Improving the Relevance of Artificial Instances for Curriculum-Based Course Timetabling through Feasibility Prediction
PROCEEDINGS OF THE 2023 GENETIC AND EVOLUTIONARY COMPUTATION CONFERENCE COMPANION, GECCO 2023 COMPANION(2023)
摘要
Solvers for Curriculum-Based Course Timetabling were until recently difficult to configure and evaluate because of the limited number of benchmark instances. Recent work has proposed new real-world instances, as well as thousands of generated ones that can be used to train configurators and for machine learning applications. The less numerous real-world instances can then be used as a test set. To assess whether the generated instances exhibit sufficiently similar behavior to the real ones, we choose to consider a basic indicator: feasibility. We find that 38 % of the artificial instances are infeasible versus 6 % of real-world ones, and show that a feasibility prediction model trained on artificial instances performs extremely poorly on real-world ones. The objective of this paper is therefore to be able to predict which generated instances behave like the real-world instances in order to improve the quality of the training set. As a first step, we propose a selection procedure for the artificial training set that produces a feasibility prediction model that works as well as if it were trained on real-world instances. Then, we propose a pipeline to build a selection model that picks artificial instances that match the infeasibility behavior of the real-world ones.
更多查看译文
关键词
University timetabling,feasibility prediction,real-world instances,synthetic instances
AI 理解论文
溯源树
样例
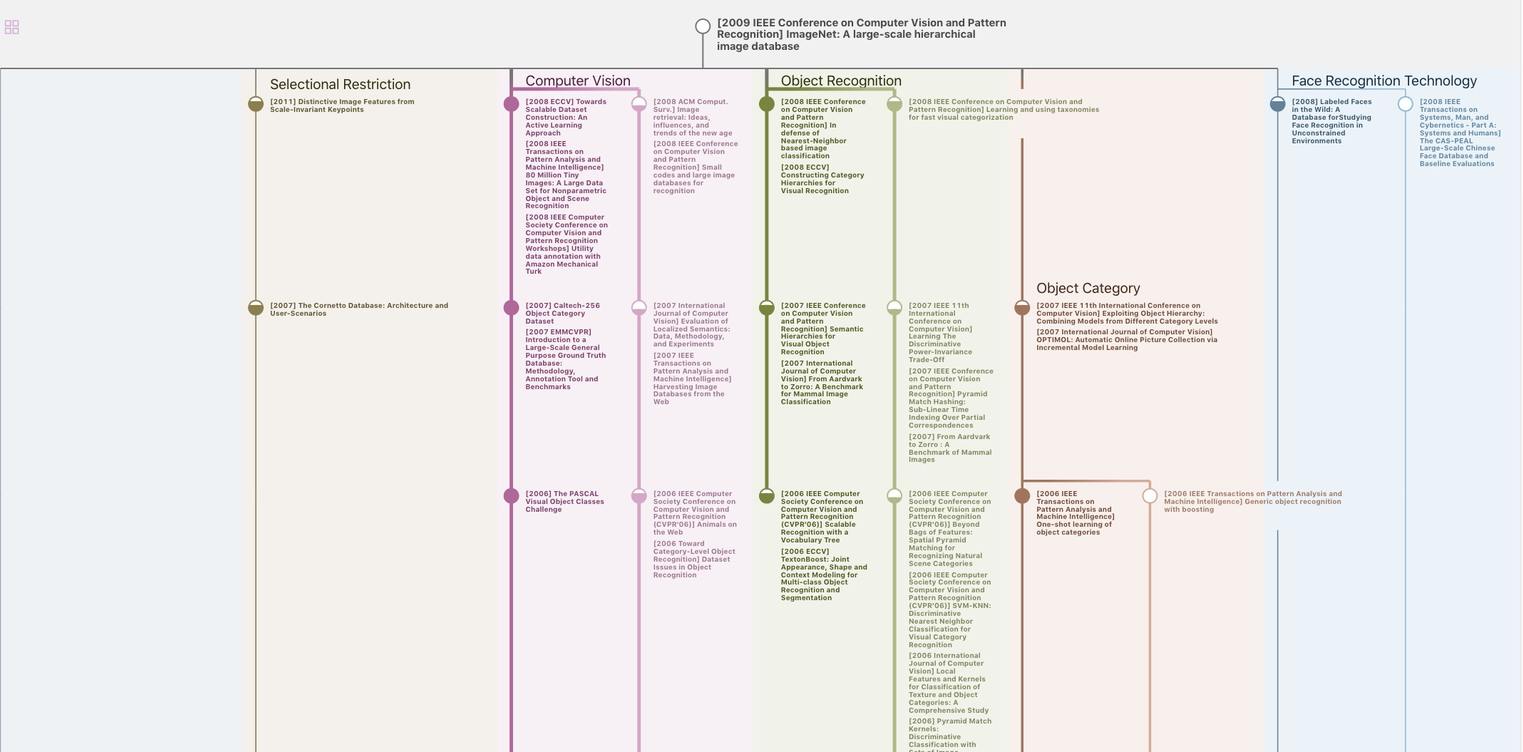
生成溯源树,研究论文发展脉络
Chat Paper
正在生成论文摘要