Prostate cancer risk assessment using a radiogenomic analysis.
MeMeA(2023)
摘要
Prostate cancer (PCa) is a very prevalent cancer type with a heterogeneous prognosis. An accurate assessment of tumor aggressiveness can pave the way for tailored treatment strategies, potentially leading to a better prognosis. Tumor aggressiveness is typically assessed based on invasive methods (e.g. biopsy), but combining diagnostic imaging with genomic information can help uncover aggressive (imaging) phenotypes, which can provide non-invasive advice on individualized treatment regimens. In this study, we aim to identify relevant tumor imaging features from diagnostic multi-parametric MRI sequences, which can then be related to the underlying genomic information derived based on RNA sequencing data. To isolate relevant imaging features that can represent the underlying tumor phenotype, different machine learning models (support vector machine [SVM], k-nearest neighbors [KNN], and logistic regression [LR]) were trained and optimized to classify tumors in either clinically insignificant or significant PCa, based on their Gleason score. These models were trained and validated in two independent cohorts consisting of 45 and 35 patients, respectively. An LR model obtained the highest performance in the validation dataset with a balanced accuracy = 73%, sensitivity = 54%, and specificity = 91%. Significant correlations were found between the identified perfusion-based imaging features and genomic features, highlighting a relationship between imaging characteristics and the underlying genomic information.
更多查看译文
关键词
magnetic resonance imaging,prostate cancer,radiogenomics,machine learning
AI 理解论文
溯源树
样例
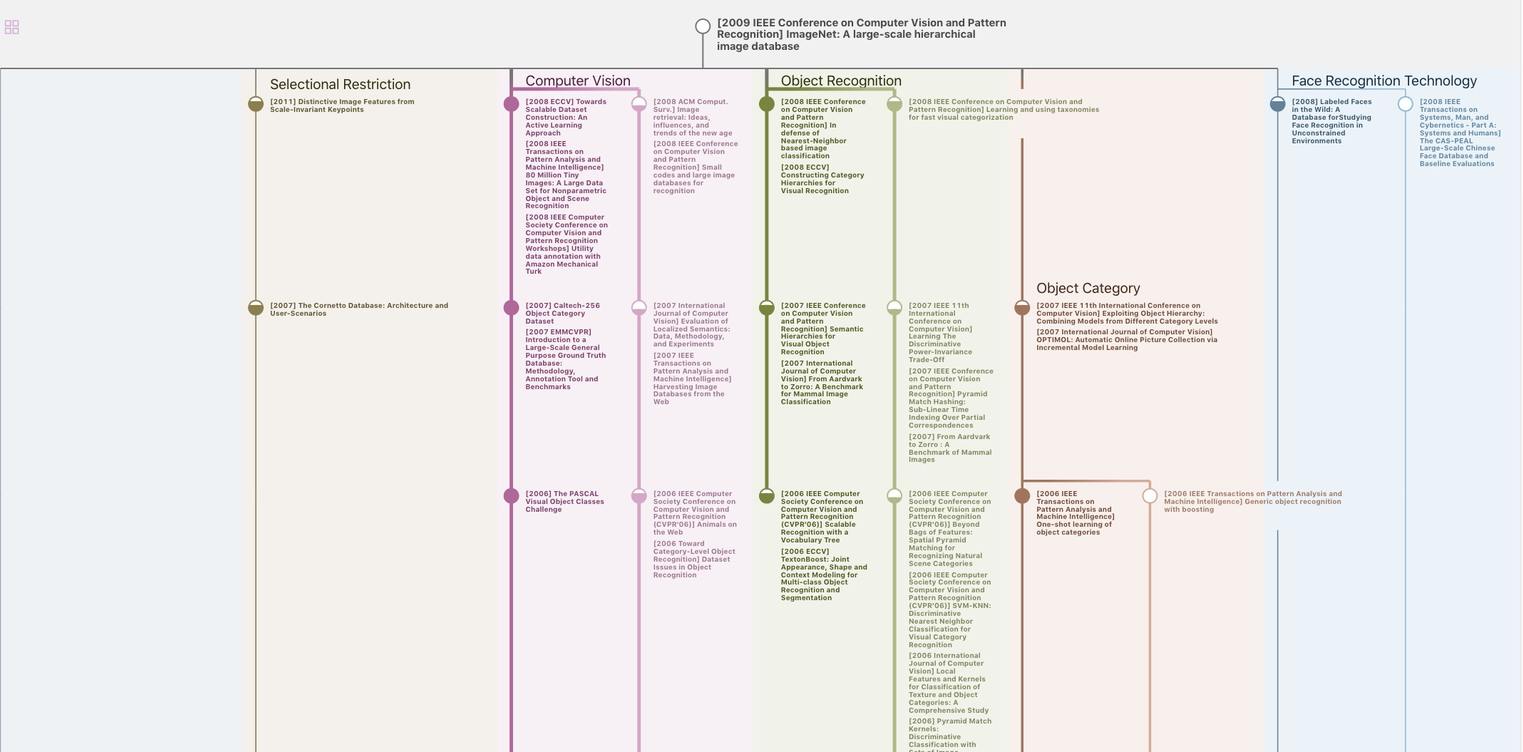
生成溯源树,研究论文发展脉络
Chat Paper
正在生成论文摘要