Multi-Grained Topological Pre-Training of Language Models in Sponsored Search
PROCEEDINGS OF THE 46TH INTERNATIONAL ACM SIGIR CONFERENCE ON RESEARCH AND DEVELOPMENT IN INFORMATION RETRIEVAL, SIGIR 2023(2023)
摘要
Relevance models measure the semantic closeness between queries and the candidate ads, widely recognized as the nucleus of sponsored search systems. Conventional relevance models solely rely on the textual data within the queries and ads, whose performance is hindered by the scarce semantic information in these short texts. Recently, user behavior graphs have been incorporated to provide complementary information beyond pure textual semantics. Despite the promising performance, behavior-enhanced models suffer from exhausting resource costs due to the extra computations introduced by explicit topological aggregations. In this paper, we propose a novel Multi-Grained Topological Pre-Training paradigm, MGTLM, to teach language models to understand multi-grained topological information in behavior graphs, which contributes to eliminating explicit graph aggregations and avoiding information loss. Extensive experimental results over online and offline settings demonstrate the superiority of our proposal.
更多查看译文
关键词
Sponsored Search,Relevance Modeling,Language Model
AI 理解论文
溯源树
样例
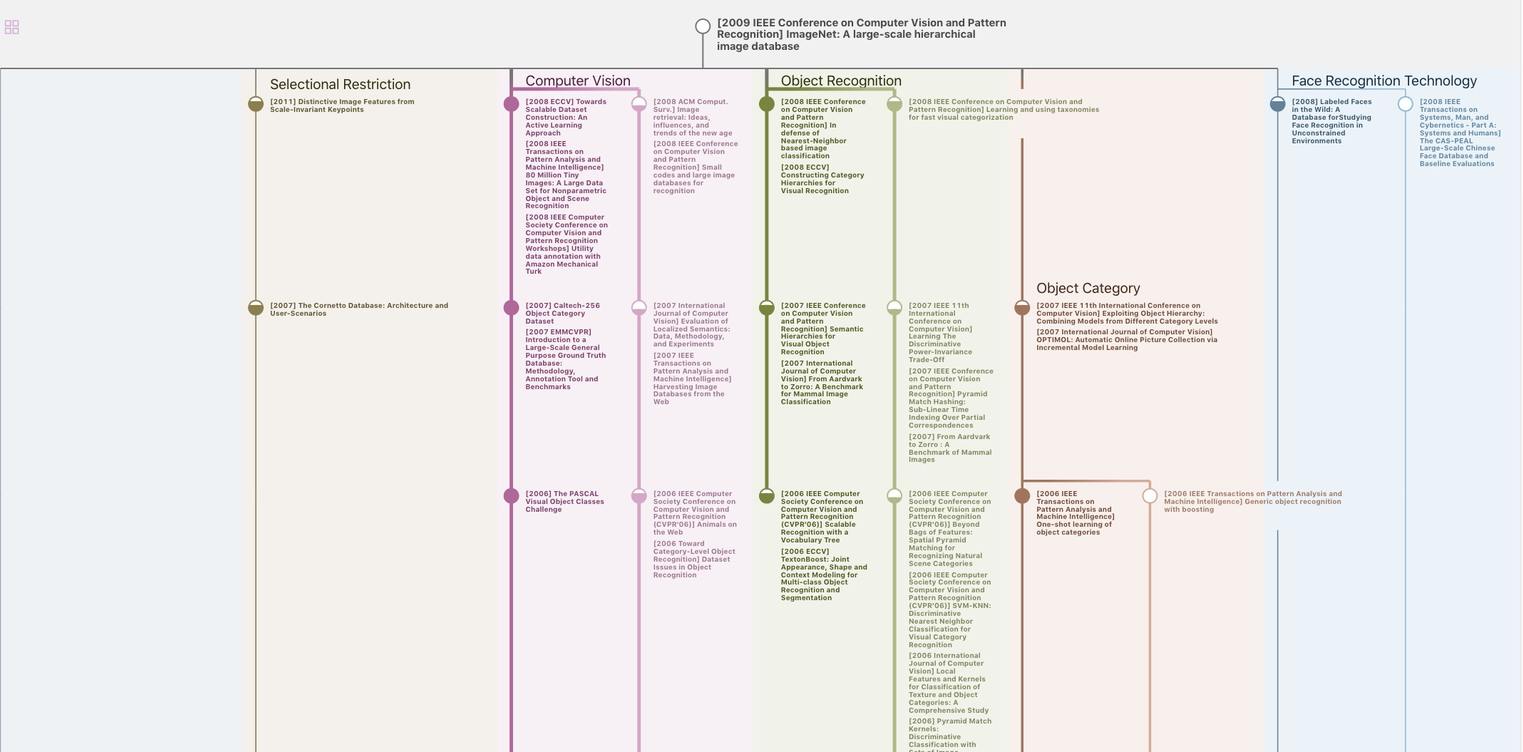
生成溯源树,研究论文发展脉络
Chat Paper
正在生成论文摘要