Self-supervised Deep Heterogeneous Graph Neural Networks with Contrastive Learning.
ICCS (1)(2023)
摘要
Heterogeneous graph neural networks have shown superior capabilities on graphs that contain multiple types of entities with rich semantic information. However, they are usually (semi-)supervised learning methods which rely on costly task-specific labeled data. Due to the problem of label sparsity on heterogeneous graphs, the performance of these methods is limited, prompting the emergence of some self-supervised learning methods. However, most of self-supervised methods aggregate meta-path based neighbors without considering implicit neighbors that also contain rich information, and the mining of implicit neighbors is accompanied by the problem of introducing irrelevant nodes. Therefore, in this paper we propose a self-supervised deep heterogeneous graph neural networks with contrastive learning (DHG-CL) which not only preserves the information of implicitly valuable neighbors but also further enhances the distinguishability of node representations. Specifically, (1) we design a cross-layer semantic encoder to incorporate information from different high-order neighbors through message passing across layers; and then (2) we design a graph-based contrastive learning task to distinguish semantically dissimilar nodes, further obtaining discriminative node representations. Extensive experiments conducted on a variety of real-world heterogeneous graphs show that our proposed DHG-CL outperforms the state-of-the-arts.
更多查看译文
关键词
graph neural networks,contrastive learning,self-supervised
AI 理解论文
溯源树
样例
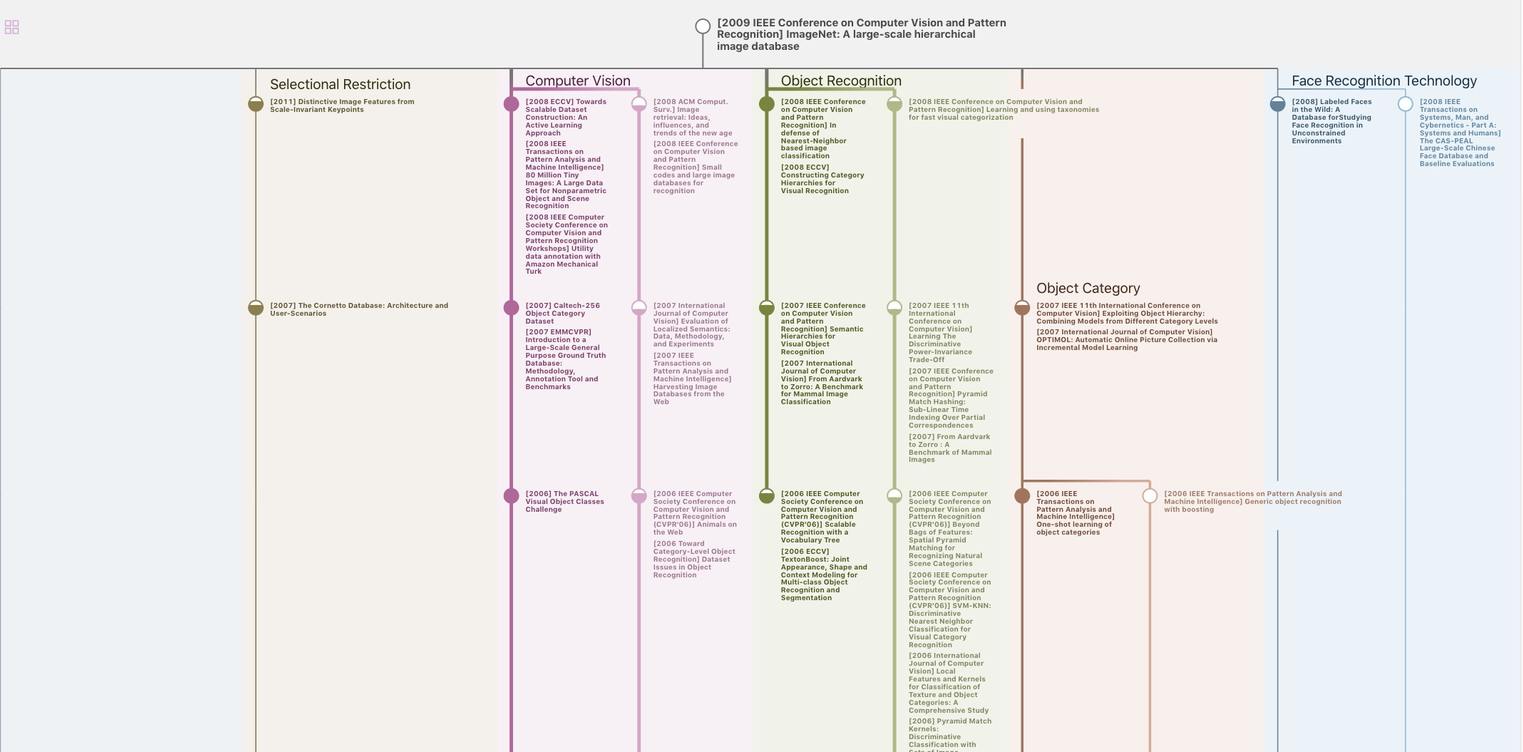
生成溯源树,研究论文发展脉络
Chat Paper
正在生成论文摘要