A Novel Duo-Stage driven Deep Neural Network Approach for Mitigating Electrode Shift Impact on Myoelectric Pattern Recognition Systems.
MeMeA(2023)
摘要
A major barrier to the commercialization of pattern recognition (PR)-based myoelectric prostheses is the lack of robustness to confounding factors such as electrode shift which has been lingering for years. To overcome this challenge, a novel Duo-Stage Convolutional Neural Network (DS-CNN) is proposed. The DS-CNN is comprised of two cascaded stages in which the first stage deciphers the occurrence of a particular kind of shift upon which a requisite CNN model is triggered in the second stage for accurate decoding of individual motion intent, which is necessary for initiating robust control of the prostheses. The proposed scheme works on raw EMG signals as input which reduces the preprocessing time that would be required in conventional machine learning-based PR schemes, to effectively mitigate both transverse and longitudinal shifts using the same network architecture. This approach was validated for four distinct electrode shift conditions (with shifts in the range of 7.50mm-10.05mm) in a dataset obtained from 18 able-bodied subjects that performed 8 classes of targeted hand gestures. The experimental results show that the proposed dual-stage driven deep neural network model can adequately resolve the effects of electrode shift with classification accuracy near the No-shift scenario (< 1.70% difference between shift mitigation and No shift scenarios). These outcomes suggest that our method can provide a practical solution for adaptation to electrode shift, thus improving the robustness of the EMG pattern recognition systems in both clinical and commercial settings.
更多查看译文
关键词
Upper limb prosthesis,Convolution Neural Network,Electromyogram EMG,Electrode Shift
AI 理解论文
溯源树
样例
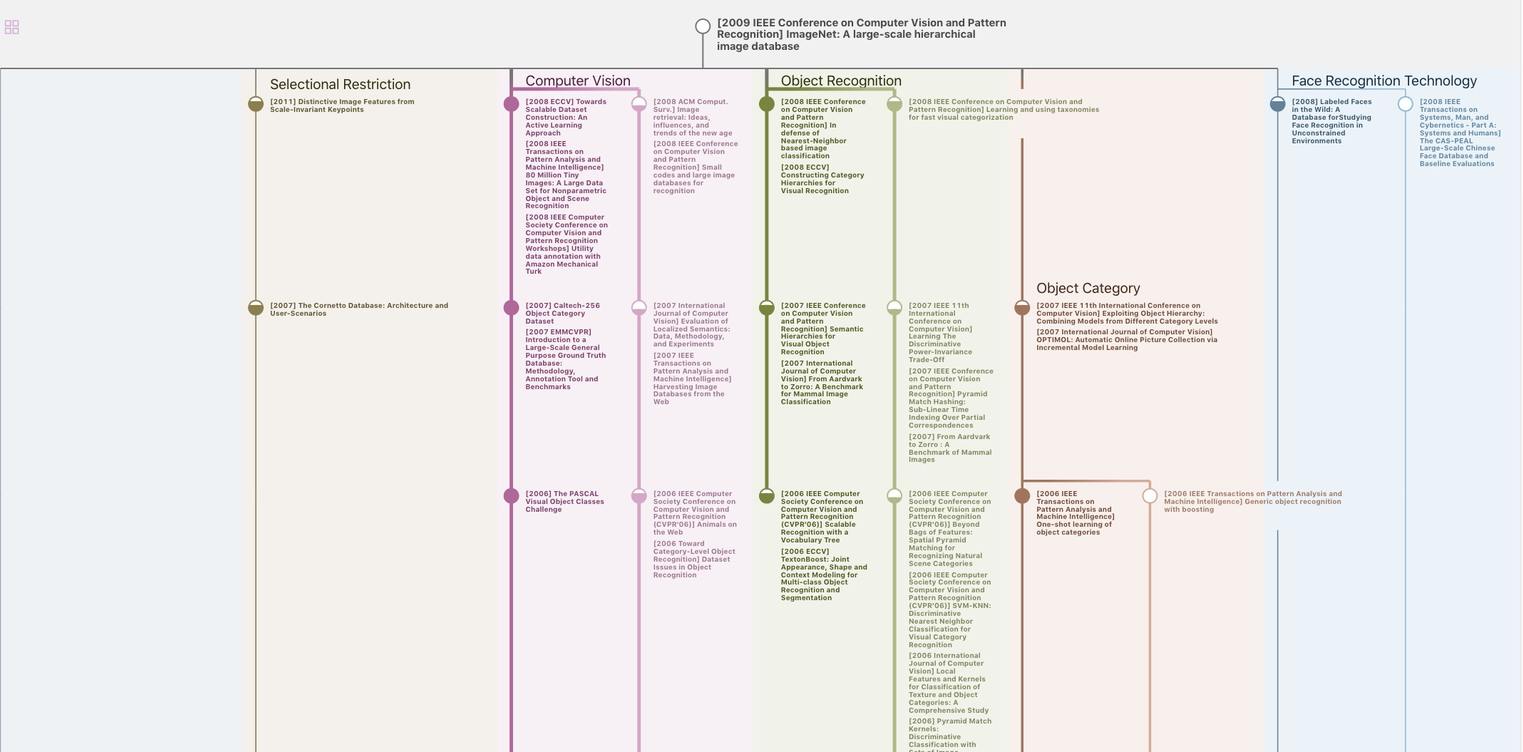
生成溯源树,研究论文发展脉络
Chat Paper
正在生成论文摘要