An Equivalence Analysis of Binary Quantification Methods.
AAAI(2023)
摘要
Quantification (or prevalence estimation) algorithms aim at predicting the class distribution of unseen sets (or bags) of examples. These methods are useful for two main tasks: 1) quantification applications, for instance when we need to track the proportions of several groups of interest over time, and 2) domain adaptation problems, in which we usually need to adapt a previously trained classifier to a different -albeit related-target distribution according to the estimated prevalences. This paper analyzes several binary quantification algorithms showing that not only do they share a common framework but are, in fact, equivalent. Inspired by this study, we propose a new method that extends one of the approaches analyzed. After an empirical evaluation of all these methods using synthetic and benchmark datasets, the paper concludes recommending three of them due to their precision, efficiency, and diversity.
更多查看译文
关键词
equivalence analysis,quantification,methods
AI 理解论文
溯源树
样例
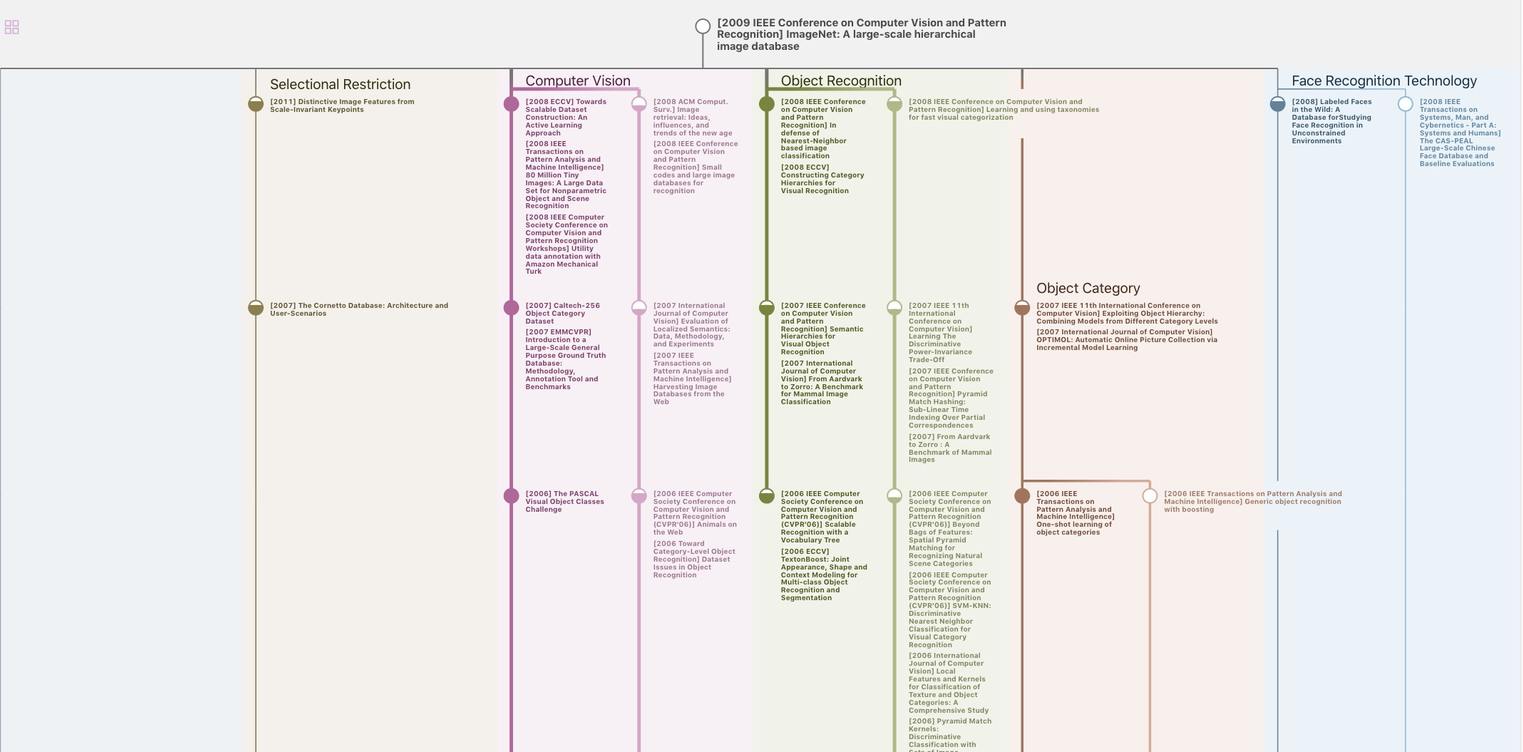
生成溯源树,研究论文发展脉络
Chat Paper
正在生成论文摘要