LinRec: Linear Attention Mechanism for Long-term Sequential Recommender Systems
PROCEEDINGS OF THE 46TH INTERNATIONAL ACM SIGIR CONFERENCE ON RESEARCH AND DEVELOPMENT IN INFORMATION RETRIEVAL, SIGIR 2023(2023)
摘要
Transformer models have achieved remarkable success in sequential recommender systems (SRSs). However, computing the attention matrix in traditional dot-product attention mechanisms results in a quadratic complexity with sequence lengths, leading to high computational costs for long-term sequential recommendation. Motivated by the above observation, we propose a novel L2-Normalized Linear Attention for the Transformer-based Sequential Recommender Systems (LinRec), which theoretically improves efficiency while preserving the learning capabilities of the traditional dot-product attention. Specifically, by thoroughly examining the equivalence conditions of efficient attention mechanisms, we show that LinRec possesses linear complexity while preserving the property of attention mechanisms. In addition, we reveal its latent efficiency properties by interpreting the proposed LinRec mechanism through a statistical lens. Extensive experiments are conducted based on two public benchmark datasets, demonstrating that the combination of LinRec and Transformer models achieves comparable or even superior performance than state-of-the-art Transformer-based SRS models while significantly improving time and memory efficiency. The implementation code is available online at https://github.com/Applied-Machine-Learning-Lab/LinRec.
更多查看译文
关键词
Efficient Transformer,Sequential Recommender Systems,Linear Complexity,L2 Normalization
AI 理解论文
溯源树
样例
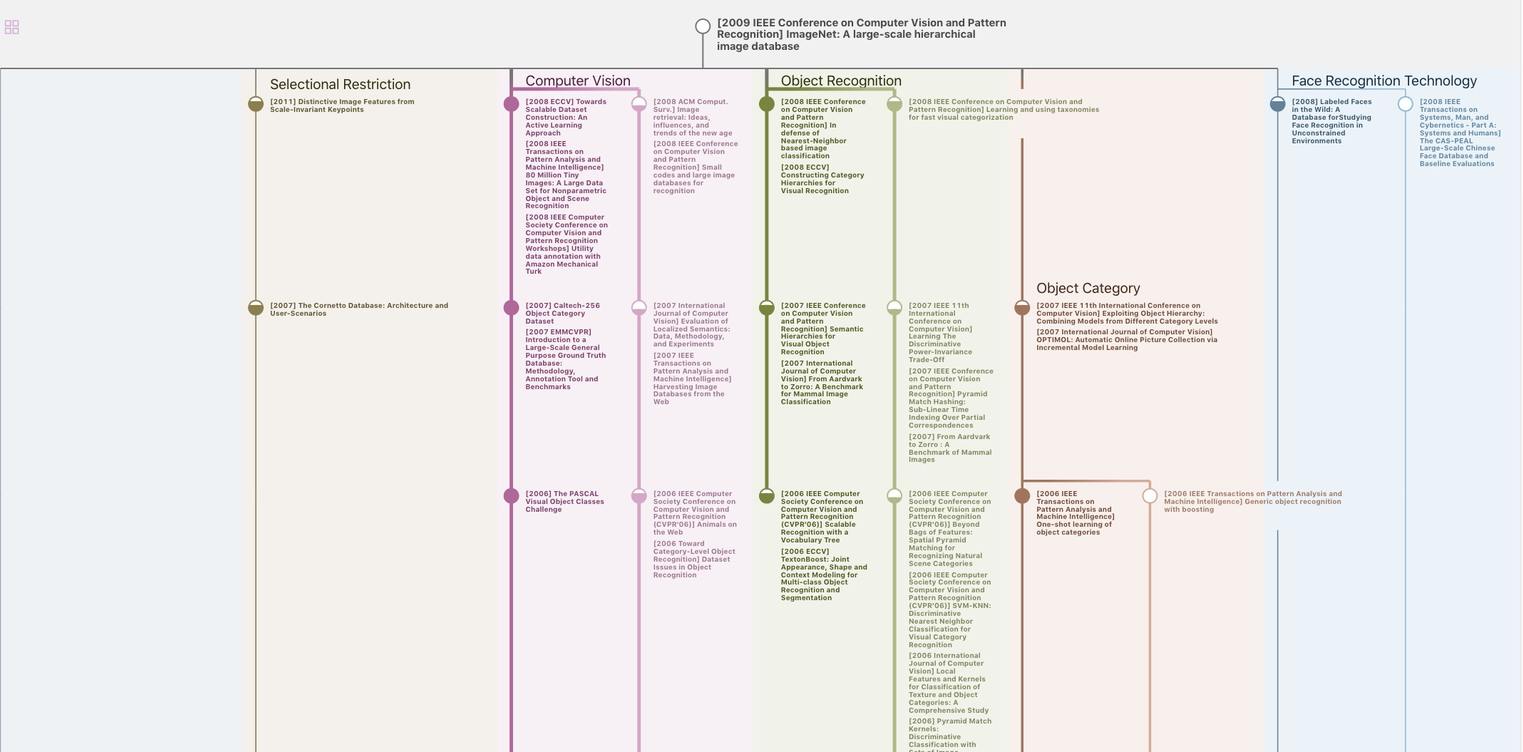
生成溯源树,研究论文发展脉络
Chat Paper
正在生成论文摘要