ATS: A Fully Automatic Troubleshooting System with Efficient Anomaly Detection and Localization.
ICCS (5)(2023)
摘要
As network scale expands and concurrent requests grow, unexpected network anomalies are more frequent, leading to service interruptions and degraded user experience. Real-time, accurate troubleshooting is critical for ensuring satisfactory service. Existing troubleshooting solutions adopt ensemble anomaly detection (EAD) to detect anomalies due to its robustness. However, the fixed base classifier parameters in EAD set by expert experience may reduce the efficiency of anomaly detection when faced with different data distributions. Furthermore, the binary results fed to the secondary classifier in EAD cause information loss, leading to compromised accuracy and inaccurate root cause localization. Besides, key performance indicators (KPIs) are crucial for measuring the system performance, but relying on multiple redundant KPIs to identify the root causes of anomalies is time-consuming and error-prone. To address the above issues, we propose a fully automatic troubleshooting system, ATS. A new EAD method is introduced to detect anomalies, then a module is designed to trigger the root cause localization. Specifically, the EAD method updates the parameters of base classifiers to dynamically adapt to different KPI data distributions. The ensemble of soft labels generated by base classifiers is subsequently fed into the secondary classifier to achieve information-lossless anomaly detection. Then, a heuristic module is proposed to select the most appropriate KPI data based on the metric i.e., bilayer relative difference to trigger the efficient root cause localization. Extensive experiments demonstrate that ATS is more than twice as fast as most state-of-the-art solutions while with higher troubleshooting accuracy.
更多查看译文
关键词
automatic troubleshooting system,efficient anomaly detection,anomaly detection,ats
AI 理解论文
溯源树
样例
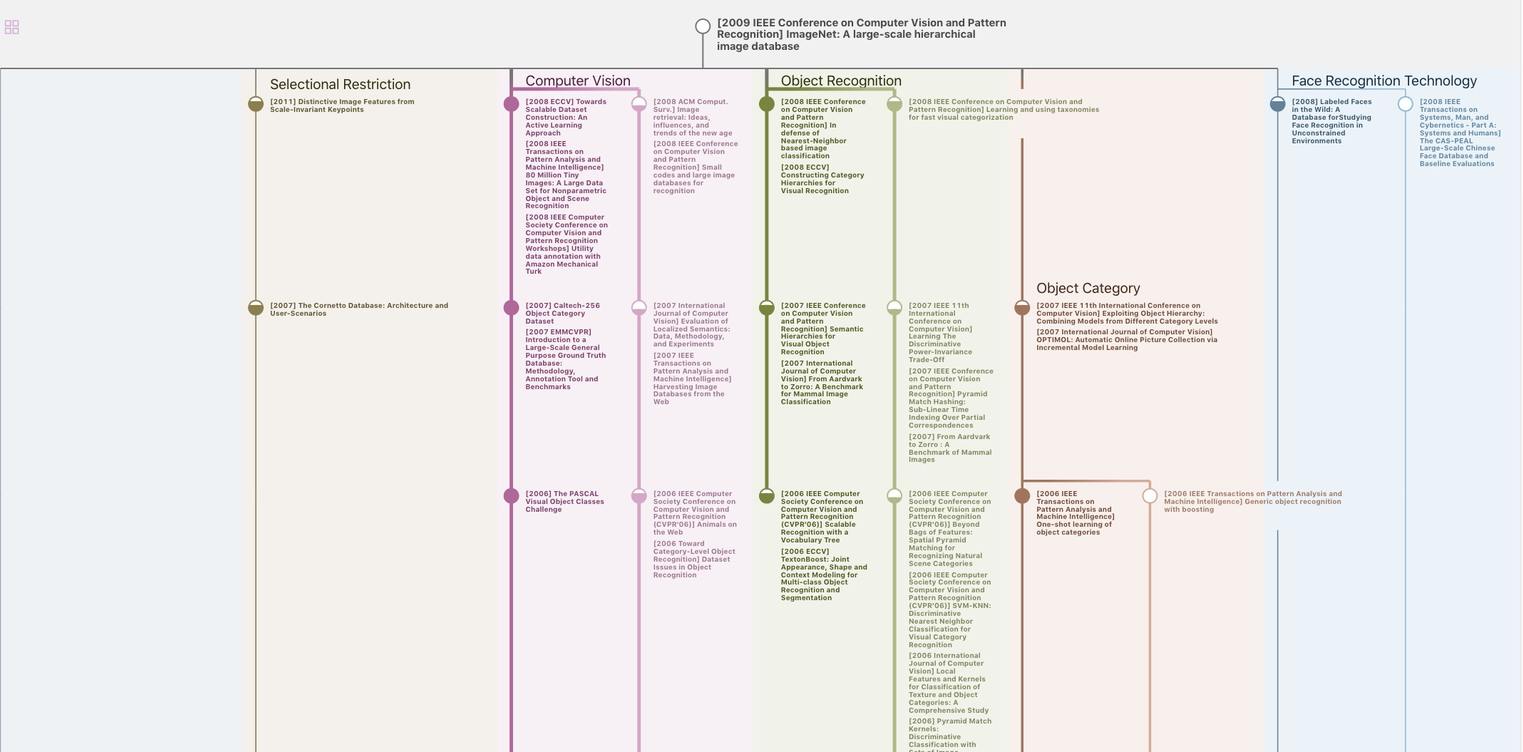
生成溯源树,研究论文发展脉络
Chat Paper
正在生成论文摘要