95% when compared to the classification accuracy of the KT-GAN, DR-GAN and BSD-GAN models. There are only 5% of instances that are incorrectly classified, according to the 5% false classification ratio. The model's stability is also 95%, indicating consistent and dependable performance across several runs or modifications. The method introduces higher performance in stability and presents higher classification performance.
更多查看译文
关键词
GAN,CNN,Image synthesis,Data augmentation,MVFSIGM,STM,CTM,OTM,ISS,MVFSS
AI 理解论文
溯源树
样例
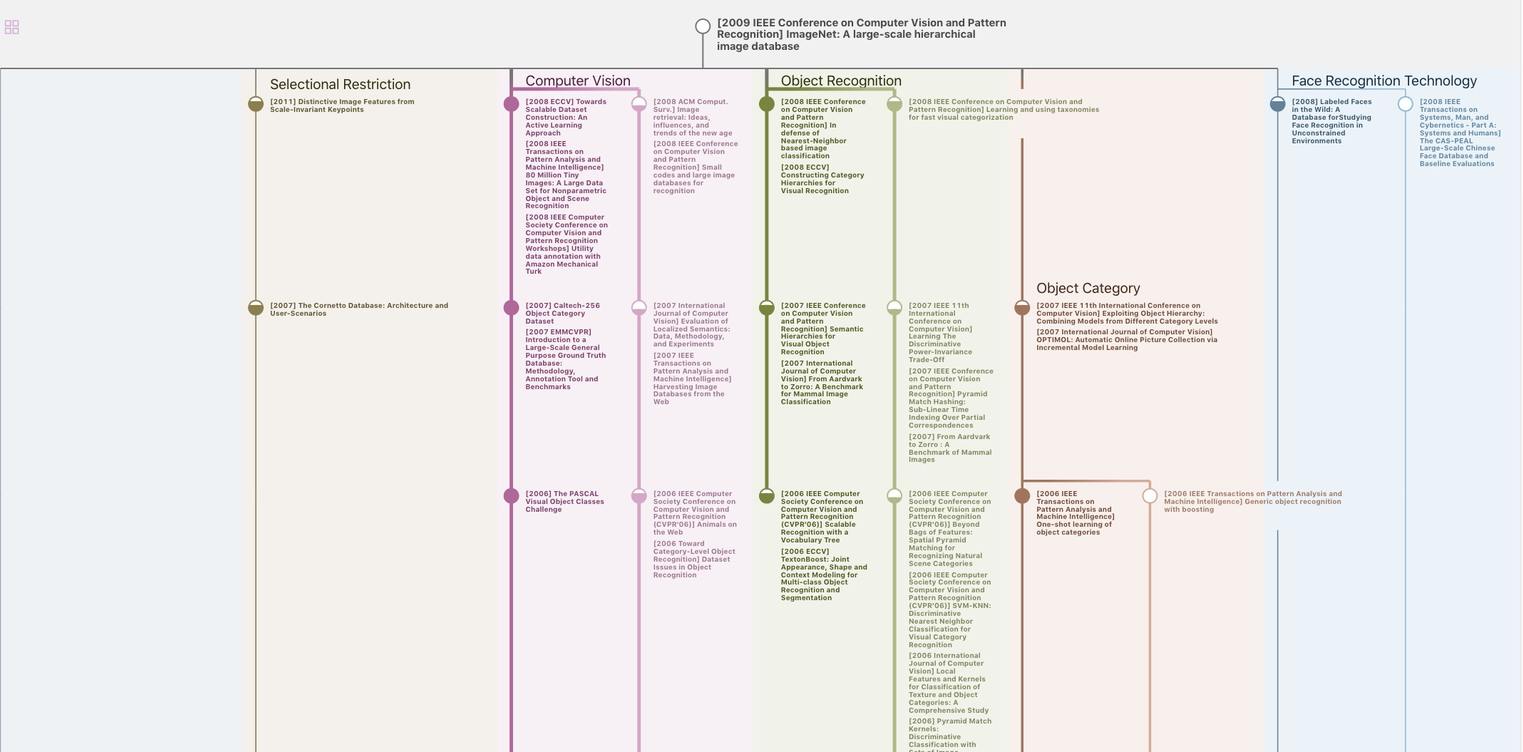
生成溯源树,研究论文发展脉络
Chat Paper
正在生成论文摘要