Safety Guaranteed Optimal Control Policy for Multi-agent Data Harvesting Using a CLF-CBF Approach.
2023 AMERICAN CONTROL CONFERENCE, ACC(2023)
Abstract
We consider the problem of harvesting data from a set of targets in a wireless sensor network using a collection of mobile agents. The targets lie in a one dimensional mission space and broadcast their data while the agents move overhead. The agents are required to collect all the data and move to terminal locations to offload that data. We use a Hamiltonian analysis to show that the optimal control can be described using a parameterized policy and then develop a gradient descent scheme using infinitesimal perturbation analysis (IPA) to calculate the gradients of the cost function with respect to the control parameters. To avoid collisions between agents, we then apply a Control Lyapunov Function-Control Barrier Function (CLF-CBF) technique to ensure the agents closely track the desired optimal trajectory to complete their mission while avoiding any collisions. Additionally, we analyze the problem of symmetric deadlock in the CLF-CBF controller and show that it can be avoided by adding a small perturbation to the initial and final heights of the agents over the mission space. The approach is demonstrated through simulation.
MoreTranslated text
Key words
Wireless Energy Harvesting,RF Energy Harvesting,Multi-Agent Systems,Cooperative Control,Distributed Optimization
求助PDF
上传PDF
View via Publisher
AI Read Science
AI Summary
AI Summary is the key point extracted automatically understanding the full text of the paper, including the background, methods, results, conclusions, icons and other key content, so that you can get the outline of the paper at a glance.
Example
Background
Key content
Introduction
Methods
Results
Related work
Fund
Key content
- Pretraining has recently greatly promoted the development of natural language processing (NLP)
- We show that M6 outperforms the baselines in multimodal downstream tasks, and the large M6 with 10 parameters can reach a better performance
- We propose a method called M6 that is able to process information of multiple modalities and perform both single-modal and cross-modal understanding and generation
- The model is scaled to large model with 10 billion parameters with sophisticated deployment, and the 10 -parameter M6-large is the largest pretrained model in Chinese
- Experimental results show that our proposed M6 outperforms the baseline in a number of downstream tasks concerning both single modality and multiple modalities We will continue the pretraining of extremely large models by increasing data to explore the limit of its performance
Upload PDF to Generate Summary
Must-Reading Tree
Example
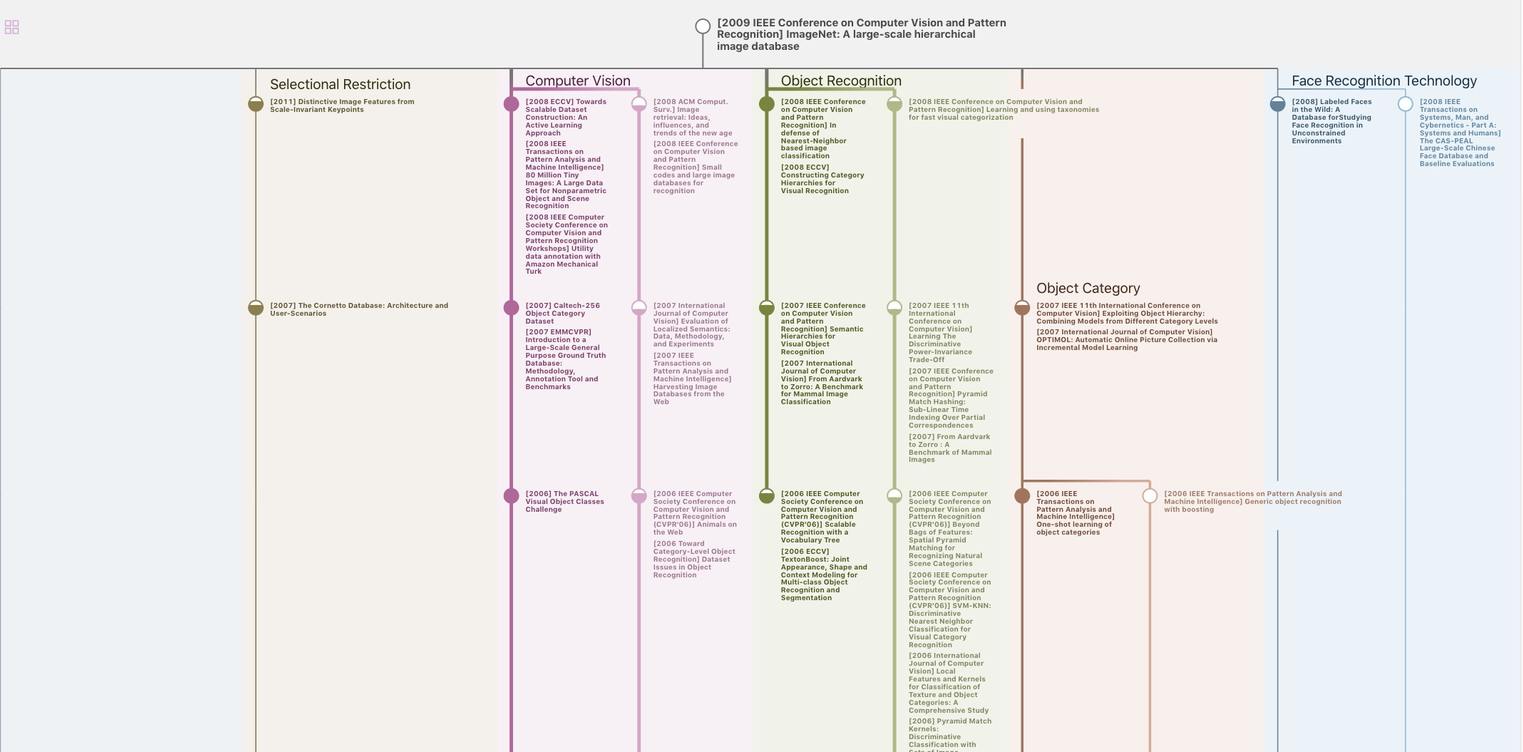
Generate MRT to find the research sequence of this paper
Related Papers
Guaranteed Stabilization and Safety of Nonlinear Systems Via Sliding Mode Control
IEEE CONTROL SYSTEMS LETTERS 2023
被引用0
Data Disclaimer
The page data are from open Internet sources, cooperative publishers and automatic analysis results through AI technology. We do not make any commitments and guarantees for the validity, accuracy, correctness, reliability, completeness and timeliness of the page data. If you have any questions, please contact us by email: report@aminer.cn
Chat Paper