Decentralized Application Identification via Burst Feature Aggregation.
CSCWD(2023)
摘要
With the development of blockchain technology, de-centralized applications (DApps) are increasingly being developed and deployed on blockchain platforms. However, the complex data validation mechanism and strict encryption protocol settings of blockchain often lead to sparse traffic behavior of DApps. This sparsity poses a challenge for existing encrypted traffic identification methods to extract distinguishable DApps traffic features. In this study, we propose a novel approach for identifying DApps traffic features by observing the differences in burst timing features of DApps. We introduce a continuous burst feature matrix (CBFM) method based on burst feature aggregation that can aggregate sparse features and express the burst timing differences of DApps encrypted traffic. Additionally, we design a deep learning classifier to automatically extract the features contained in the CBFM. Our experimental results on real datasets demonstrate that the proposed CBFM method achieves a classification accuracy of 94%, outperforming state-of-the-art methods.
更多查看译文
关键词
Decentralized applications,encrypted traffic classification,deep learning,blockchain
AI 理解论文
溯源树
样例
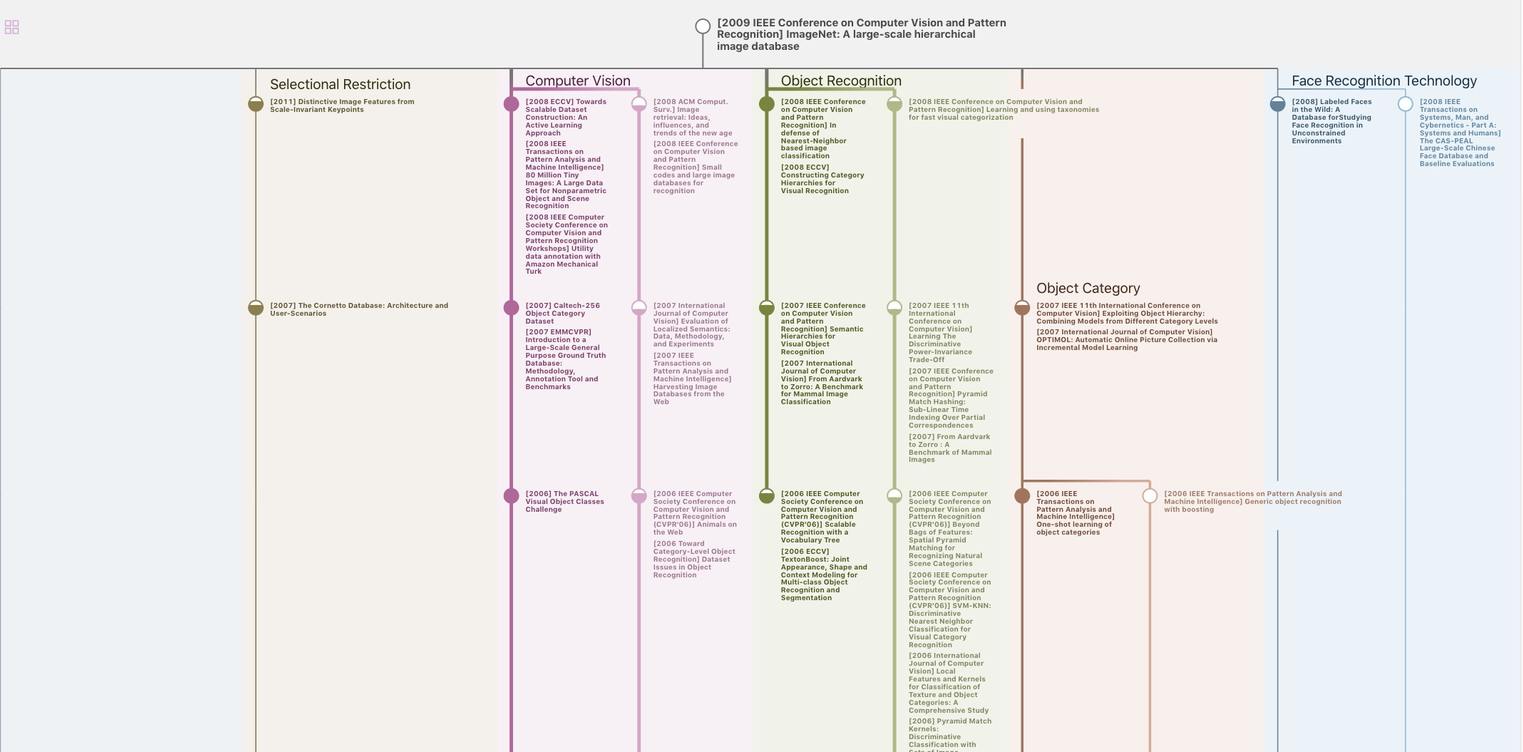
生成溯源树,研究论文发展脉络
Chat Paper
正在生成论文摘要