Curvature-Driven Knowledge Graph Embedding for Link Prediction.
CSCWD(2023)
摘要
Knowledge Graph Embedding (KGE) aims to learn how to represent the low-dimensional vectors for entities and relations based on the observed triplets in knowledge graph. Most of the existing models use simple structural features, such as node degrees and directed edges, and pay little attention to advanced inherent information of structured knowledge. In this paper, we propose CD-GCN, a curvature-driven KGE method for link prediction. Specifically, we first apply Ricci curvature to knowledge graph. Then, we use curvature information to drive the state update, which aims to further exploit the graph-structured information. Finally, we use a ConvE scoring function to output the link prediction results. Through extensive experiments on public datasets FB15k-237 and WN18RR, CD-GCN has achieved state-of-the-art results compared with all baseline models.
更多查看译文
关键词
knowledge graph embedding,graph convolutional network,link prediction,ricci curvature
AI 理解论文
溯源树
样例
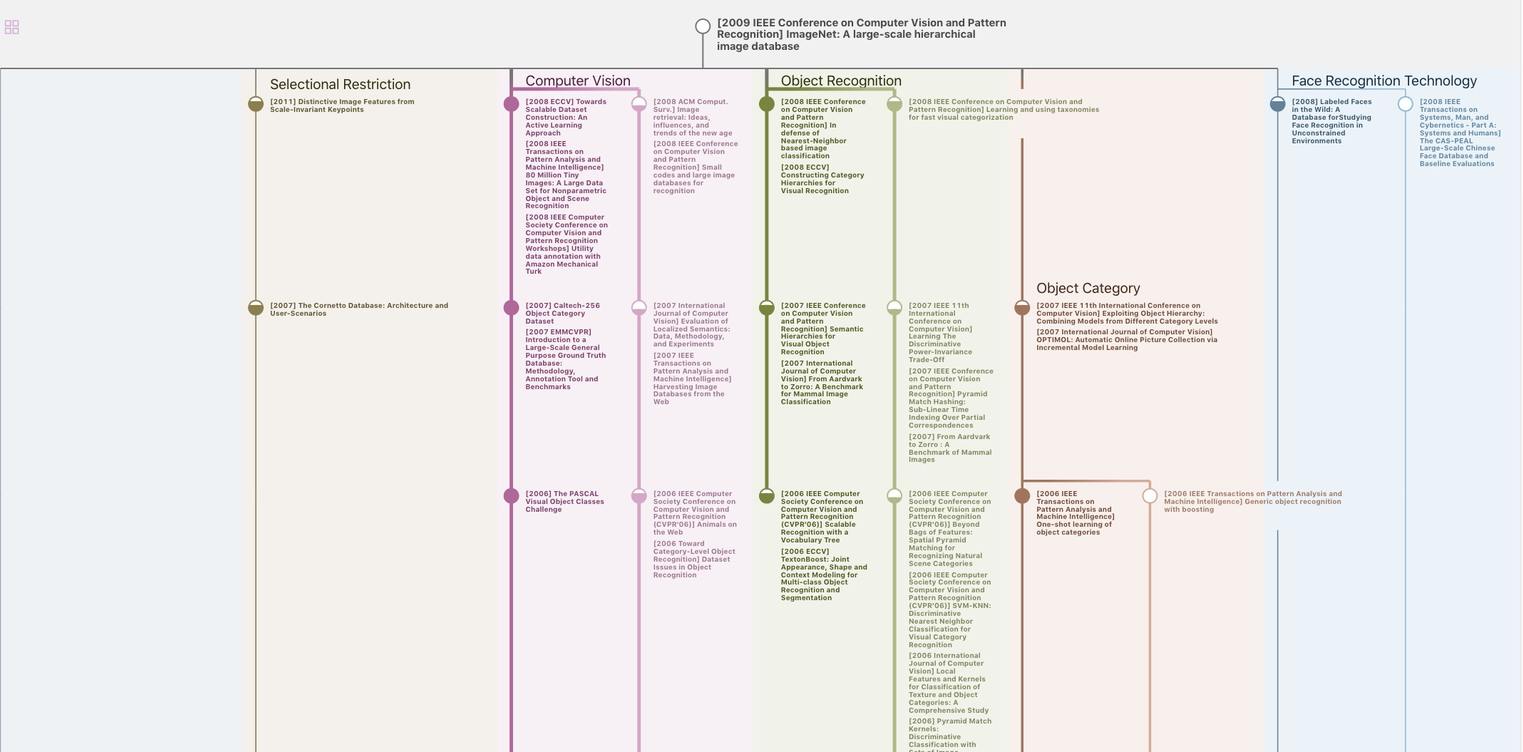
生成溯源树,研究论文发展脉络
Chat Paper
正在生成论文摘要