Predicting Stance to Detect Misinformation in Few-shot Learning.
TAS(2023)
摘要
Stance is an important insight to perceive and understand the public's attitude of opinion towards a topic, e.g., support (stance) of vaccines (target). With a large amount of data available on Twitter to be collected, it provides an opportunity for researchers to make use of past data to develop and explore effective methods in machine learning. However, developing a model to achieve stance classification can be time-consuming due to the requirement to obtain high-quality labelled data to achieve a significant result in supervised learning. Hence, our work aims to study few-shot stance classification and proposed a framework to address the challenges of limited annotated labels. This type of system also tends to lack in explaining its trustworthiness and transparency during the training and decision-making process. Our research emphasises bridging the gap between humans and machines to enable thorough understanding via a collaborative process. This paper presents our few-shot learning setting and approach to train a base learner on extensive similar few-shot tasks using only a few labelled samples, utilising this type of semi-supervised technique to exploit unlabelled data and effectively identify the stance on Twitter with the supervision of a human-in-the-loop.
更多查看译文
关键词
stance classification, few-shot learning, online social networks, human-in-the-loop
AI 理解论文
溯源树
样例
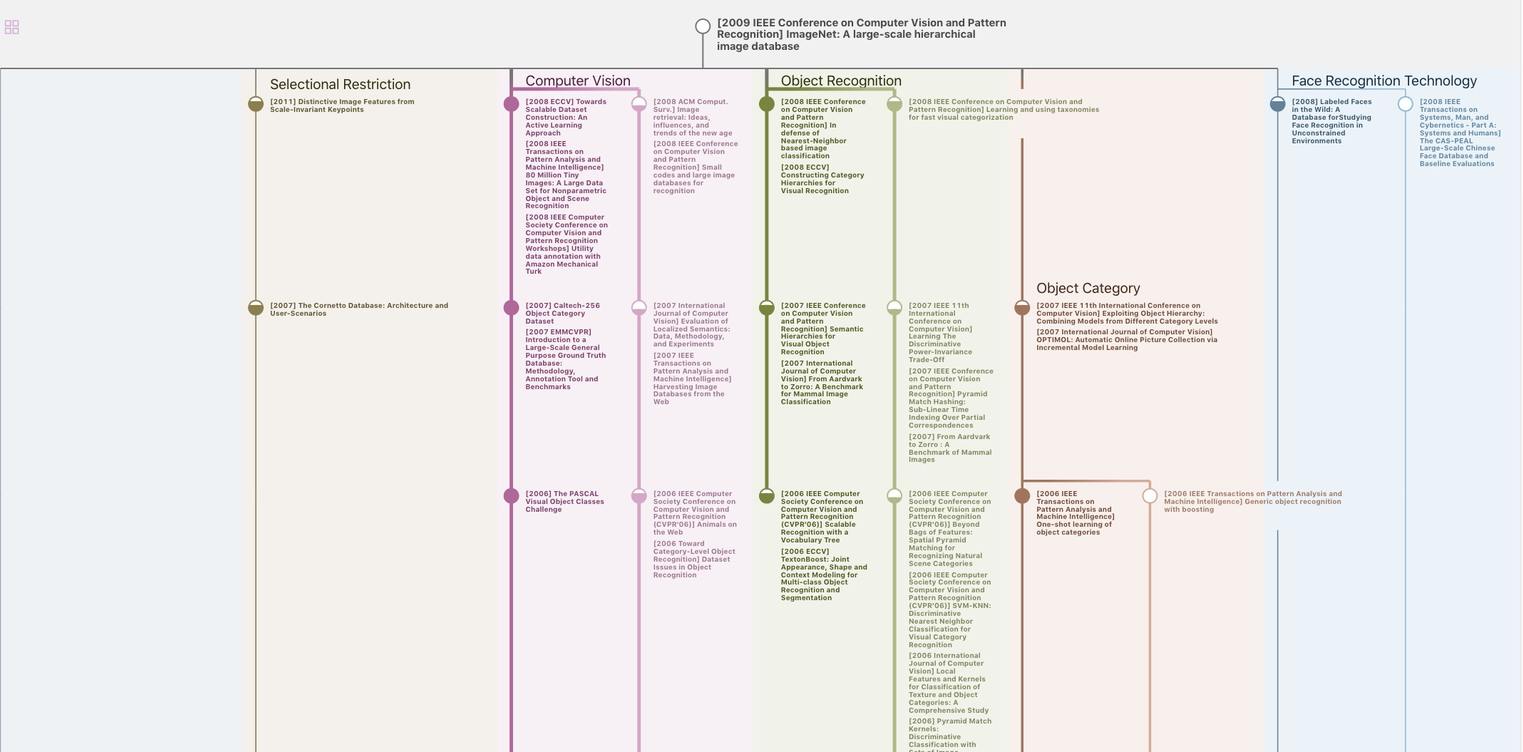
生成溯源树,研究论文发展脉络
Chat Paper
正在生成论文摘要