Soft Target-Enhanced Matching Framework for Deep Entity Matching
THIRTY-SEVENTH AAAI CONFERENCE ON ARTIFICIAL INTELLIGENCE, VOL 37 NO 4(2023)
摘要
Deep Entity Matching (EM) is one of the core research topics in data integration. Typical existing works construct EM models by training deep neural networks (DNNs) based on the training samples with onehot labels. However, these sharp supervision signals of onehot labels harm the generalization of EM models, causing them to overfit the training samples and perform badly in unseen datasets. To solve this problem, we first propose that the challenge of training a well-generalized EM model lies in achieving the compromise between fitting the training samples and imposing regularization, i.e., the bias-variance tradeoff . Then, we propose a novel S oft T arget- E nh A nced M atching ( STEAM ) framework, which exploits the automatically generated soft targets as label-wise regularizers to constrain the model training. Specifically, STEAM regards the EM model trained in previous iteration as a virtual teacher and takes its softened output as the extra regularizer to train the EM model in the current iteration. As such, STEAM effectively calibrates the obtained EM model, achieving the bias-variance tradeoff without any additional computational cost. We conduct extensive experiments over open datasets and the results show that our proposed STEAM outperforms the state-of-the-art EM approaches in terms of effectiveness and label efficiency.
更多查看译文
关键词
Named Entity Recognition,Ensemble Learning,Topic Modeling,Data Integration,Entity Resolution
AI 理解论文
溯源树
样例
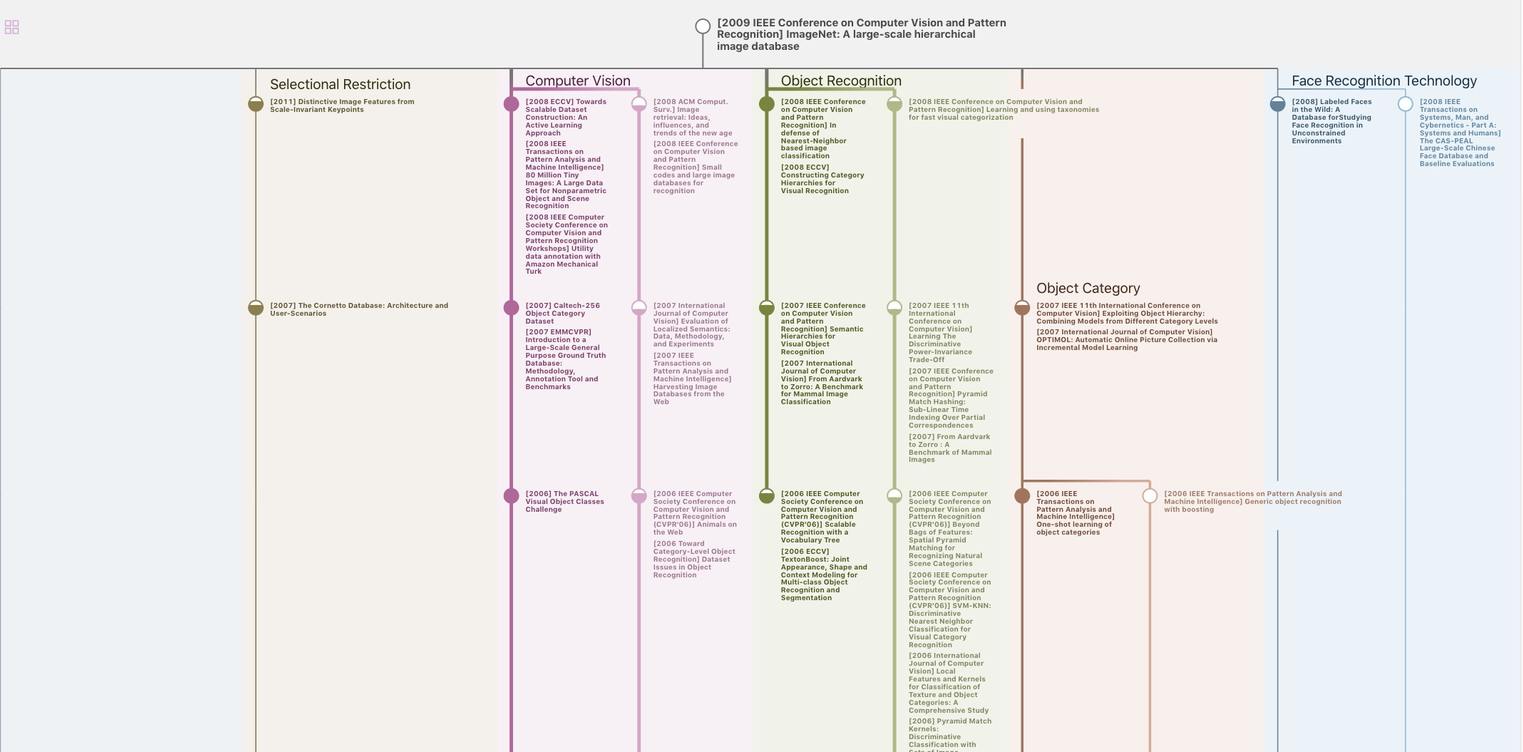
生成溯源树,研究论文发展脉络
Chat Paper
正在生成论文摘要