Hybrid Controller Synthesis for Nonlinear Systems Subject to Reach-Avoid Constraints.
CAV (1)(2023)
摘要
There is a pressing need for learning controllers to endow systems with properties of safety and goal-reaching, which are crucial for many safety-critical systems. Reinforcement learning (RL) has been deployed successfully to synthesize controllers from user-defined reward functions encoding desired system requirements. However, it remains a significant challenge in synthesizing provably correct controllers with safety and goal-reaching requirements. To address this issue, we try to design a special hybrid polynomial-DNN controller which is easy to verify without losing its expressiveness and flexibility. This paper proposes a novel method to synthesize such a hybrid controller based on RL, low-degree polynomial fitting and knowledge distillation. It also gives a computational approach, by building and solving a constrained optimization problem coming from verification conditions to produce barrier certificates and Lyapunov-like functions, which can guarantee every trajectory from the initial set of the system with the resulted controller satisfies the given safety and goal-reaching requirements. We evaluate the proposed hybrid controller synthesis method on a set of benchmark examples, including several high-dimensional systems. The results validate the effectiveness and applicability of our approach.
更多查看译文
关键词
nonlinear systems,nonlinear systems subject,constraints,hybrid,synthesis,reach-avoid
AI 理解论文
溯源树
样例
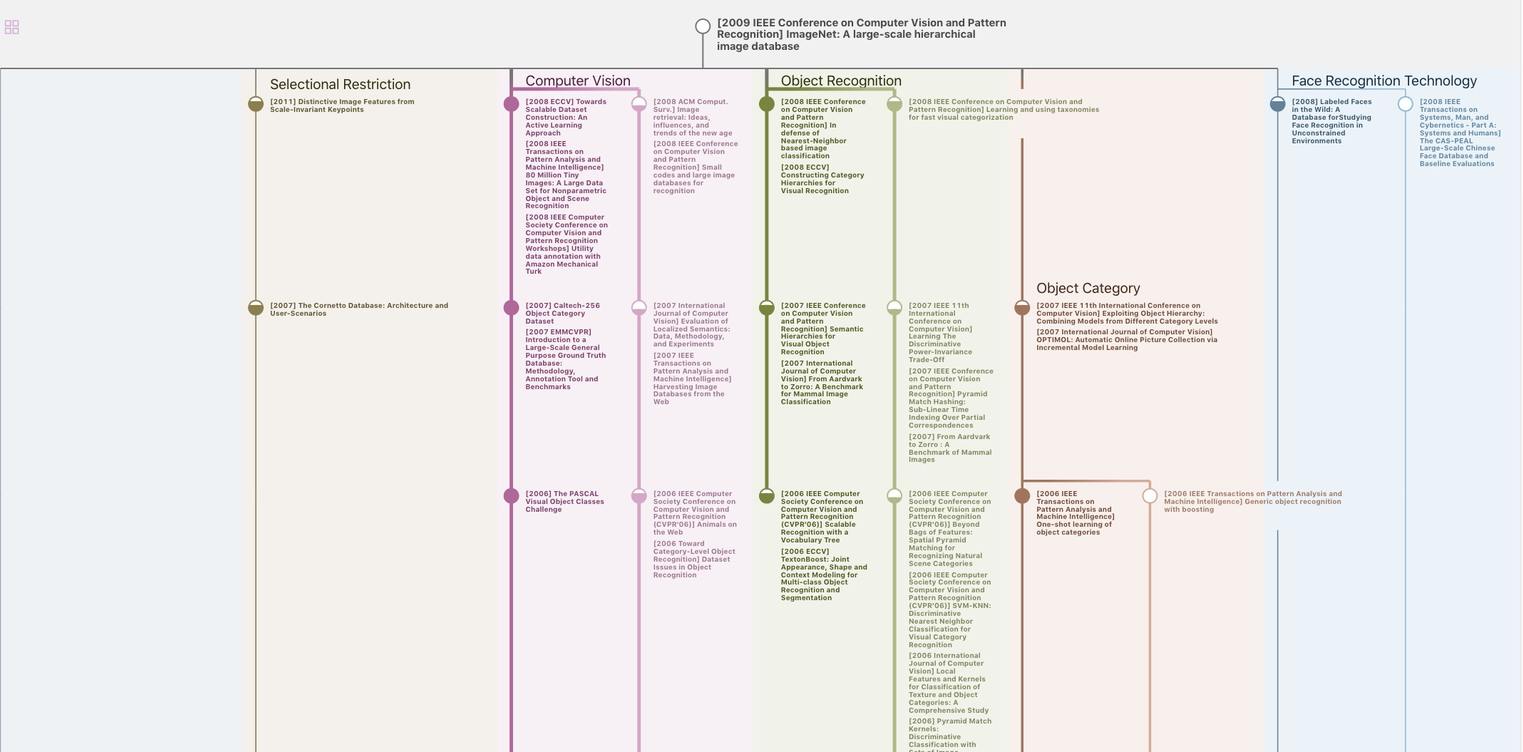
生成溯源树,研究论文发展脉络
Chat Paper
正在生成论文摘要