Tectonic-Shift: A Composite Storage Fabric for Large-Scale ML Training.
USENIX Annual Technical Conference(2023)
摘要
Tectonic-Shift is the storage fabric for Meta's production machine learning (ML) training infrastructure. Industrial storage fabrics for ML need to meet both the intensive IO and high-capacity storage demands of training jobs. Our prior storage fabric, Tectonic, used hard disk drives (HDDs) to store training data. However, HDDs provide poor IO-per-watt performance. This inefficiency hindered the scalability of our storage fabric, and thus limited our ability to keep pace with rapidly growing training IO demands. This paper describes our journey to build and deploy Tectonic-Shift, a composite storage fabric that efficiently serves the needs of our training infrastructure. We begin with a deep workload characterization that guided an extensive hardware and software design space exploration. We then present the principled design of Tectonic-Shift, which maximizes storage power efficiency by combining Shift, a flash storage tier, with Tectonic. Shift improves efficiency by absorbing reads using IO-efficient flash, reducing required HDD capacity. Shift maximizes IO absorption via novel application-aware cache policies that infer future access patterns from training dataset specifications. Shift absorbs 1.51- 3.28x more IO than an LRU flash cache and reduces power demand in a petabyte-scale production Tectonic-Shift cluster by 29%.
更多查看译文
AI 理解论文
溯源树
样例
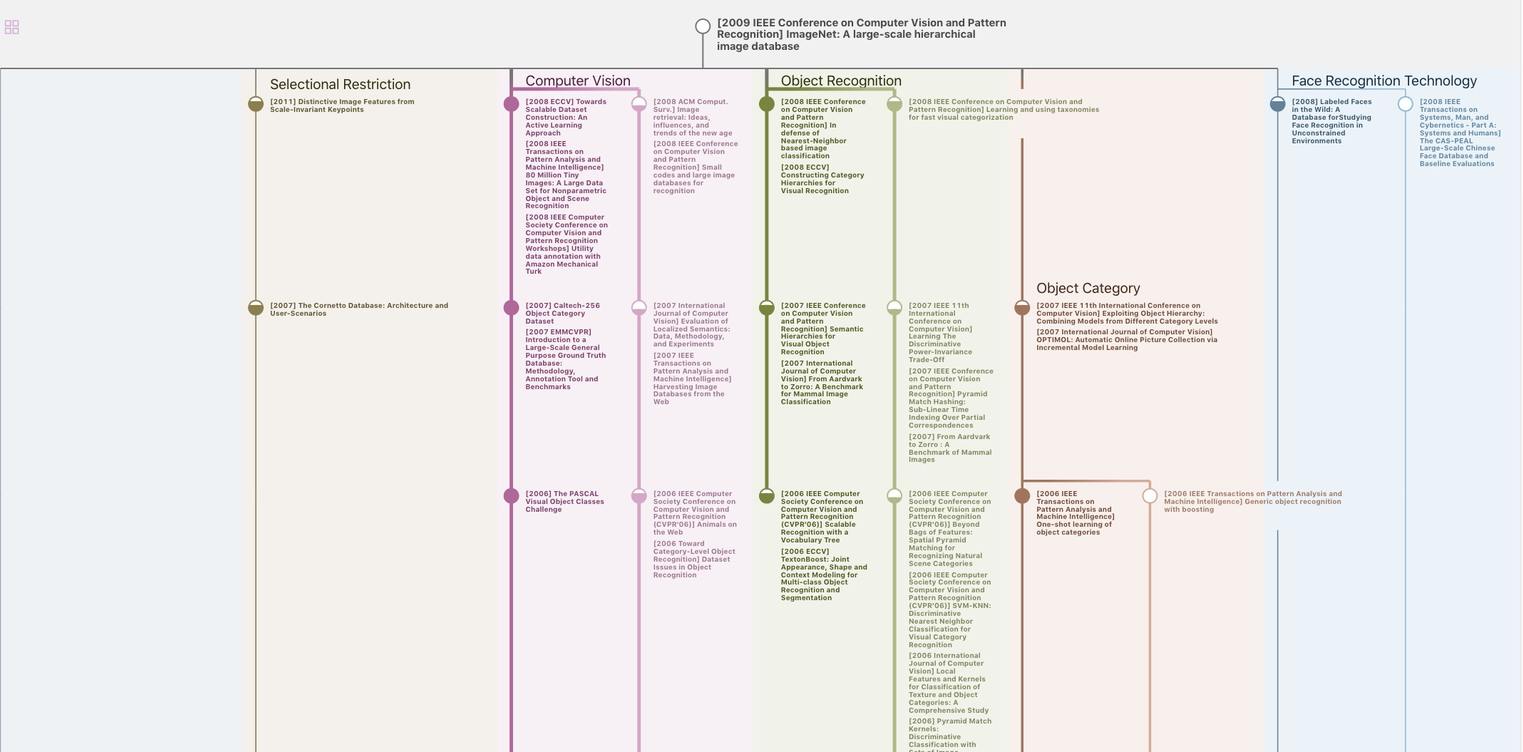
生成溯源树,研究论文发展脉络
Chat Paper
正在生成论文摘要