Distributionally Robust Sequential Recommendation
SIGIR(2023)
摘要
Modeling user sequential behaviors have been demonstrated to be effective in promoting the recommendation performance. While previous work has achieved remarkable successes, they mostly assume that the training and testing distributions are consistent, which may contradict with the diverse and complex user preferences, and limit the recommendation performance in real-world scenarios. To alleviate this problem, in this paper, we propose a robust sequential recommender framework to overcome the potential distribution shift between the training and testing sets. In specific, we firstly simulate different training distributions via sample reweighting. Then, we minimize the largest loss induced by these distributions to optimize the "worst-case" loss for improving the model robustness. Considering that there can be too many sample weights, which may introduce too much flexibility and be hard to optimize, we cluster the training samples based on both hard and soft strategies, and assign each cluster with a unified weight. At last, we analyze our framework by presenting the generalization error bound of the above minimax objective, which help us to better understand the proposed framework from the theoretical perspective. We conduct extensive experiments based on three real-world datasets to demonstrate the effectiveness of our proposed framework. To reproduce our experiments and promote this research direction, we have released our project at https://anonymousrsr.github.io/RSR/.
更多查看译文
关键词
Sequential Recommendation,Robust Learning,Distribution Shifts
AI 理解论文
溯源树
样例
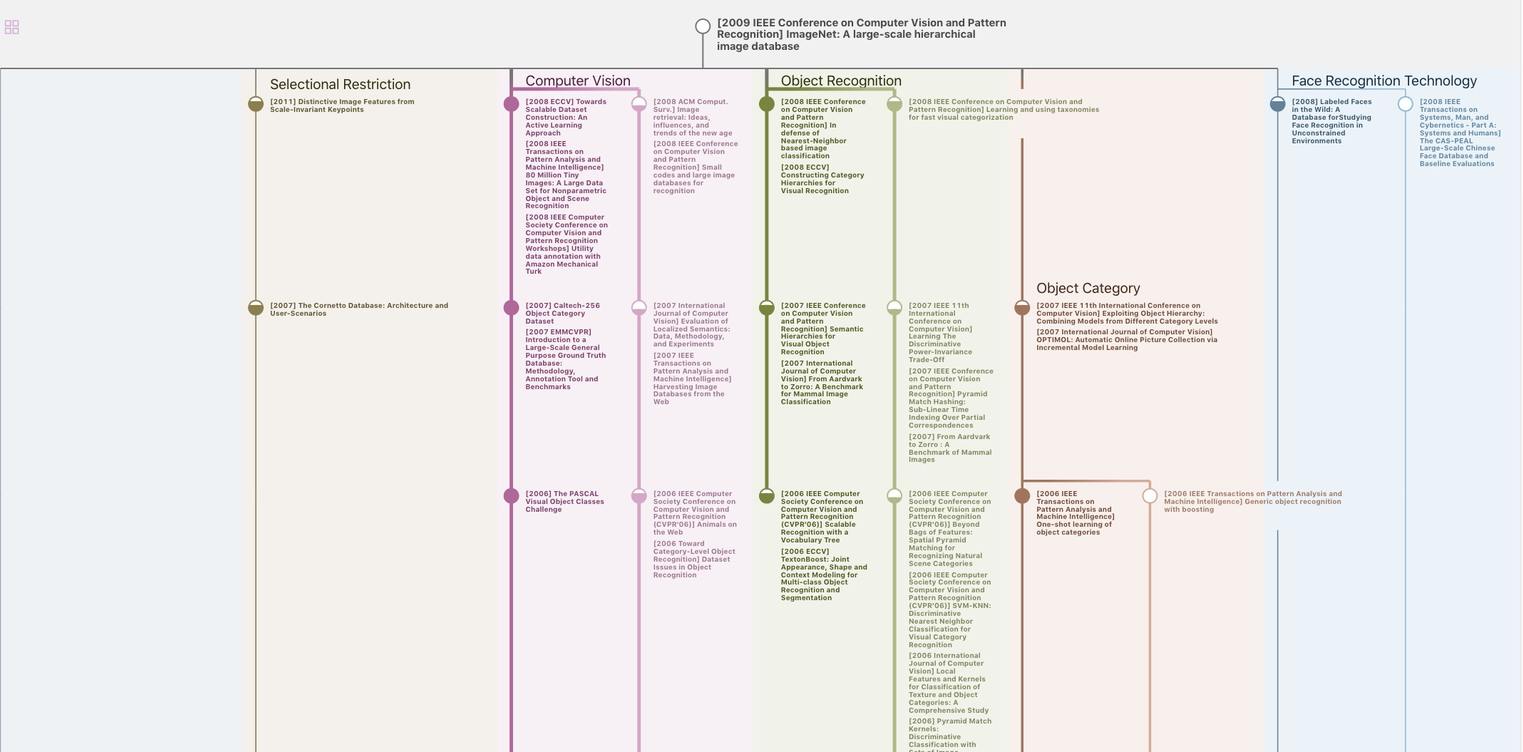
生成溯源树,研究论文发展脉络
Chat Paper
正在生成论文摘要