CEC: Towards Learning Global Optimized Recommendation through Causality Enhanced Conversion Model
PROCEEDINGS OF THE 46TH INTERNATIONAL ACM SIGIR CONFERENCE ON RESEARCH AND DEVELOPMENT IN INFORMATION RETRIEVAL, SIGIR 2023(2023)
摘要
Most e-commerce platforms consist of multiple entries (e.g., recommendation, search, shopping cart and etc.) for users to purchase their liked items. Among the research on the recommendation entry, most of them focus on improving the conversion volumes merely in the recommendation entry. However, such way could not ensure an increase in the global conversion volumes of the e-commerce platform. To achieve this goal by optimizing the recommendation entry only, in this paper, we focus on modeling the causality between the recommendation-entry-impression and the conversion by proposing the two-stage Causality Enhanced Conversion (CEC) model. In the first stage, we define the recommendation-entry-impression as treatment, then we estimate the conversion rate conditioned on the inclusion or exclusion of treatment respectively and calculate the corresponding individual treatment effect (ITE). In the second stage, we propose a propensity-normalization (PN) based method to transform the learned ITE to a weight term for instance weighting in the conversion loss. Extensive offline and online experiments on a large-scale food e-commerce scenario demonstrate that the CEC model could focus more on those conversed instances that can improve the global conversion volumes of the platform.
更多查看译文
关键词
Individual Treatment Effect Estimation,Conversion Rate Prediction
AI 理解论文
溯源树
样例
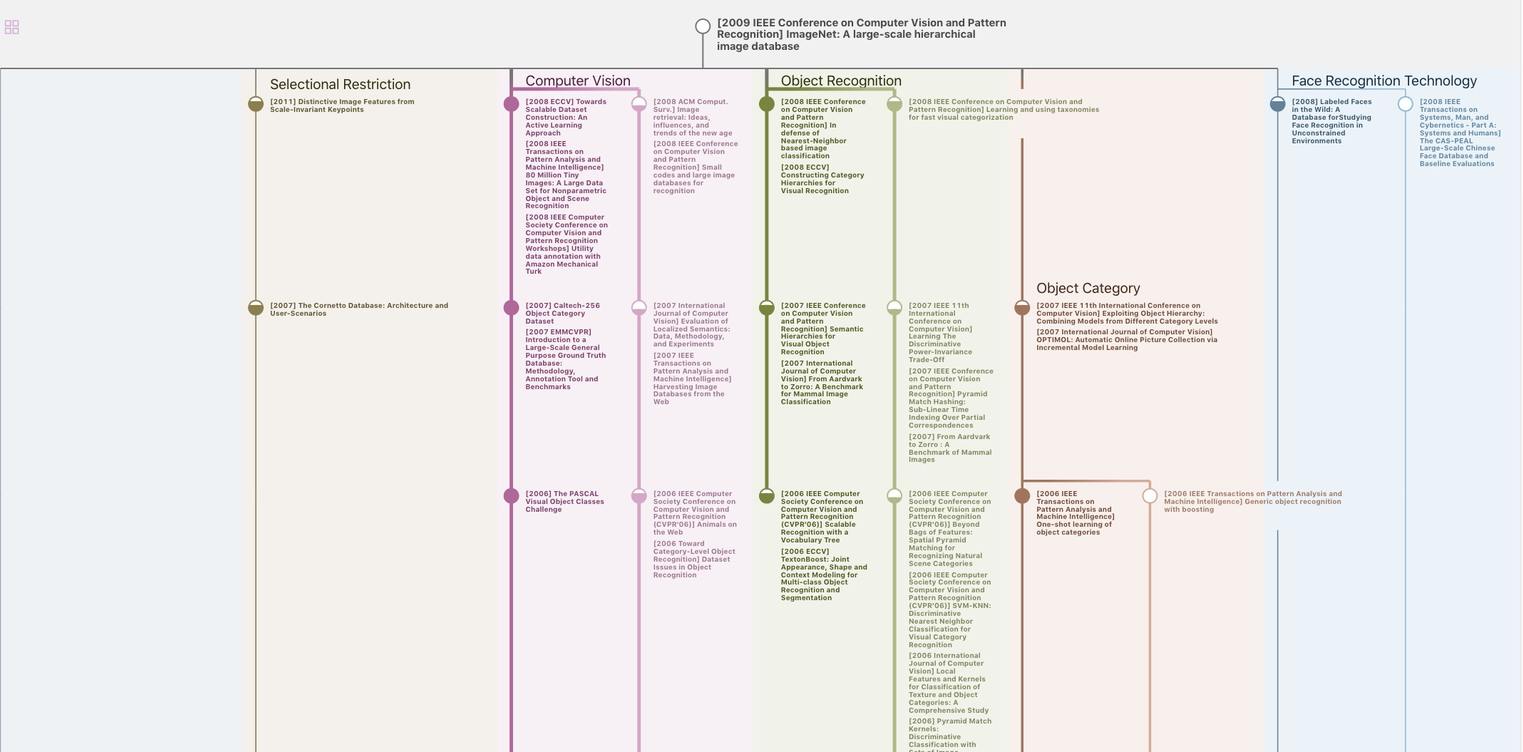
生成溯源树,研究论文发展脉络
Chat Paper
正在生成论文摘要