Exploration of Unknown Scalar Fields with Multifidelity Gaussian Processes Under Localization Uncertainty
2023 AMERICAN CONTROL CONFERENCE, ACC(2023)
摘要
Autonomous marine vehicles are deployed in oceans and lakes to collect spatio-temporal data. GPS is often used for localization, but is inaccessible underwater. Poor localization underwater makes it difficult to pinpoint where data are collected, to accurately map, or to autonomously explore the ocean and other aquatic environments. This paper proposes the use of multifidelity Gaussian process regression to incorporate data associated with uncertain locations. With the proposed approach, an adaptive sampling algorithm is developed for exploration and mapping of unknown scalar fields. The reconstruction performance based on the multifidelity model is compared to that based on a single-fidelity Gaussian process model that only uses data with known locations, and to that based on a single-fidelity Gaussian process model that ignores the localization error. Numerical results show that the proposed multifidelity approach outperforms both single-fidelity approaches in terms of the reconstruction accuracy.
更多查看译文
AI 理解论文
溯源树
样例
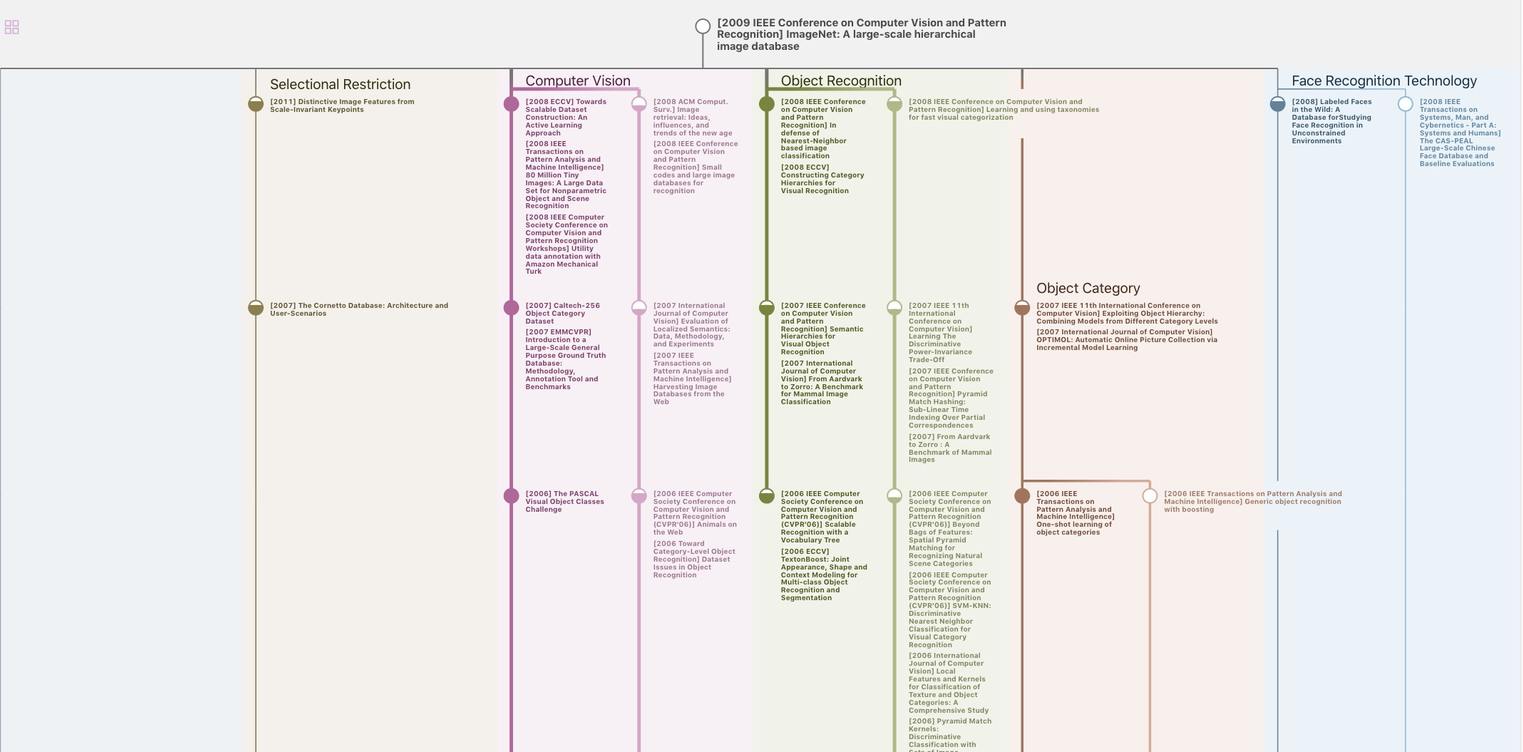
生成溯源树,研究论文发展脉络
Chat Paper
正在生成论文摘要