Aligning Distillation for Cold-start Item Recommendation.
SIGIR 2023(2023)
摘要
Recommending cold items in recommendation systems is a longstanding challenge due to the inherent differences between warm items, which are recommended based on user behavior, and cold items, which are recommended based on content features. To tackle this, generative models generate synthetic embeddings from content features, while dropout models enhance the robustness of the recommendation system by randomly dropping behavioral embeddings during training. However, these models primarily focus on handling the recommendation of cold items, but do not effectively address the differences between warm and cold recommendations. As a result, generative models may over-recommend either warm or cold items, neglecting the other type, and dropout models may negatively impact warm item recommendations. To address this, we propose the Aligning Distillation (ALDI) framework, which leverages warm items as "teachers" to transfer their behavioral information to cold items, referred to as "students". ALDI aligns the students with the teachers by comparing the differences in their recommendation characters, using tailored rating distribution aligning, ranking aligning, and identification aligning losses to narrow these differences. Furthermore, ALDI incorporates a teacher-qualifying weighting structure to prevent students from learning inaccurate information from unreliable teachers. Experiments on three datasets show that our approach outperforms state-of-the-art baselines in terms of overall, warm, and cold recommendation performance with three different recommendation backbones.
更多查看译文
关键词
cold-start recommendation,aligning distillation,content features
AI 理解论文
溯源树
样例
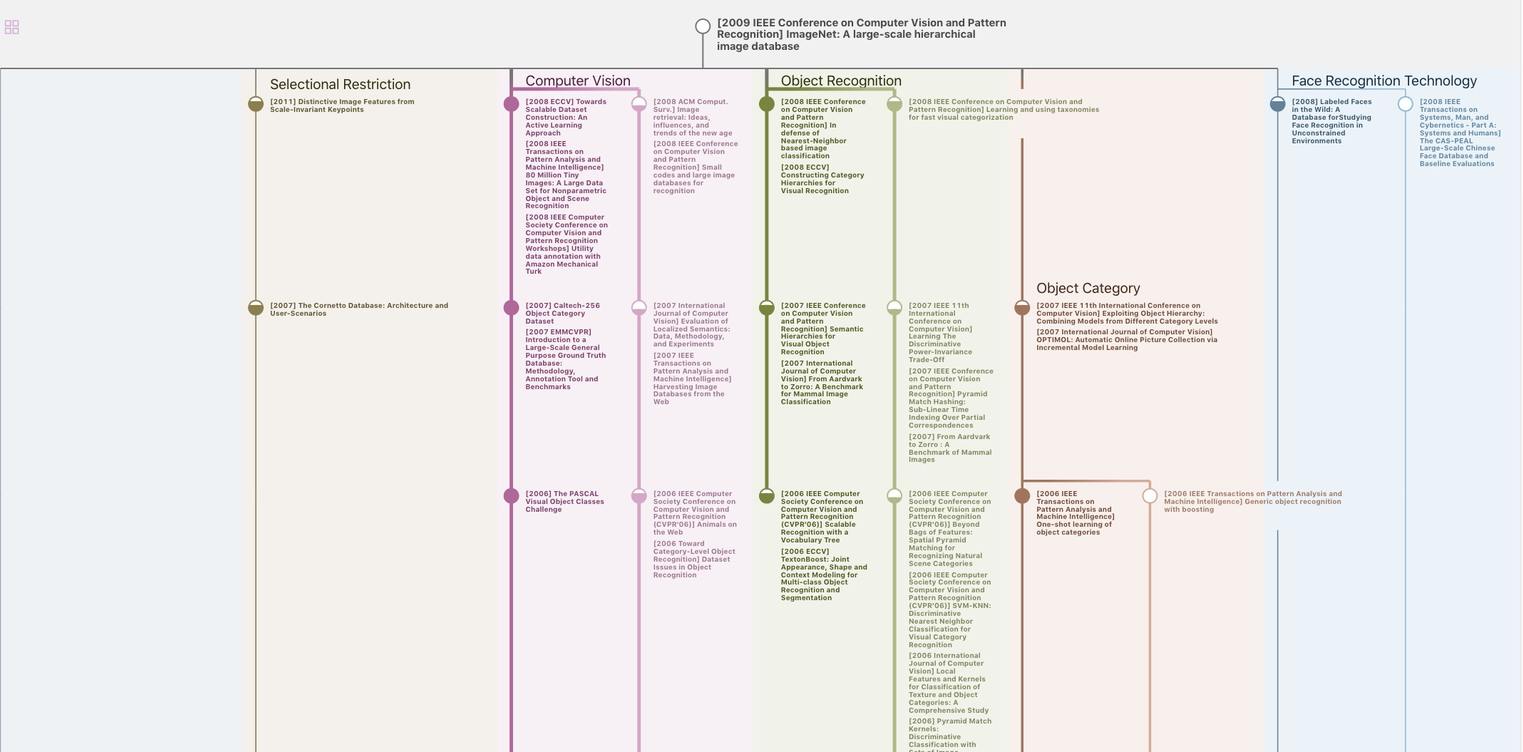
生成溯源树,研究论文发展脉络
Chat Paper
正在生成论文摘要