A Dynamic Partial Update for Covariance Matrix Adaptation
PROCEEDINGS OF THE 2023 GENETIC AND EVOLUTIONARY COMPUTATION CONFERENCE COMPANION, GECCO 2023 COMPANION(2023)
摘要
Tackling large-scale and ill-conditioned problems is demanding even for the covariance matrix adaptation evolution strategy (CMA-ES), which is a state-of-the-art algorithm for black-box optimization. The coordinate selection is a technique that mitigates the ill-conditionality of large-scale problems by updating parameters in partially selected coordinate spaces. This technique can be applied to various CMA-ES variants and improves their performance especially for ill-conditioned problems. However, it often fails to improve the performance ofwell-conditioned problems, because it is difficult to choose appropriate coordinate spaces according to the ill-conditionality of problems. We introduce a dynamic partial update method for coordinate selection to solve the above problem. We use the second-order partial derivatives of an objective function to estimate the condition number and select coordinates so that the condition number of each pair does not exceed the given allowable value. In this method, the number of clusters becomes to be small for well-conditioned problems and large for ill-conditioned cases. In particular, the selection does not execute if the condition number of the full space is less than the allowable value. We observe significant improvements in well-conditioned problems and comparable performances in ill-conditioned cases in numerical experiments.
更多查看译文
关键词
Covariance Matrix Adaptation,Large scale optimization
AI 理解论文
溯源树
样例
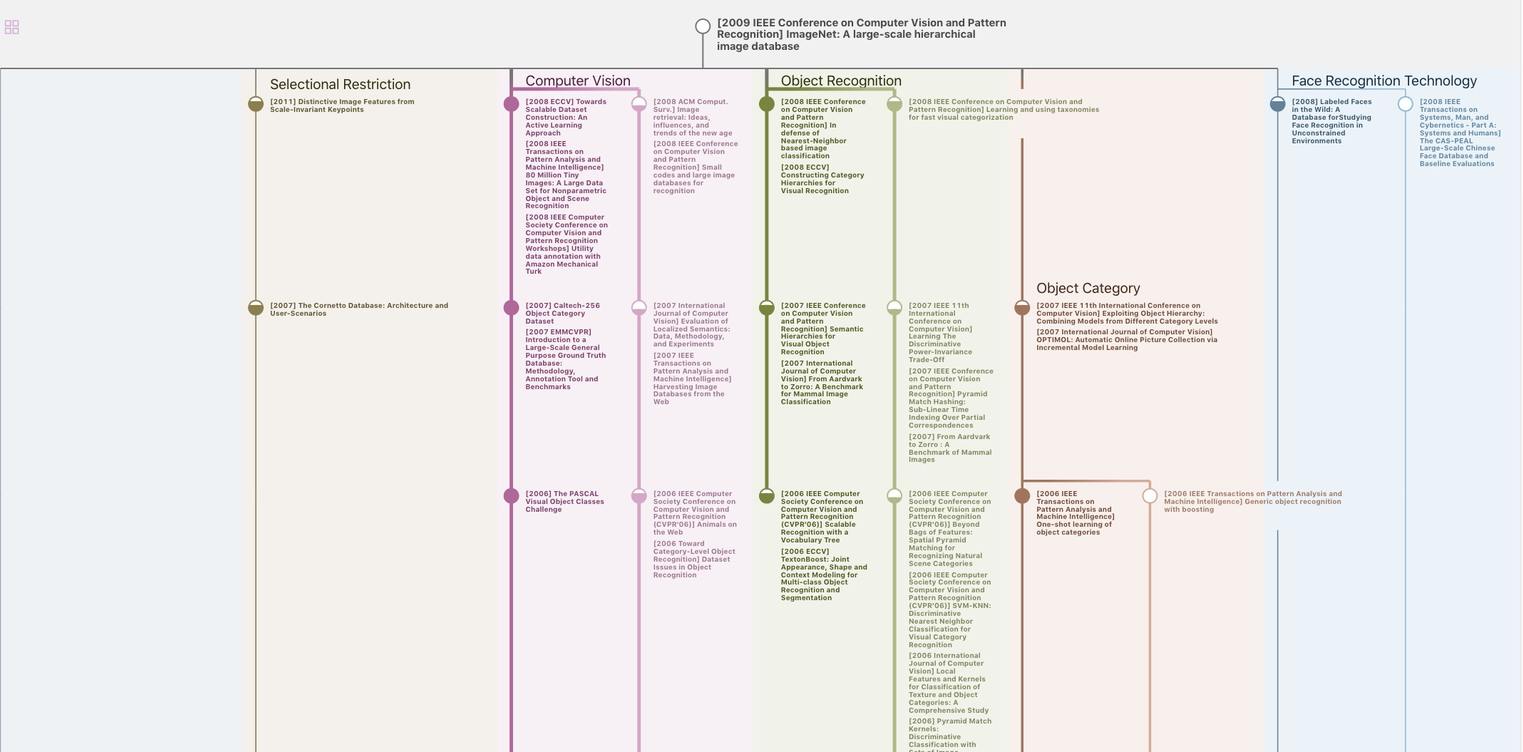
生成溯源树,研究论文发展脉络
Chat Paper
正在生成论文摘要