Multivariate Time Series Anomaly Detection with Deep Learning Models Leveraging Inter-Variable Relationships.
SVCC(2023)
摘要
This paper presents a system for multivariate time series anomaly detection using deep learning, with an added module to reflect variable relationships. The system uses an autoencoder to extract latent variables that reflect the time series characteristics of the variables, and calculates variable importance using the similarities among the variables. To evaluate the proposed method, experiments were conducted using three similarity measures: cosine similarity, distance correlation, and DTW. Four time series datasets were used for evaluation, and the results showed that the proposed model outperformed the baseline model in HAI 22.04 and HAI 21.03 datasets. For the WADI dataset, the F1-score improved only when using cosine similarity, while the TaPR-F1 score improved only when using DTW. However, no performance improvement was observed in the SWaT dataset. These results suggest that the effectiveness of utilizing intervariable relationships is dependent on the characteristics of the data and the similarity calculation method employed. Therefore, a careful selection of the appropriate similarity calculation method for a given dataset is necessary to achieve optimal performance improvements.
更多查看译文
关键词
time-series anomaly detection,GRU,AutoEncoder,latent variable,similarity
AI 理解论文
溯源树
样例
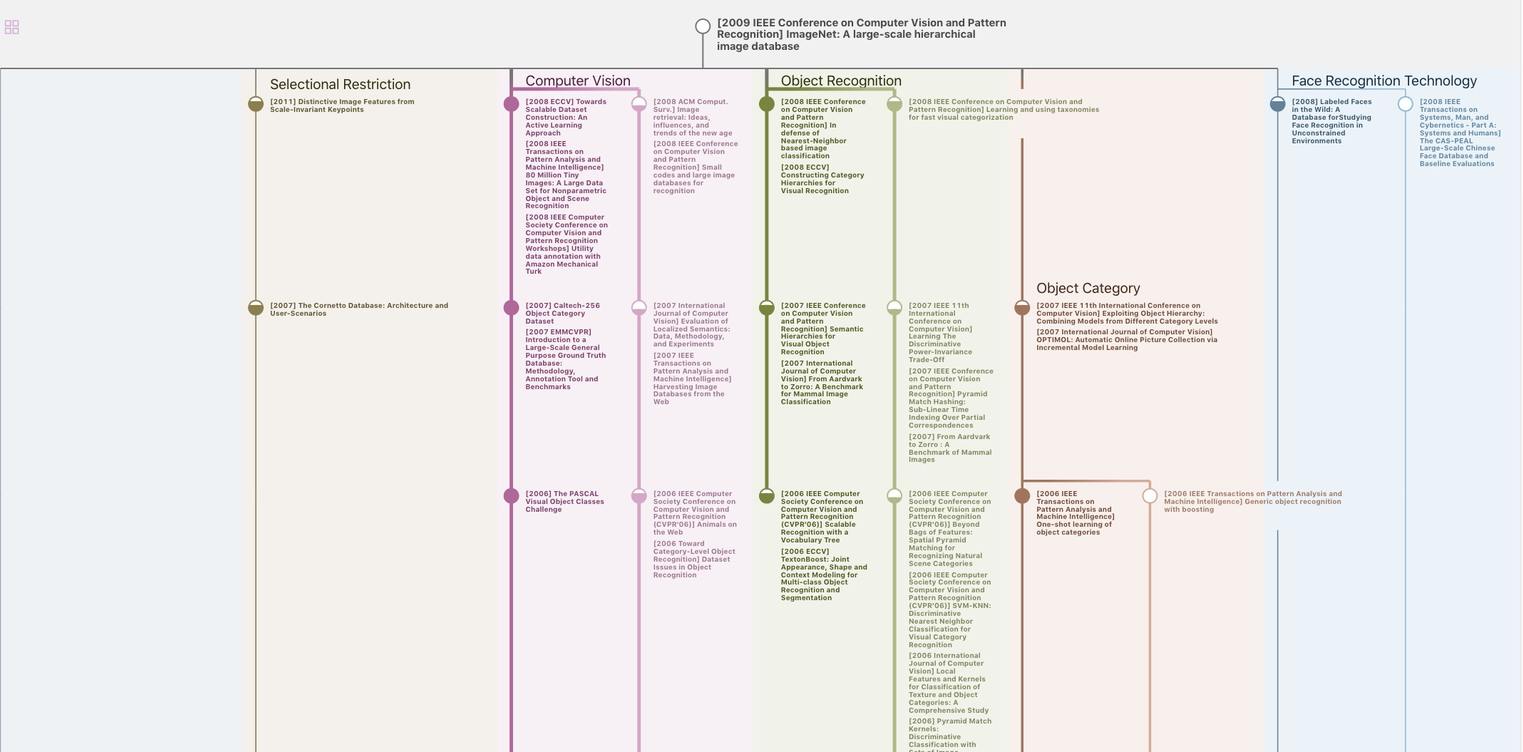
生成溯源树,研究论文发展脉络
Chat Paper
正在生成论文摘要