Knowledge Distillation with Source-free Unsupervised Domain Adaptation for BERT Model Compression.
CSCWD(2023)
摘要
The pre-training language model BERT has brought significant performance improvements to a series of natural language processing tasks, but due to the large scale of the model, it is difficult to be applied in many practical application scenarios. With the continuous development of edge computing, deploying the models on resource-constrained edge devices has become a trend. Considering the distributed edge environment, how to take into account issues such as data distribution differences, labeling costs, and privacy while the model is shrinking is a critical task. The paper proposes a new BERT distillation method with source-free unsupervised domain adaptation. By combining source-free unsupervised domain adaptation and knowledge distillation for optimization and improvement, the performance of the BERT model is improved in the case of cross-domain data. Compared with other methods, our method can improve the average prediction accuracy by up to around 4% through the experimental evaluation of the cross-domain sentiment analysis task.
更多查看译文
关键词
Knowledge Distillation,Unsupervised Domain Adaptation,BERT Model,Model Compression
AI 理解论文
溯源树
样例
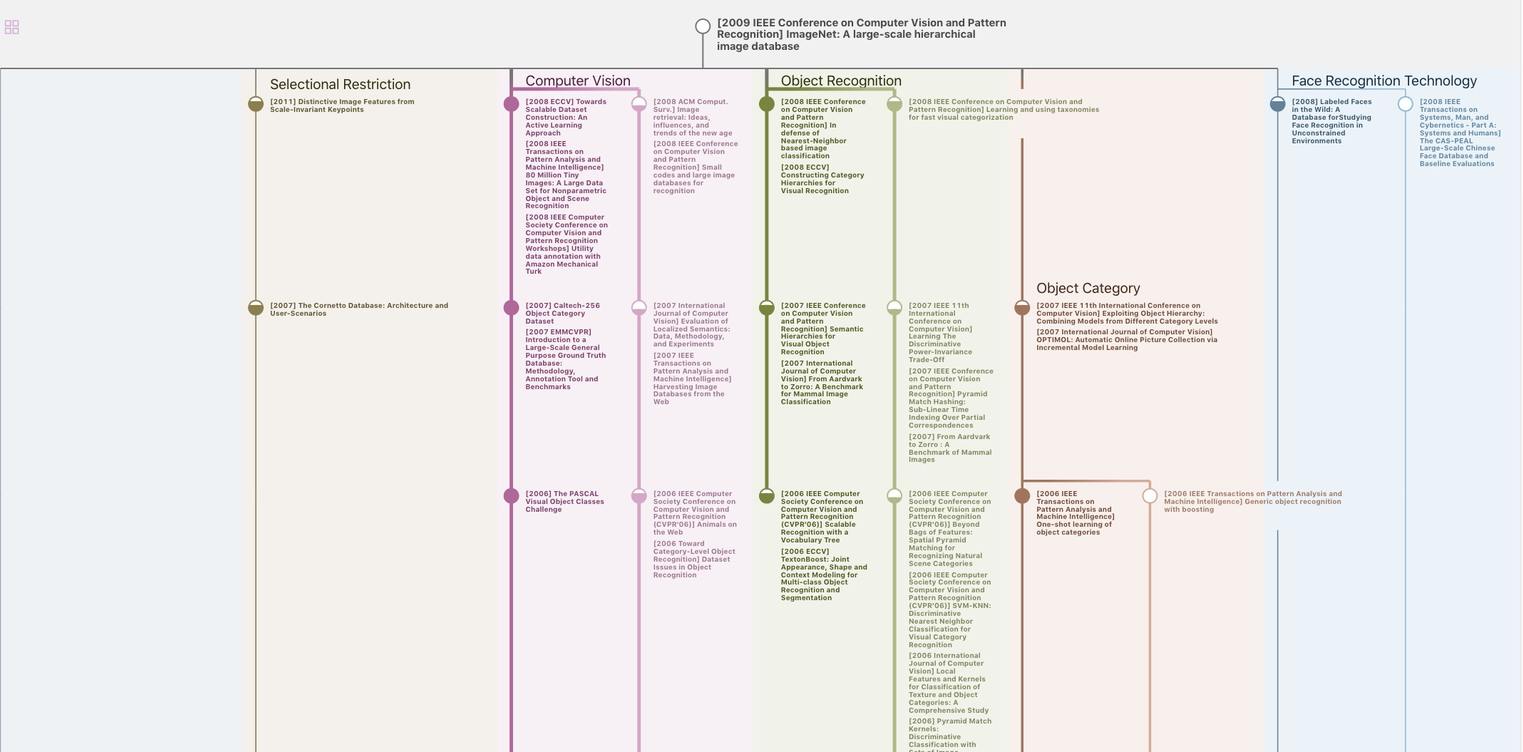
生成溯源树,研究论文发展脉络
Chat Paper
正在生成论文摘要