Learning Shape-Preserving Autoencoder for the Reconstruction of Functional Data from Noisy Observations.
ICCS (2)(2023)
摘要
We propose a new autoencoder preserving input functions’ general shape (monotonicity, convexity) after their reconstruction without imposing a priori constraints. These properties are inherent to the coefficients of the Bernstein-Durrmeyer polynomials that serve here as theoretical descriptors. Their estimates, computed from noisy observations by the coder, play the role of latent variables. The approach is purely nonparametric, i.e., no prior finite-dimensional model is assumed. The answer to the question of how many latent variables should be used for an acceptable reconstruction accuracy of a family of functional data is inferred from learning based on the proposed approximation of the Akaike Information Criterion. A distinguishing feature of this autoencoder is that the coder and encoder are designed as precomputed and stored matrices with the Bernstein polynomial entries. Thus, after selecting the number of latent variables, the autoencoder usage has a low computational complexity since it is linear with respect to observations. The proposed computational algorithms are tested on real data arising in mechanical engineering when control of damping vibrations is necessary.
更多查看译文
关键词
functional data,learning,observations,shape-preserving
AI 理解论文
溯源树
样例
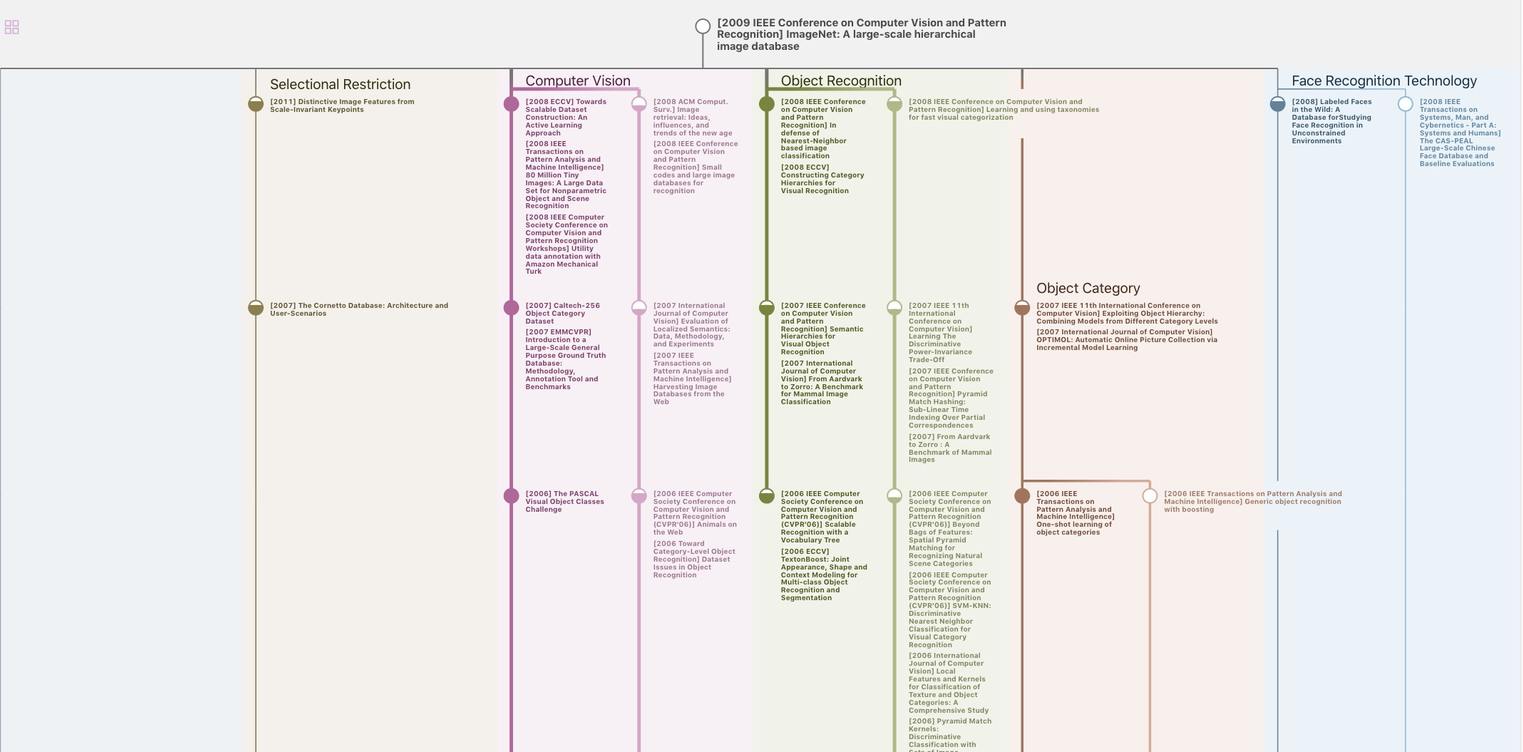
生成溯源树,研究论文发展脉络
Chat Paper
正在生成论文摘要