Machine Learning-Based Ensemble Band Selection for Early Water Stress Identification in Groundnut Canopy Using UAV-Based Hyperspectral Imaging.
IEEE Geosci. Remote. Sens. Lett.(2023)
摘要
This letter presents the early identification of water stress in groundnut (GN) canopy using unmanned aerial vehicle (UAV)-based hyperspectral imaging (HSI) (in 385-1020 nm) and machine learning (ML) techniques. An efficient HSI data analysis pipeline was presented which includes image quality assessment (IQA), denoising, band selection (BS), and classification. A novel ML-based ensemble feature selection (FS) algorithm has been proposed for optimal water stress sensitive waveband selection. The data analysis pipeline and the selected bands were validated on HSI data acquired at two different water stress levels. Wavelengths 515.05, 552.16, 711.92, 724.75, and 931.92 nm were identified as optimal water stress sensitive bands in the GN canopy, using which we could identify early stress with 96.46% accuracy. The proposed data analysis pipeline and ensemble FS algorithm will benefit crop phenotyping applications such as early abiotic stress detection.
更多查看译文
关键词
hyperspectral imaging,early water stress identification,groundnut canopy,learning-based,uav-based
AI 理解论文
溯源树
样例
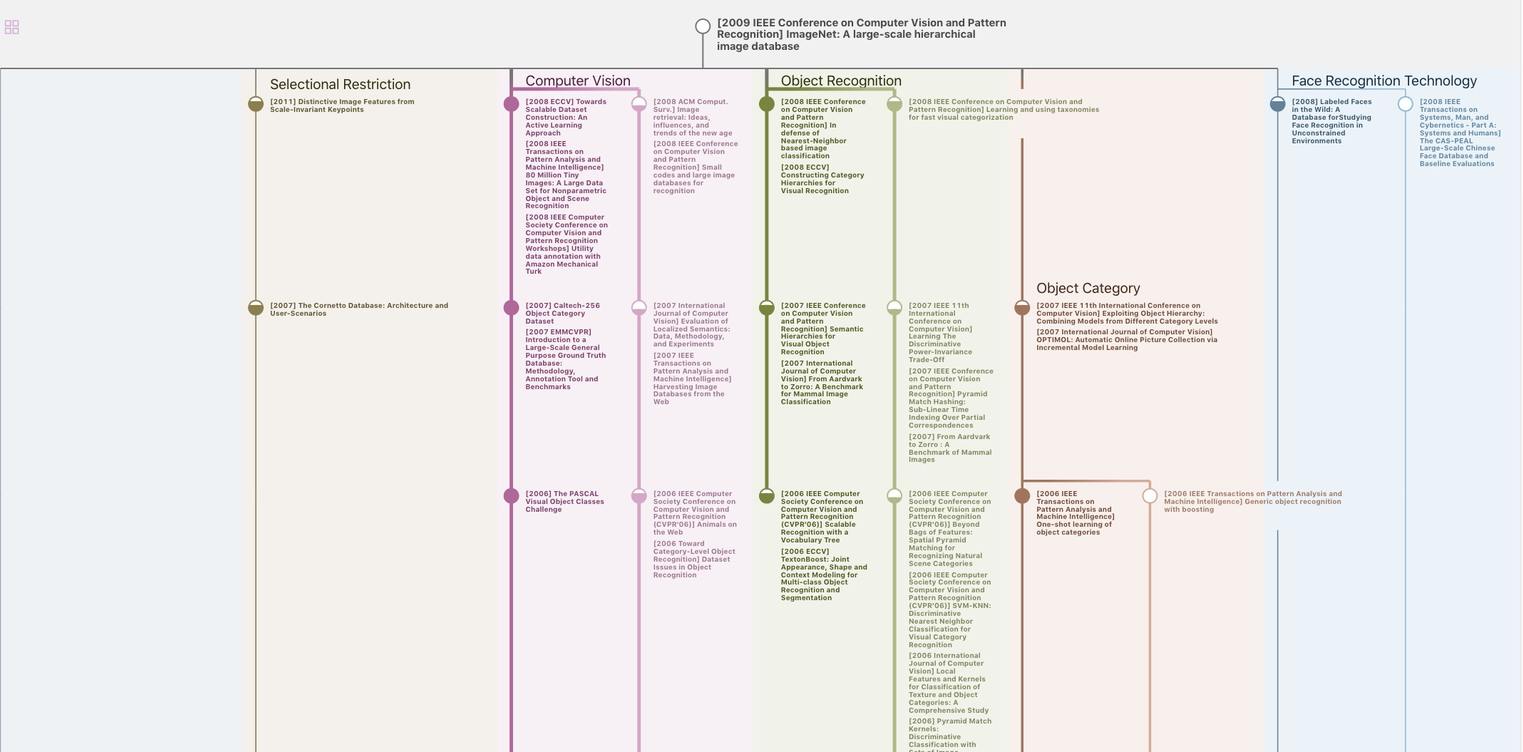
生成溯源树,研究论文发展脉络
Chat Paper
正在生成论文摘要