Diagnosis of Alzheimer's disease and Mild Cognitive Impairment using Rethinking and Deep Neural Networks.
DSP(2023)
摘要
The early diagnosis of Alzheimer’s Disease (AD) and its prodromal stage, Mild Cognitive Impairment (MCI), are important for doctors to treat patients as soon as possible. In this paper we focus exclusively on the use of EEG for the Machine Learning based diagnosis of MCI and AD. The choice of EEG is made because this modality is relatively inexpensive, non-invasive and easily available even outside the clinical environment. Unlike the majority of ML algorithms for AD diagnosis which treat EEG as an 1-D signal we propose a 2-D approach. We first split the EEG channel signals into fixed length segments and then we reshape them into 2-D images used to train a powerful VGG-16 deep Convolutional Neural Network model. In order to improve the performance of the VGG-16 model and avoid overfitting, we employed a powerful pruning method called Rethinking.We study two experimental tasks: (a) classifying segments into AD/MCI or Health Control (HC) categories (b) classifying subjects (patients and healthy individuals) into the same categories. Our results showed that the proposed method can be used as a tool to diagnose AD at early stages with accuracy over 98.8.
更多查看译文
关键词
AD diagnosis,Alzheimer disease,clinical environment,deep neural networks,EEG channel signals,fixed length segments,health control categories,mild cognitive impairment,ML algorithms,powerful pruning method,prodromal stage,VGG-16 deep convolutional neural network model
AI 理解论文
溯源树
样例
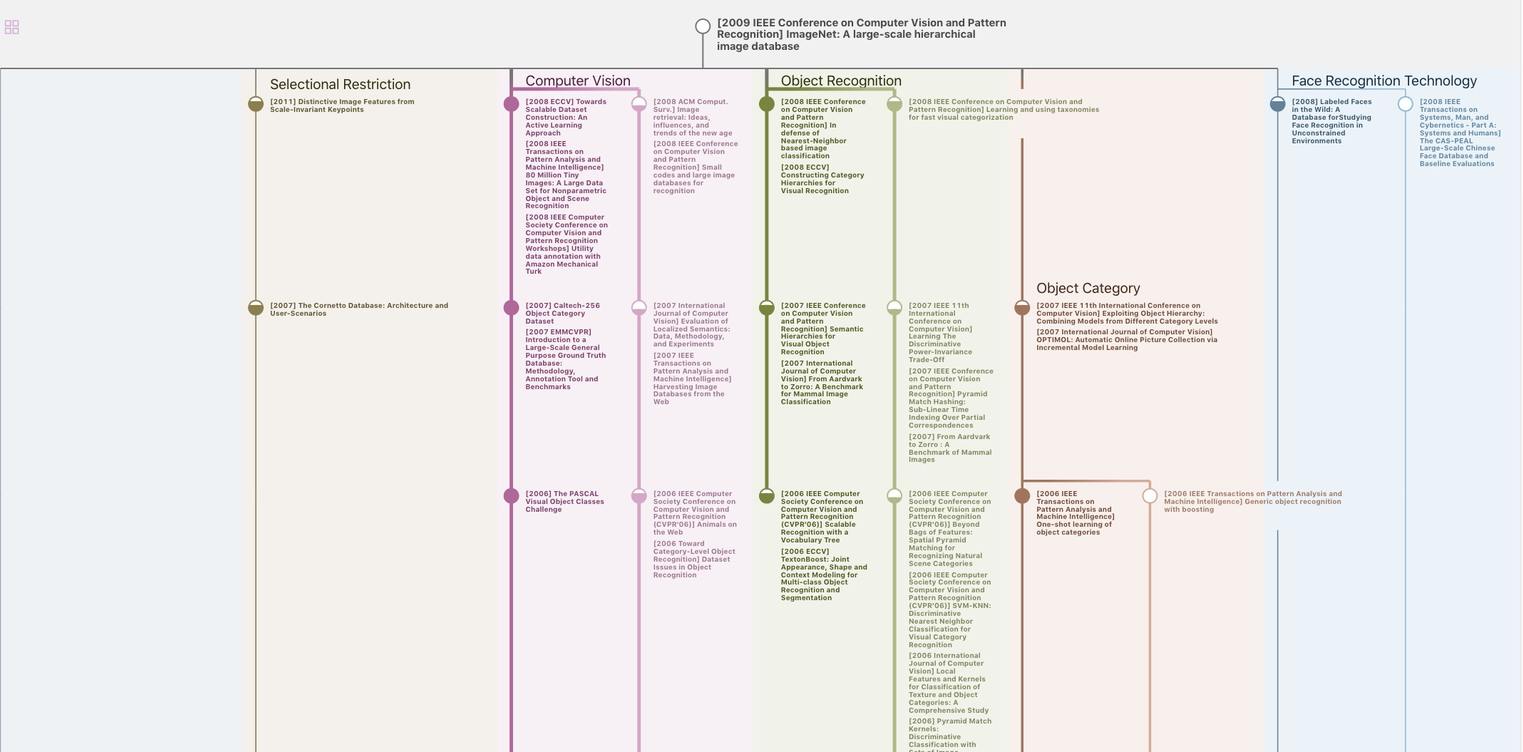
生成溯源树,研究论文发展脉络
Chat Paper
正在生成论文摘要