GMDNet: A Graph-Based Mixture Density Network for Estimating Packages' Multimodal Travel Time Distribution.
AAAI(2023)
摘要
In the logistics network, accurately estimating packages' Travel Time Distribution (TTD) given the routes greatly benefits both consumers and platforms. Although recent works perform well in predicting an expected time or a time distribution in a road network, they could not be well applied to estimate TTD in logistics networks. Because TTD prediction in the logistics network requires modeling packages' multimodal TTD (MTTD, i.e., there can be more than one likely output with a given input) while leveraging the complex correlations in the logistics network. To this end, this work opens appealing research opportunities in studying MTTD learning conditioned on graph-structure data by investigating packages' travel time distribution in the logistics network. We propose a Graph-based Mixture Density Network, named GMD-Net, which takes the benefits of both graph neural network and mixture density network for estimating MTTD conditioned on graph-structure data (i.e., the logistics network). Furthermore, we adopt the Expectation-Maximization (EM) framework in the training process to guarantee local convergence and thus obtain more stable results than gradient descent. Extensive experiments on two real-world datasets demonstrate the superiority of our proposed model.
更多查看译文
关键词
mixture density network,estimating packages,distribution,graph-based
AI 理解论文
溯源树
样例
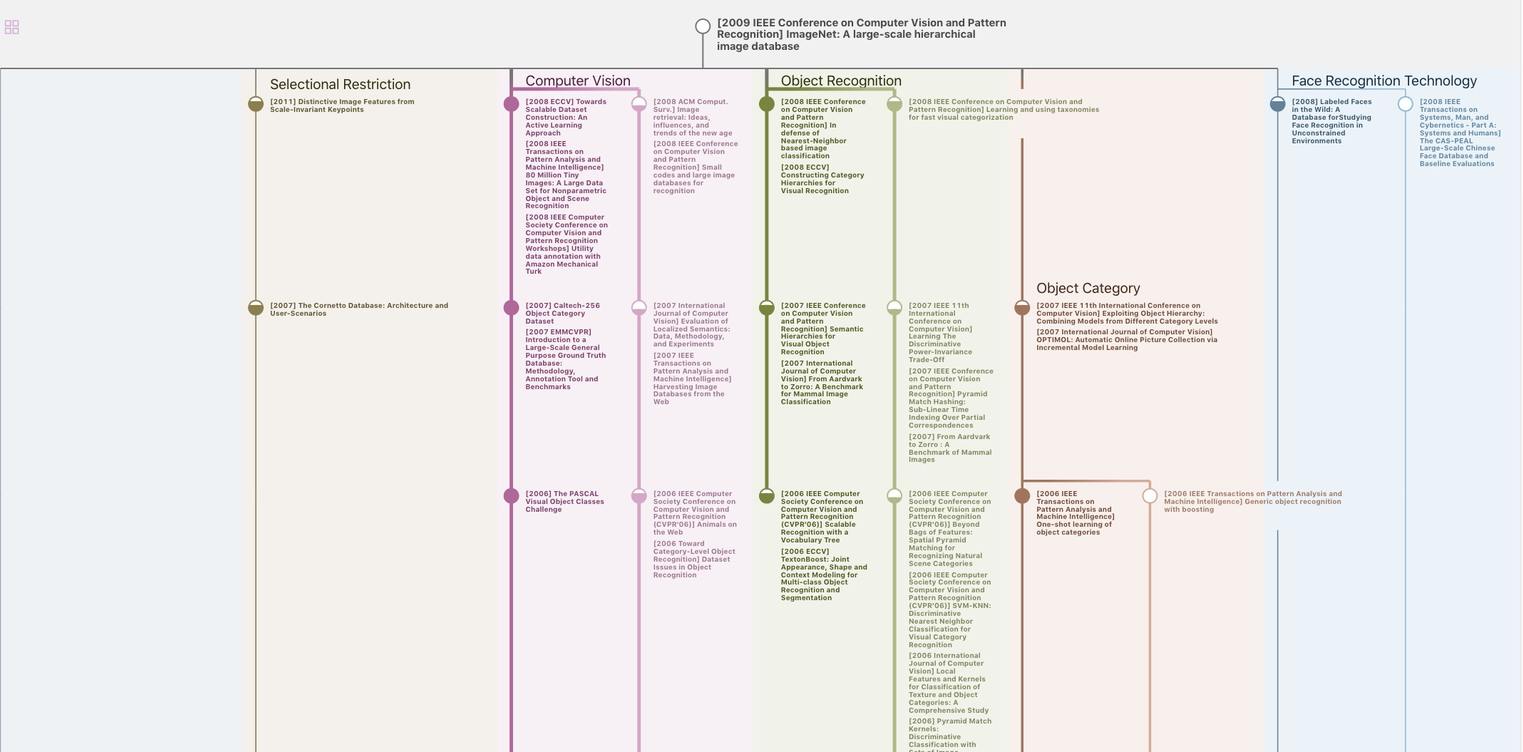
生成溯源树,研究论文发展脉络
Chat Paper
正在生成论文摘要