Synthesizing Effective Diagnostic Models from Small Samples using Structural Machine Learning: a Case Study in Automating COVID-19 Diagnosis
PROCEEDINGS OF THE 2023 GENETIC AND EVOLUTIONARY COMPUTATION CONFERENCE COMPANION, GECCO 2023 COMPANION(2023)
摘要
The global COVID-19 pandemic has demonstrated the urgent need for diagnostic tools that can be both readily applied and dynamically calibrated by non-specialists, in terms of a sensitivity/specificity tradeoff that complies with relevant healthcare policies and procedures. This article describes the design and deployment of a novel machine learning algorithm, Structural Machine Learning (SML), that combines memetic grammar-guided program synthesis with self-supervised learning in order to learn effectively from small data sets while remaining relatively resistant to overfitting. SML is used to construct a signal processing pipeline for audio time-series, which then serves as the diagnostic mechanism for a wide-spectrum, infrasound-to-ultrasound e-stethoscope. In blind trials supervised by a third party, SML is shown to be superior to Deep Learning approaches in terms of the area under the ROC curve, while allowing for transparent interpretation of the decision-making process.
更多查看译文
关键词
machine learning,genetic programming,structural machine learning,domain-specific languages,COVID-19
AI 理解论文
溯源树
样例
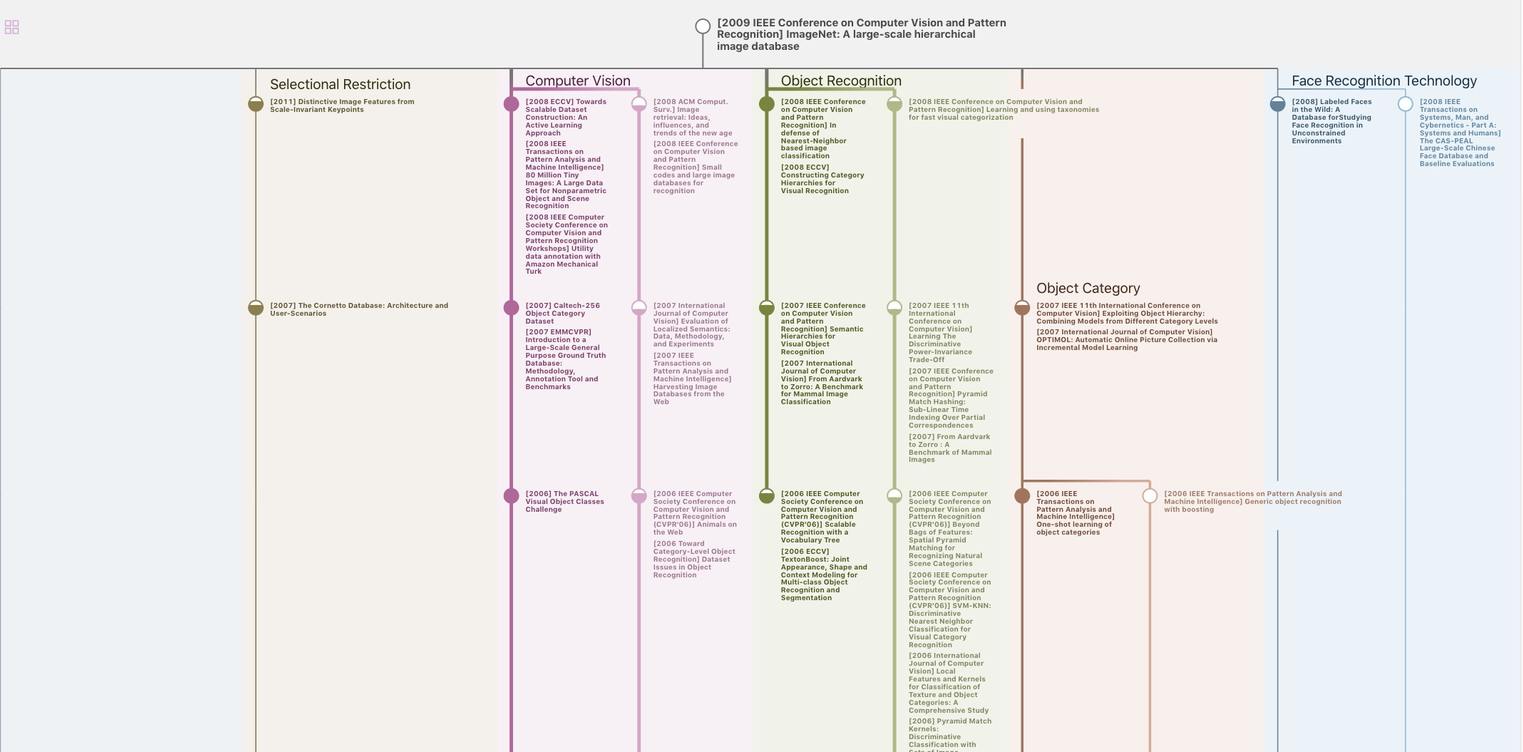
生成溯源树,研究论文发展脉络
Chat Paper
正在生成论文摘要