Vision Transformer for Pneumonia Classification in X-ray Images.
PROCEEDINGS OF 2023 8TH INTERNATIONAL CONFERENCE ON INTELLIGENT INFORMATION TECHNOLOGY, ICIIT 2023(2023)
Key words
Convolution Neural Network,Vision Transformer,Residual Neural Network,Pneumonia,Classification
AI Read Science
Must-Reading Tree
Example
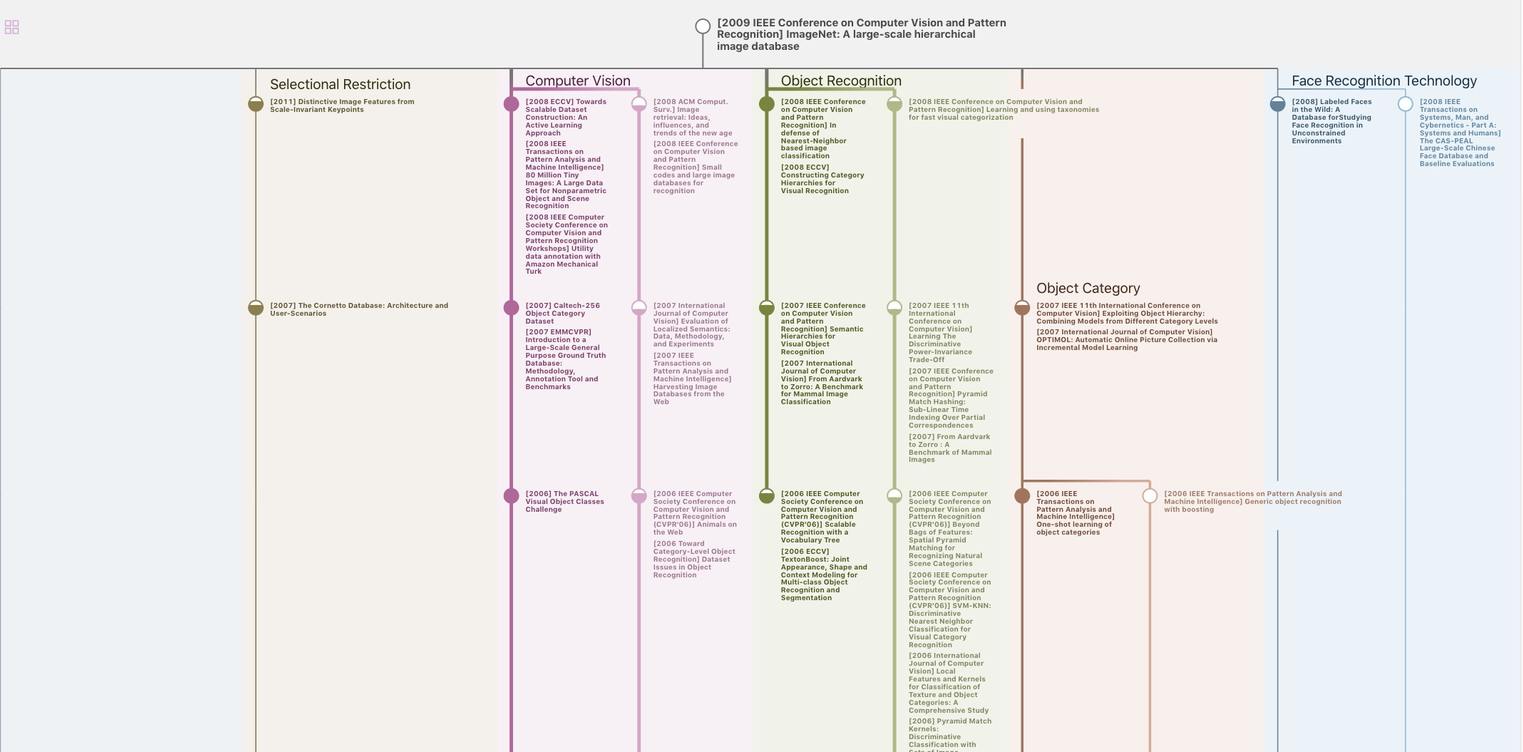
Generate MRT to find the research sequence of this paper
Chat Paper
Summary is being generated by the instructions you defined