IRL-PM: An Inverse Reinforcement Learning-based Power Minimization in Multi-User MISO Networks.
ICCCS(2023)
摘要
This paper aims to efficiently save energy consumption of multiple-input-single-output (MISO) networks. To this end, an optimization problem is formulated to minimize the transmit power of the base station (BS) while ensuring the minimal required information rate of each user. Reinforcement learning (RL) is a significant solution to tackle this non-convex problems. Nevertheless, designing efficient reward functions that accurately describe the involved optimization problems for RL is a challenging problem, which may result in low learning efficiency and even inaccurate learning. To cope with such an issue, this paper proposes an inverse reinforcement learning (IRL)-based approach to minimize the transmit power of MISO networks, in which the generative adversarial imitation learning (GAIL) is employed as the IRL framework and the reward function is represented by an artificial neural network (ANN) model, whose parameters are learned automatically in terms of an expert policy based on the semidefinite relaxation (SDR)-based approach. Since SDR is able to achieve the near optimal solution, the learned reward function is more accurate than traditional artificially designed one. Simulations show that, the proposed IRL-based approach reaches similar energy consumption to the SDR-based approach, and reduces about 50% transmit power compared to traditional RL-based one.
更多查看译文
关键词
Inverse reinforcement learning,MISO networks,power minimization,QoS constraints
AI 理解论文
溯源树
样例
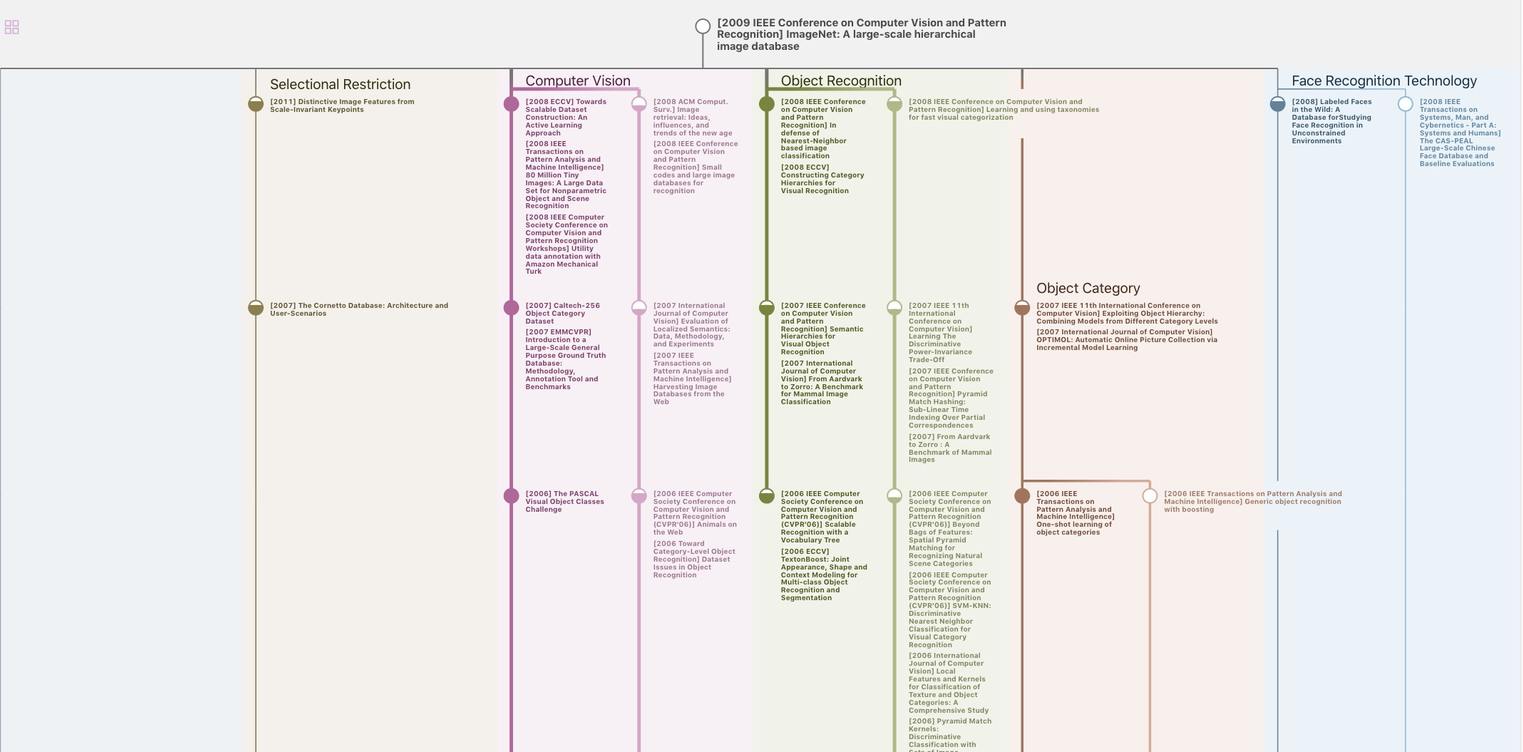
生成溯源树,研究论文发展脉络
Chat Paper
正在生成论文摘要