Game Theory for Autonomy: From Min-Max Optimization to Equilibrium and Bounded Rationality Learning
2023 AMERICAN CONTROL CONFERENCE, ACC(2023)
摘要
Finding Nash equilibria in non-cooperative games can be, in general, an exceptionally challenging task. This is owed to various factors, including but not limited to the cost functions of the game being nonconvex/nonconcave, the players of the game having limited information about one another, or even due to issues of computational complexity. The present tutorial draws motivation from this harsh reality and provides methods to approximate Nash or min-max equilibria in non-ideal settings using both optimization- and learning-based techniques. The tutorial acknowledges, however, that such techniques may not always converge, but instead lead to oscillations or even chaos. In that respect, tools from passivity and dissipativity theory are provided, which can offer explanations about these divergent behaviors. Finally, the tutorial highlights that, more frequently than often thought, the search for equilibrium policies is simply vain; instead, bounded rationality and non-equilibrium policies can be more realistic to employ owing to some players' learning imperfectly or being relatively naive - "bounded rational." The efficacy of such plays is demonstrated in the context of autonomous driving systems, where it is explicitly shown that they can guarantee vehicle safety.
更多查看译文
AI 理解论文
溯源树
样例
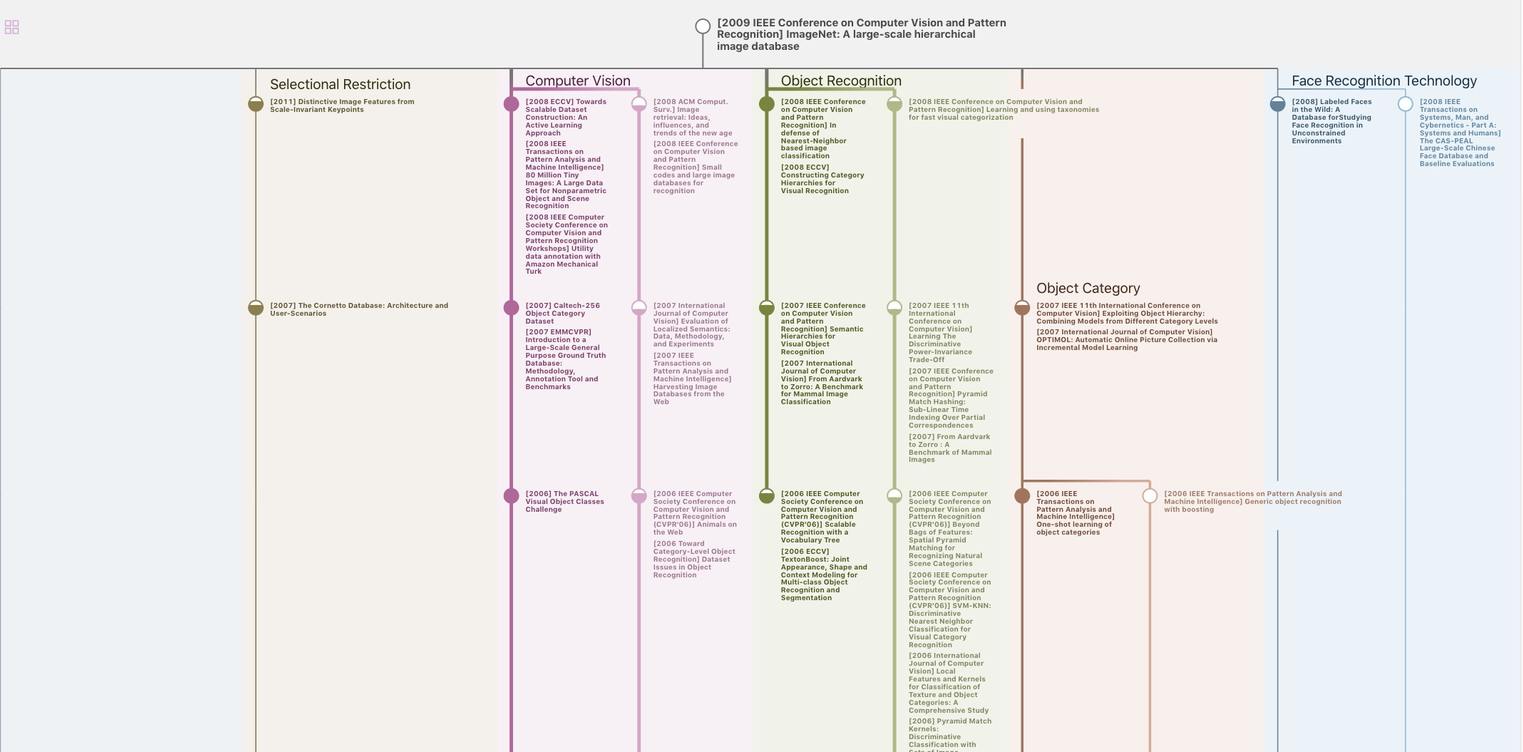
生成溯源树,研究论文发展脉络
Chat Paper
正在生成论文摘要