LSevoBN: a structure learning algorithm for large Bayesian networks
PROCEEDINGS OF THE 2023 GENETIC AND EVOLUTIONARY COMPUTATION CONFERENCE COMPANION, GECCO 2023 COMPANION(2023)
摘要
The issue of structure learning in large Bayesian networks has gained significant attention from the academic community in recent years due to its wide-ranging applications in various fields. Efficient algorithms for structure learning are crucial for enabling the practical use of Bayesian networks in real-world scenarios where the number of variables can be substantial. In previous approaches, addressing this problem has resulted in either prolonged convergence times or significant degradation of the problem. Although evolutionary algorithms have been effective in training Bayesian Networks (BNs), their application to large BNs has been impractical due to lengthy convergence times. In this paper, we introduce a modified evolutionary algorithm for training large BNs by dividing the network into smaller ones, coding and connecting them through evolutionary optimization. Our proposed approach significantly accelerates the training process, achieving a time acceleration of more than twenty times compared to existing solutions, while maintaining comparable or slightly degraded quality.
更多查看译文
关键词
Bayesian Networks,Evolutionary Optimization,Structural Learning
AI 理解论文
溯源树
样例
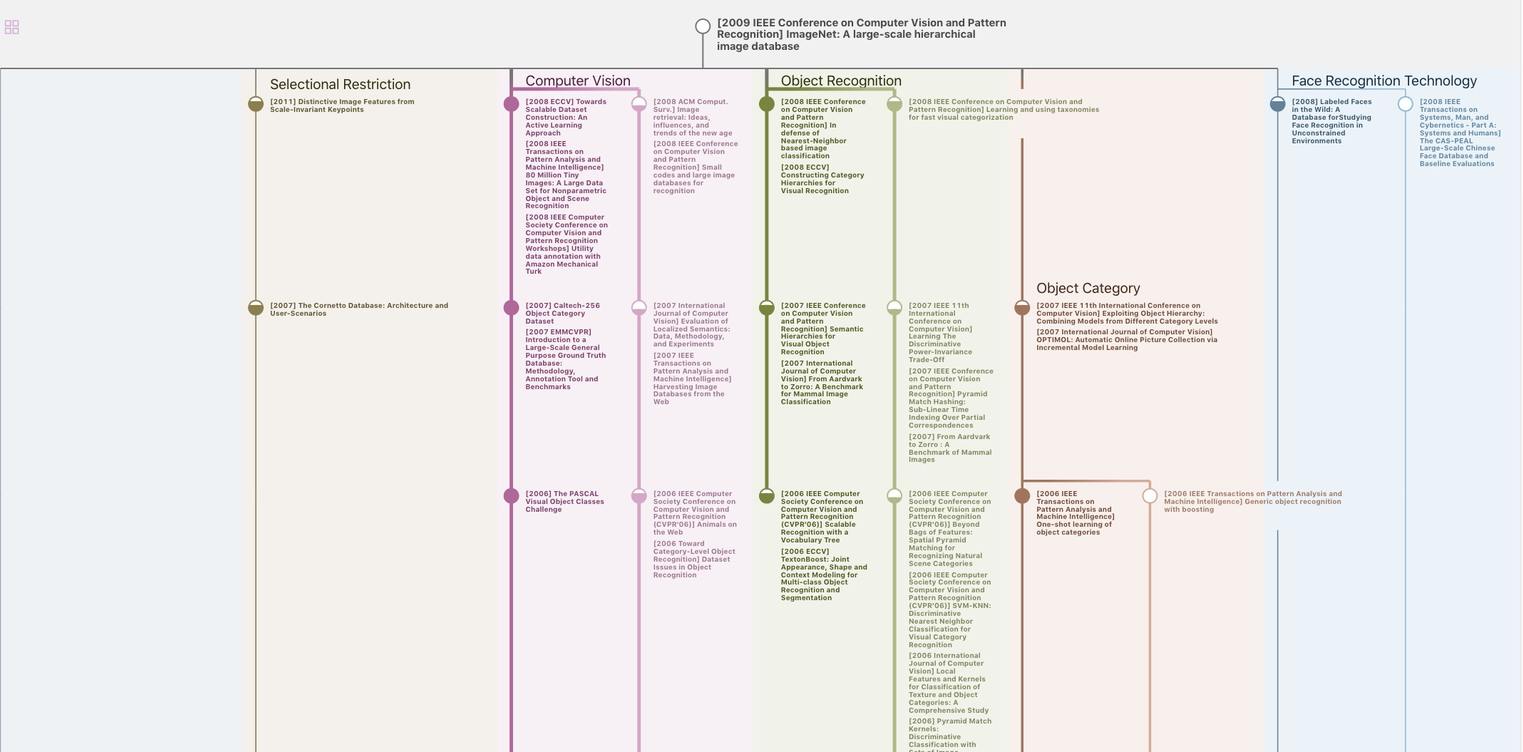
生成溯源树,研究论文发展脉络
Chat Paper
正在生成论文摘要