Bayesian Optimization For Choice Data
PROCEEDINGS OF THE 2023 GENETIC AND EVOLUTIONARY COMPUTATION CONFERENCE COMPANION, GECCO 2023 COMPANION(2023)
摘要
In this work we introduce a new framework for multi-objective Bayesian optimisation where the multi-objective functions can only be accessed via choice judgements, such as "I pick options x(1), x(2), x(3) among this set of five options x(1), x(2),..., x(5)". The fact that the option x(4) is rejected means that there is at least one option among the selected ones x(1), x(2), x(3) that I strictly prefer over x(4) (but I do not have to specify which one). We assume that there is a latent vector function u for some dimension.. which embeds the options into the real vector space of dimension.., so that the choice set can be represented through a Pareto set of non-dominated options. By placing a Gaussian process prior on u and by using a novel likelihood model for choice data, we derive a surrogate model for the latent vector function. We then propose two novel acquisition functions to solve the multi-objective Bayesian optimisation from choice data.
更多查看译文
关键词
multi-objective optimization,Bayesian optimization,choice learning
AI 理解论文
溯源树
样例
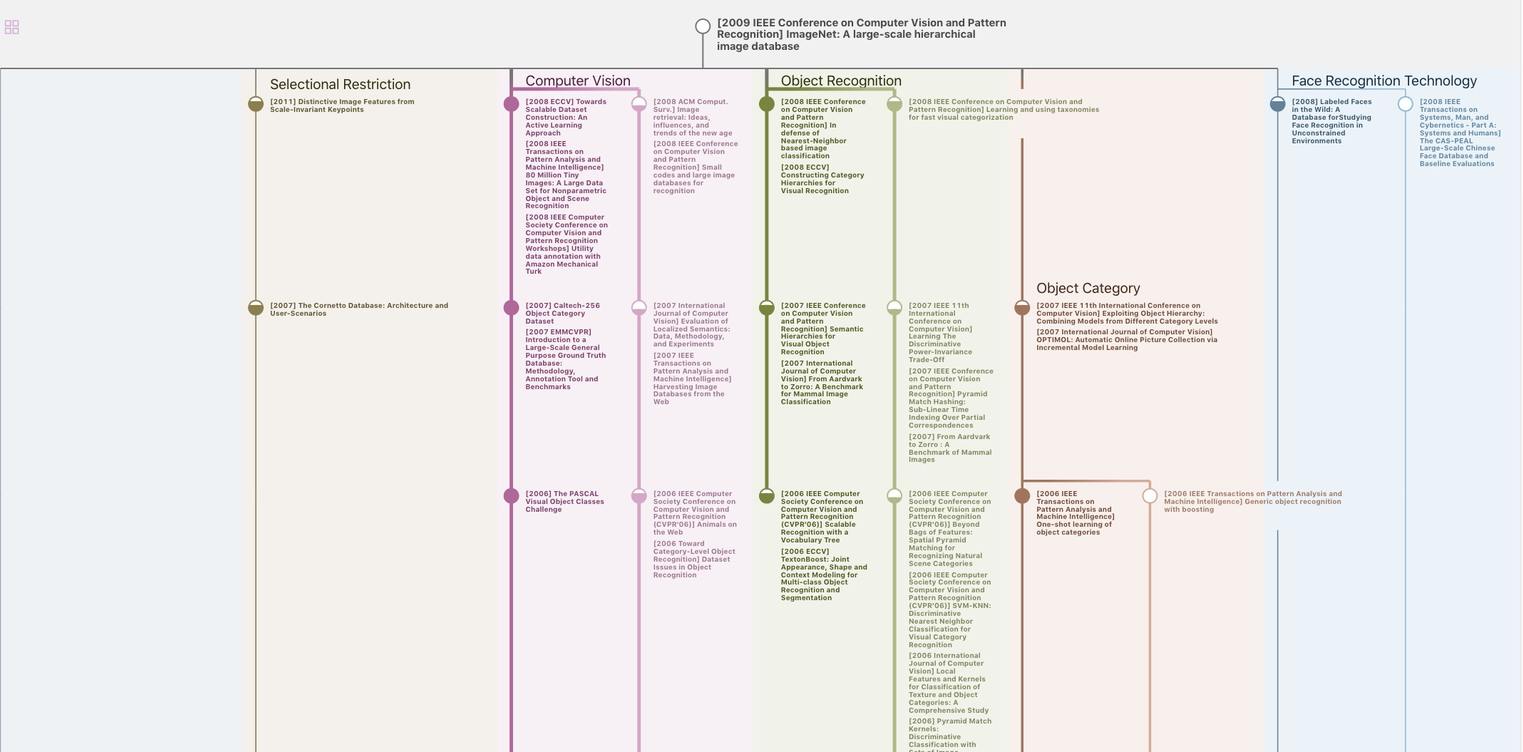
生成溯源树,研究论文发展脉络
Chat Paper
正在生成论文摘要