Incremental Recursive Ranking Grouping - A Decomposition Strategy for Additively and Nonadditively Separable Problems
PROCEEDINGS OF THE 2023 GENETIC AND EVOLUTIONARY COMPUTATION CONFERENCE COMPANION, GECCO 2023 COMPANION(2023)
摘要
Many real-world optimization problems may be classified as Large-Scale Global Optimization (LSGO) problems. When these high-dimensional problems are continuous, it was shown effective to embed a decomposition strategy into a Cooperative Co-Evolution (CC) framework. The effectiveness of the method that decomposes a problem into subproblems and optimizes them separately may depend on the decomposition accuracy and cost. Recent decomposition strategy advances focus mainly on Differential Grouping (DG). However, when a considered problem is nonadditively separable, DG-based strategies may report some variables as interacting, although the interaction between them does not exist. Monotonicity checking strategies do not suffer from this disadvantage. However, they suffer from another decomposition inaccuracy - monotonicity checking strategies may miss discovering many existing interactions. Therefore, Incremental Recursive Ranking Grouping (IRRG) is a new proposition that accurately decomposes both additively and nonadditively separable problems. The decomposition cost of IRRG is higher when compared with Recursive DG 3 (RDG3). Since the higher cost was a negligible part of the overall computational budget, optimization results of the considered CC frameworks were affected mainly by the decomposition accuracy.
更多查看译文
关键词
Large-Scale Global Optimization,Problem Decomposition,Monotonicity Checking,Nonadditive Separability
AI 理解论文
溯源树
样例
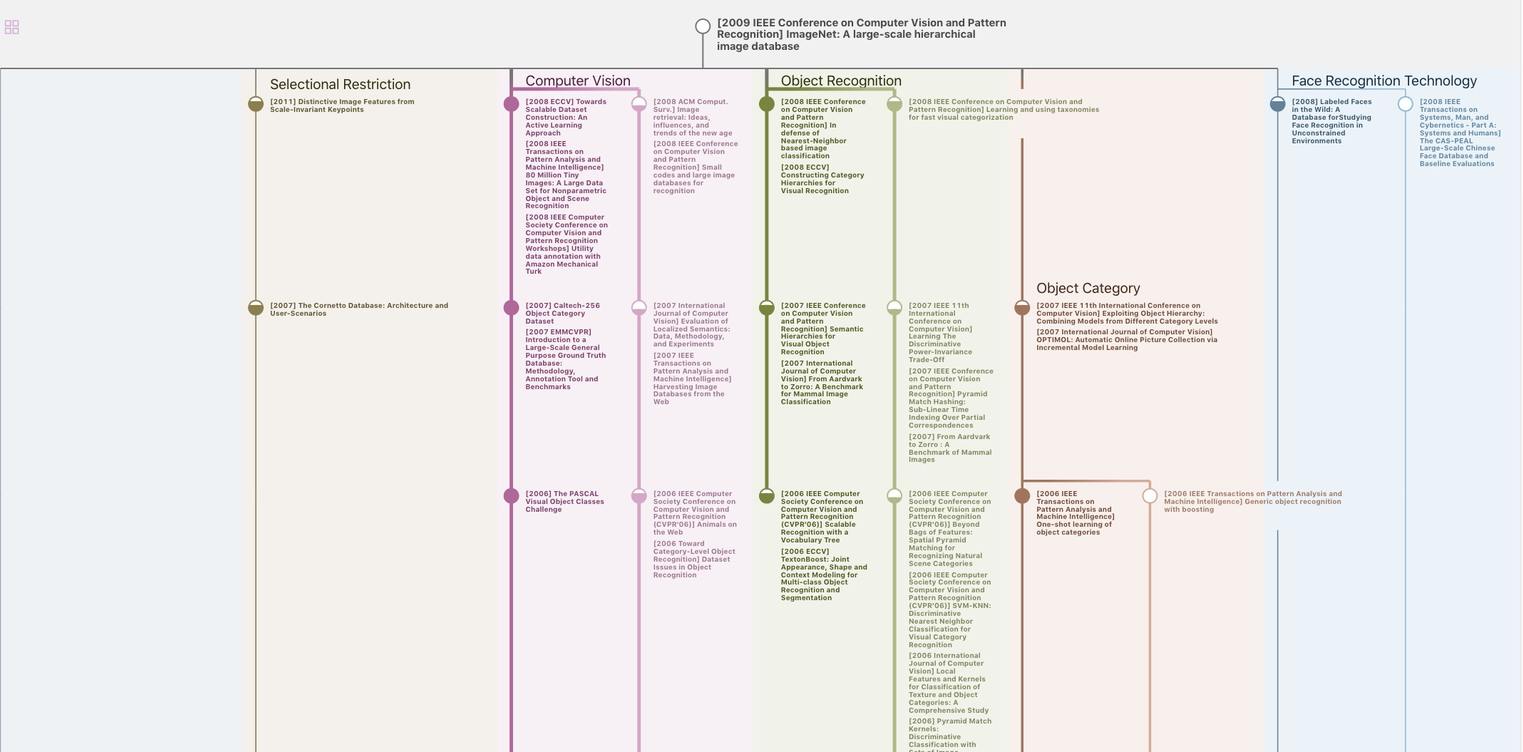
生成溯源树,研究论文发展脉络
Chat Paper
正在生成论文摘要