Research on Satellite Traffic Classification Based on Deep Packet Recognition and Convolution Neural Network.
ICCCS(2023)
National Mobile Communications Research Laboratory
Abstract
Over the past decade, Satellite communication has been a crucial topic. In particular, as we move from the 5G era to the 6G era, satellites will play an increasingly critical role in providing coverage and flexibility. With the increasing complexity of satellite network environment, the resource allocation of satellite network is becoming more and more important. Accurate identification of traffic types for classification can allocate network resources more effectively. Each method has its own advantages and disadvantages. In order to minimize the impact of the disadvantages, a reasonable combination of them is a new way to accomplish this task. In this paper, we propose a traffic classification method based on deep packet inspection (DPI) and convolution neural network (CNN), and verify it with open data sets. Experimental results show the effectiveness of our proposed method.
MoreTranslated text
Key words
satellite communication,traffic classification,deep packet inspection(DPI),convolution neural network(CNN)
求助PDF
上传PDF
View via Publisher
AI Read Science
AI Summary
AI Summary is the key point extracted automatically understanding the full text of the paper, including the background, methods, results, conclusions, icons and other key content, so that you can get the outline of the paper at a glance.
Example
Background
Key content
Introduction
Methods
Results
Related work
Fund
Key content
- Pretraining has recently greatly promoted the development of natural language processing (NLP)
- We show that M6 outperforms the baselines in multimodal downstream tasks, and the large M6 with 10 parameters can reach a better performance
- We propose a method called M6 that is able to process information of multiple modalities and perform both single-modal and cross-modal understanding and generation
- The model is scaled to large model with 10 billion parameters with sophisticated deployment, and the 10 -parameter M6-large is the largest pretrained model in Chinese
- Experimental results show that our proposed M6 outperforms the baseline in a number of downstream tasks concerning both single modality and multiple modalities We will continue the pretraining of extremely large models by increasing data to explore the limit of its performance
Upload PDF to Generate Summary
Must-Reading Tree
Example
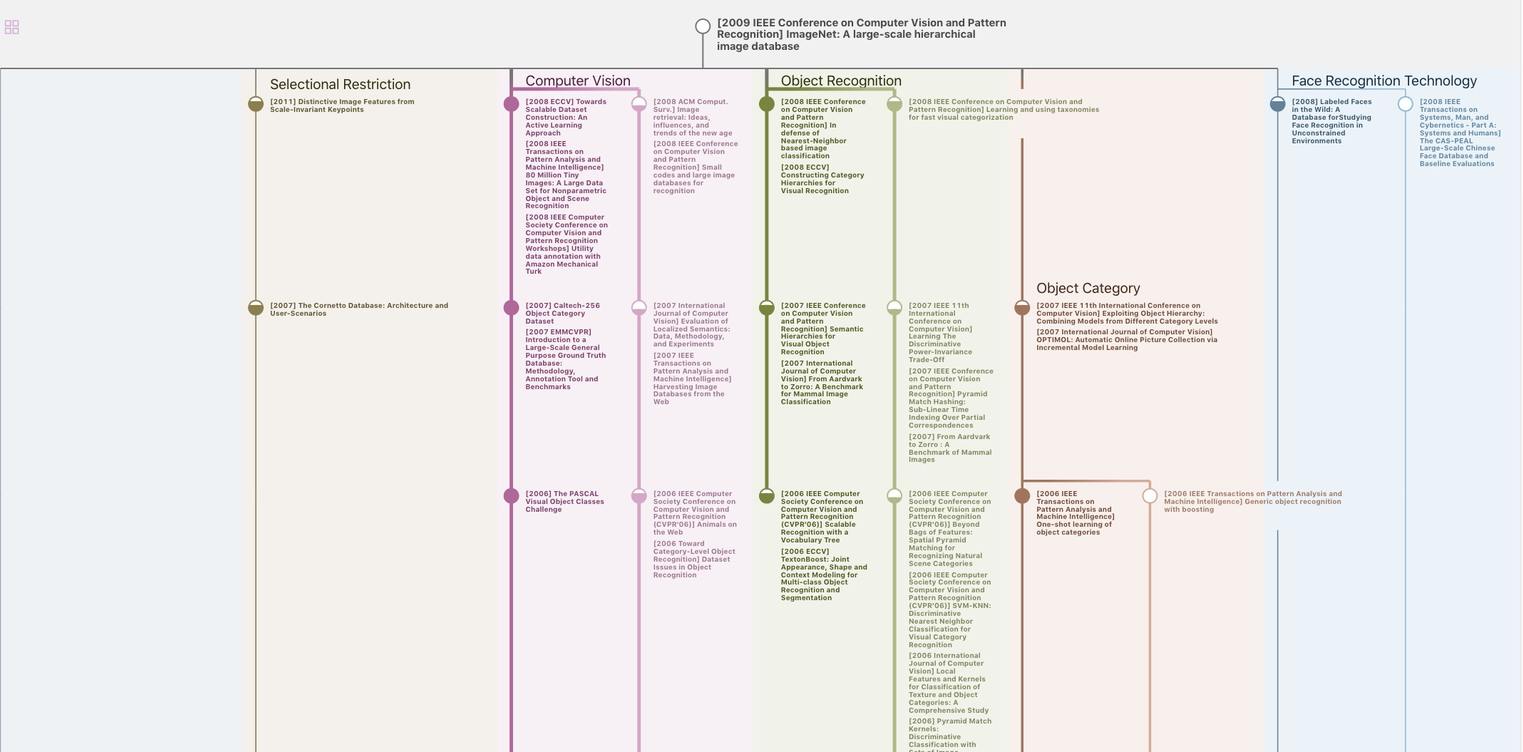
Generate MRT to find the research sequence of this paper
Related Papers
Applications of Deep Learning in Satellite Communication: A Survey
SPACE INFORMATION NETWORKS, SINC 2023 2024
被引用0
One-dimensional CNN Model of Network Traffic Classification Based on Transfer Learning
KSII TRANSACTIONS ON INTERNET AND INFORMATION SYSTEMS 2024
被引用0
Data Disclaimer
The page data are from open Internet sources, cooperative publishers and automatic analysis results through AI technology. We do not make any commitments and guarantees for the validity, accuracy, correctness, reliability, completeness and timeliness of the page data. If you have any questions, please contact us by email: report@aminer.cn
Chat Paper