A Self-Attention Based Task-Adaptive Integration of Pre-trained Global and Local Classifiers for Few-Shot Classification.
CSCWD(2023)
摘要
The few-shot image classification task aims to train a model to correctly classify unlabeled samples when only a few example images are available. Most current metric-based approaches consider only a single task and ignore the variability between tasks. In this paper, we extract potential information of images from both local and global levels by training feature extractors sensitive to global and local features. Then, the multilevel features are optimized further by employing a self-attention mechanism to assign suitable weights based on the target tasks’ characteristics. Extensive experiments on several benchmark datasets show that the proposed model has better generalization ability for different tasks, and our strategy improves by 4%~6% over the baseline, showing satisfactory results. Our code is available at: https://github.com/XiangLi0503/MTnet.
更多查看译文
关键词
Image classification,few-shot learning,self-attention,feature fusion,transfer learning
AI 理解论文
溯源树
样例
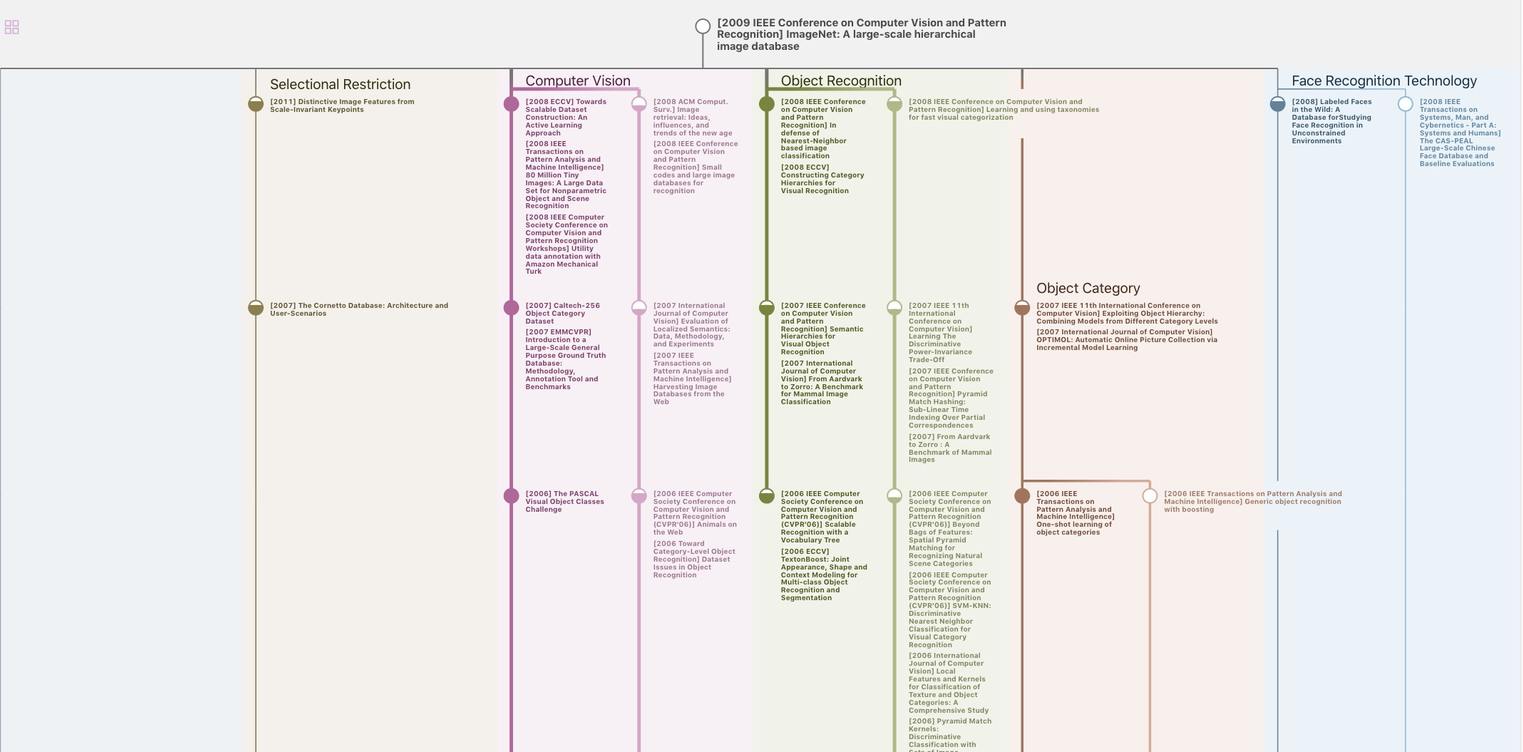
生成溯源树,研究论文发展脉络
Chat Paper
正在生成论文摘要