A Tutorial on Derivative-Free Policy Learning Methods for Interpretable Controller Representations.
2023 AMERICAN CONTROL CONFERENCE, ACC(2023)
摘要
This paper provides a tutorial overview of recent advances in learning control policy representations for complex systems. We focus on control policies that are determined by solving an optimization problem that depends on the current state and some adjustable parameters. We refer to such policies as interpretable in the sense that each of the individual components can be directly understood by practitioners once the parameters are set, i.e., the objective function encodes the desired goal and the constraint functions enforce the rules of the system. We discuss how various commonly used control policies can be viewed in this manner such as the linear quadratic regulator, (nonlinear) model predictive control, and approximate dynamic programming. Traditionally, the parameters that appear in these control policies have been tuned by hand, expert knowledge, or simple trial-and-error experimentation, which can be time consuming and lead to suboptimal results in practice. To this end, we describe how the Bayesian optimization framework, which is a class of efficient derivative-free optimization methods for noisy functions, can be used to efficiently automate this process. In addition to reviewing relevant literature and demonstrating the effectiveness of these new methods on an illustrative example problem, we also offer perspectives on future research in this area.
更多查看译文
AI 理解论文
溯源树
样例
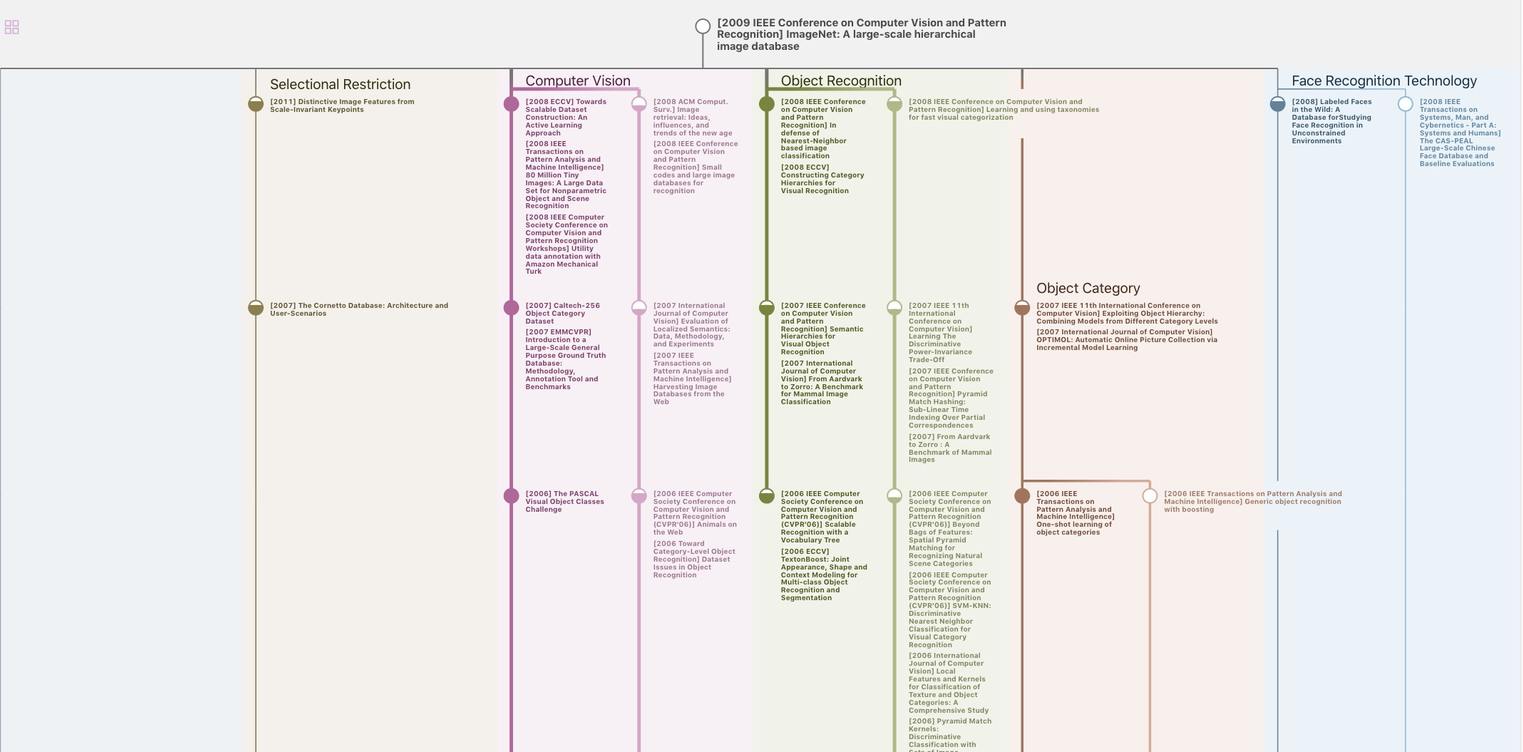
生成溯源树,研究论文发展脉络
Chat Paper
正在生成论文摘要