Convergent Second-Order Methods for Min-Max Optimizations
2023 AMERICAN CONTROL CONFERENCE, ACC(2023)
摘要
We address the use of second-order methods to solve optimizations of the form\begin{equation*}\mathop {\min }\limits_{u \in \mathcal{U}} \mathop {\max }\limits_{d \in \mathcal{D}} f(u,d),\tag{1}\end{equation*}for a twice continuously differentiable function $f:\mathcal{U} \times \mathcal{D} \to \mathbb{R}$ and sets $\mathcal{U} \subset {\mathbb{R}^{{n_u}}},\mathcal{D} \subset {\mathbb{R}^{{n_d}}}$. This type of optimization arises in numerous applications, including robust machine learning [1], model predictive control [2], [3], and in reformulating stochastic programming as a min-max optimizations [4], [5].When the sets $\mathcal{U}$ and $\mathcal{D}$ are compact and convex and the function f (u, d) is convex with respect to u and concave with respect to d, the min and max in (1) commute [6] and the optimization becomes relatively simple. However, we are especially interested here in problems for which such assumptions do not hold, the min and max do not commute, and for which the optimizations may have local optima that are not global.In this talk, we address the design of algorithms to solve nonconvex-nonconcave min-max optimizations like (1) using second order methods. These algorithms modify the Hessian matrix to obtain a search direction that can be seen as the solution to a quadratic program that locally approximates the min-max problem. We show that by selecting this type of modification appropriately, the only stable points of the resulting iterations are local min-max points. For min-max model predictive control problems, these algorithms leads to computation times that scale linearly with the horizon length.For more information please see the main tutorial paper [7].
更多查看译文
AI 理解论文
溯源树
样例
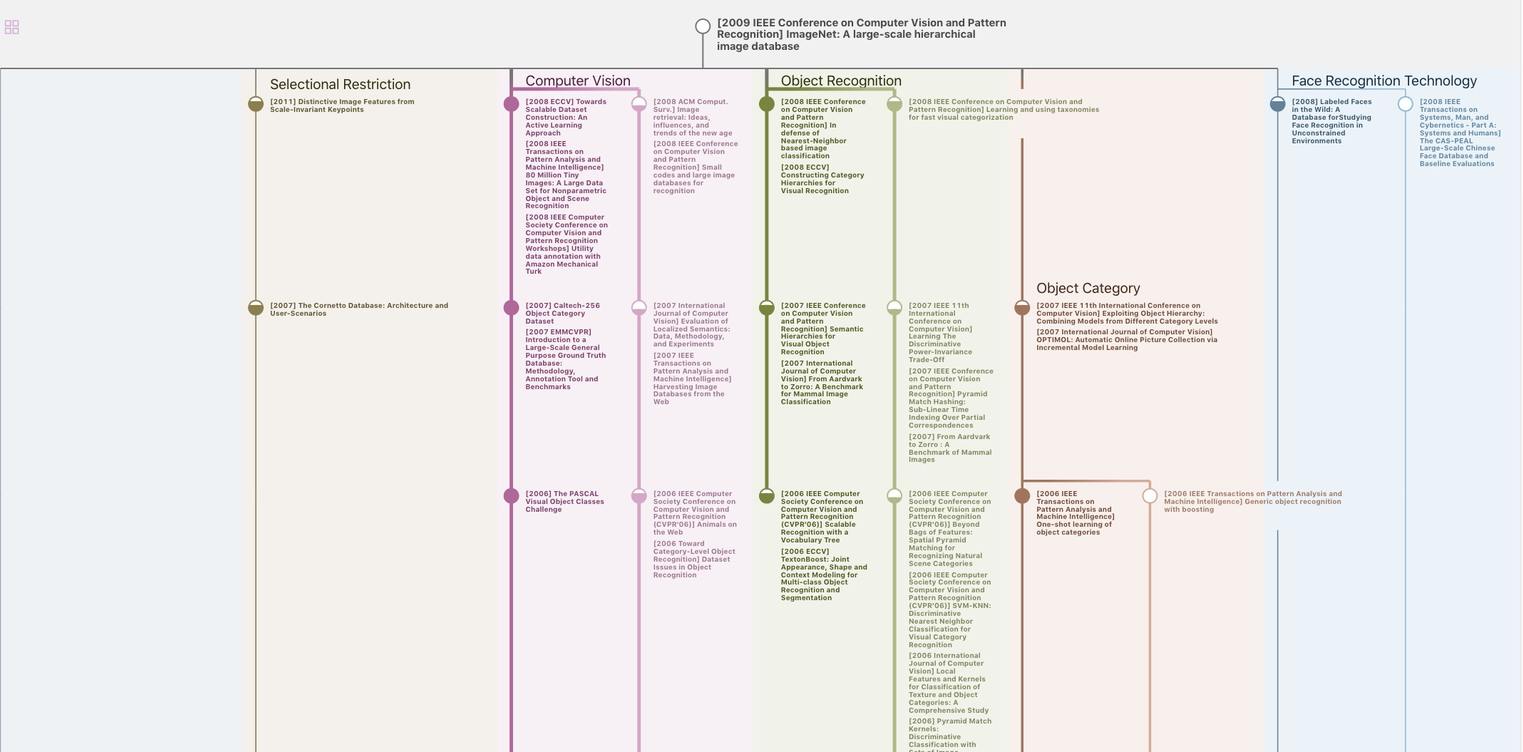
生成溯源树,研究论文发展脉络
Chat Paper
正在生成论文摘要