Closed-Form Active Learning using Expected Variance Reduction of Gaussian Process Surrogates for Adaptive Sampling
2023 AMERICAN CONTROL CONFERENCE, ACC(2023)
摘要
Adaptive sampling of latent fields remains a challenging task, especially in high-dimensional input spaces. In this paper, we propose an active learning method of expected variance reduction with Gaussian process (GP) surrogates using a closed-form gradient. The use of closed-form gradient leads the optimization to find better solutions with reduced computations. We derive the closed-form gradient for active learning Cohn (ALC) using GP surrogates that are formed with the separable squared exponential covariance function. Moreover, we provide algorithmic details for the execution of the closed-form ALC (cALC). Numerical experiments with multiple input space dimensions illustrate the efficacy of our method.
更多查看译文
AI 理解论文
溯源树
样例
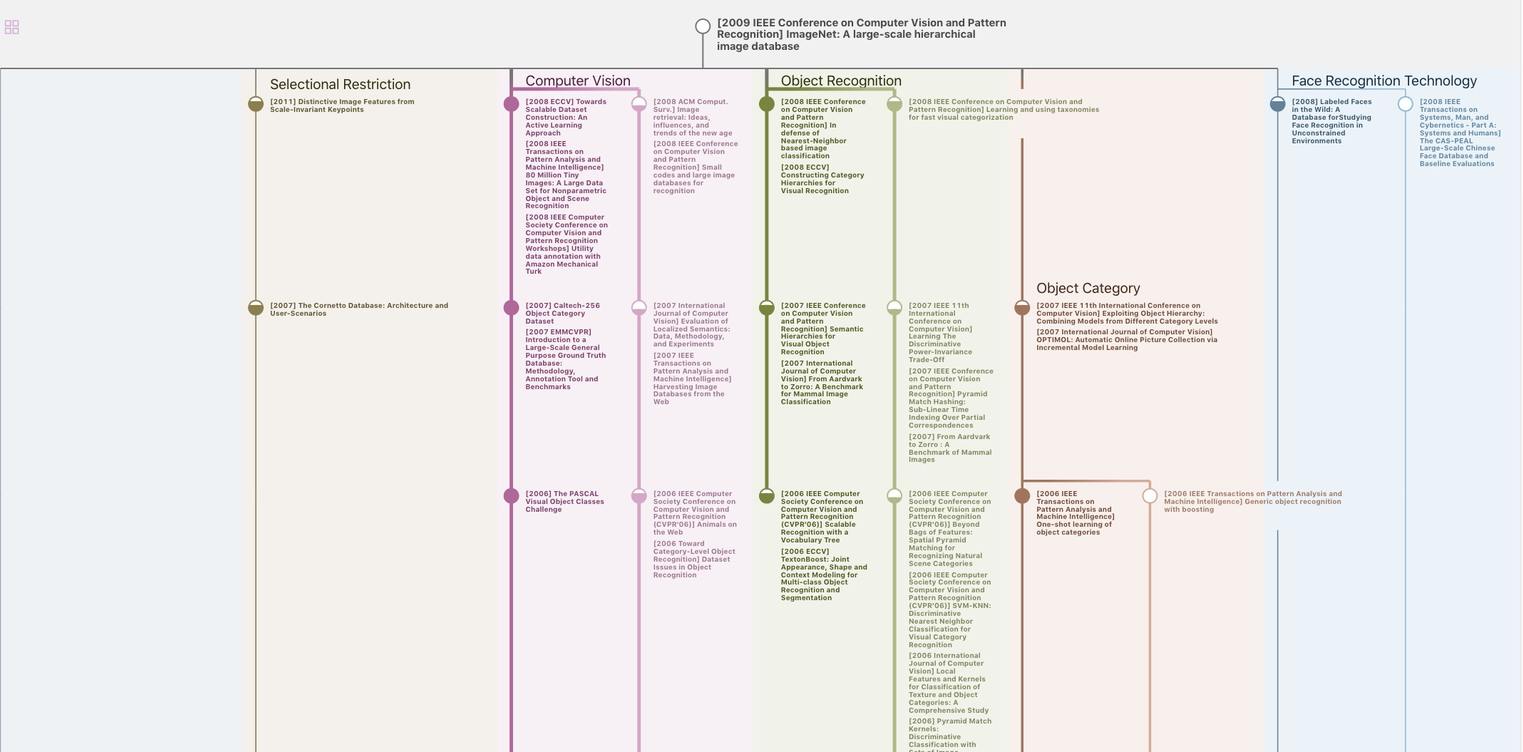
生成溯源树,研究论文发展脉络
Chat Paper
正在生成论文摘要