LagNet: Deep Lagrangian Mechanics for Plug-and-Play Molecular Representation Learning.
AAAI(2023)
摘要
Molecular representation learning is a fundamental problem in the field of drug discovery and molecular science. Whereas incorporating molecular 3D information in the representations of molecule seems beneficial, which is related to computational chemistry with the basic task of predicting stable 3D structures (conformations) of molecules. Existing machine learning methods either rely on 1D and 2D molecular properties or simulate molecular force field to use additional 3D structure information via Hamiltonian network. The former has the disadvantage of ignoring important 3D structure features, while the latter has the disadvantage that existing Hamiltonian neural network must satisfy the "canonial" constraint, which is difficult to be obeyed in many cases. In this paper, we propose a novel plug-and-play architecture LagNet by simulating molecular force field only with parameterized position coordinates, which implements Lagrangian mechanics to learn molecular representation by preserving 3D conformation without obeying any additional restrictions. LagNet is designed to generate known conformations and generalize for unknown ones from molecular SMILES. Implicit positions in LagNet are learned iteratively using discrete-time Lagrangian equations. Experimental results show that LagNet can well learn 3D molecular structure features, and outperforms previous state-of-the-art baselines related molecular representation by a significant margin.
更多查看译文
关键词
lagrangian mechanics,learning,representation,plug-and-play
AI 理解论文
溯源树
样例
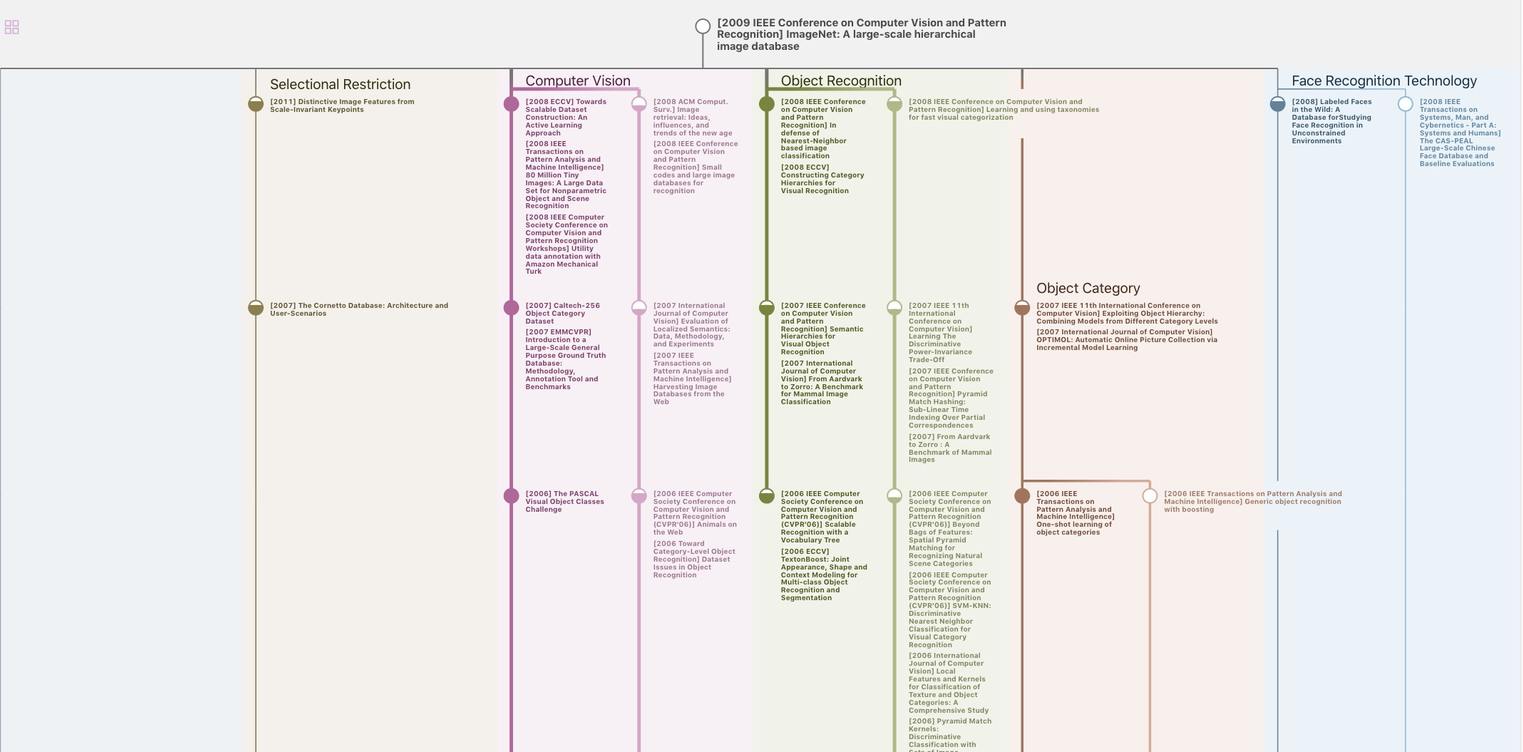
生成溯源树,研究论文发展脉络
Chat Paper
正在生成论文摘要