A 12-Nm 0.62-1.61 Mw Ultra-Low Power Digital CIM-based Deep-Learning System for End-to-End Always-on Vision.
2023 IEEE Symposium on VLSI Technology and Circuits (VLSI Technology and Circuits)(2023)
Abstract
This work proposes an ultra-low power DCIM-based DL system (DCIM-DLS) for end-to-end AoV with the power range from 0.62 to 1.61 mW (INT8, 2-15 fps). Compared to the prior art [3], the power consumption of DCIM-DLS can be reduced by 70.9% based on the following techniques: 1) an area and energy efficient DCIM that reduces compute RC loading by using pushed-rule 2p8T SRAM bitcell with folded kernels selector, 2) a DCIM-friendly dataflow strategy with dual accumulators that minimizes the DCIM power of weight update and avoids redundant data movement for power saving, and 3) a reconfigurable DCIM control scheme that supports mixed-precision to further reduce power consumption.
MoreTranslated text
Key words
2-15 fps,compute RC loading,DCIM power,DCIM-DLS,DCIM-friendly dataflow strategy,end-to-end AoV,mW ultra-low power digital CIM-based,power 0.62 mW to 1.61 mW,power consumption,pushed-rule 2p8T SRAM bitcell,reconfigurable DCIM control scheme,ultra-low power DCIM-based
求助PDF
上传PDF
View via Publisher
AI Read Science
AI Summary
AI Summary is the key point extracted automatically understanding the full text of the paper, including the background, methods, results, conclusions, icons and other key content, so that you can get the outline of the paper at a glance.
Example
Background
Key content
Introduction
Methods
Results
Related work
Fund
Key content
- Pretraining has recently greatly promoted the development of natural language processing (NLP)
- We show that M6 outperforms the baselines in multimodal downstream tasks, and the large M6 with 10 parameters can reach a better performance
- We propose a method called M6 that is able to process information of multiple modalities and perform both single-modal and cross-modal understanding and generation
- The model is scaled to large model with 10 billion parameters with sophisticated deployment, and the 10 -parameter M6-large is the largest pretrained model in Chinese
- Experimental results show that our proposed M6 outperforms the baseline in a number of downstream tasks concerning both single modality and multiple modalities We will continue the pretraining of extremely large models by increasing data to explore the limit of its performance
Upload PDF to Generate Summary
Must-Reading Tree
Example
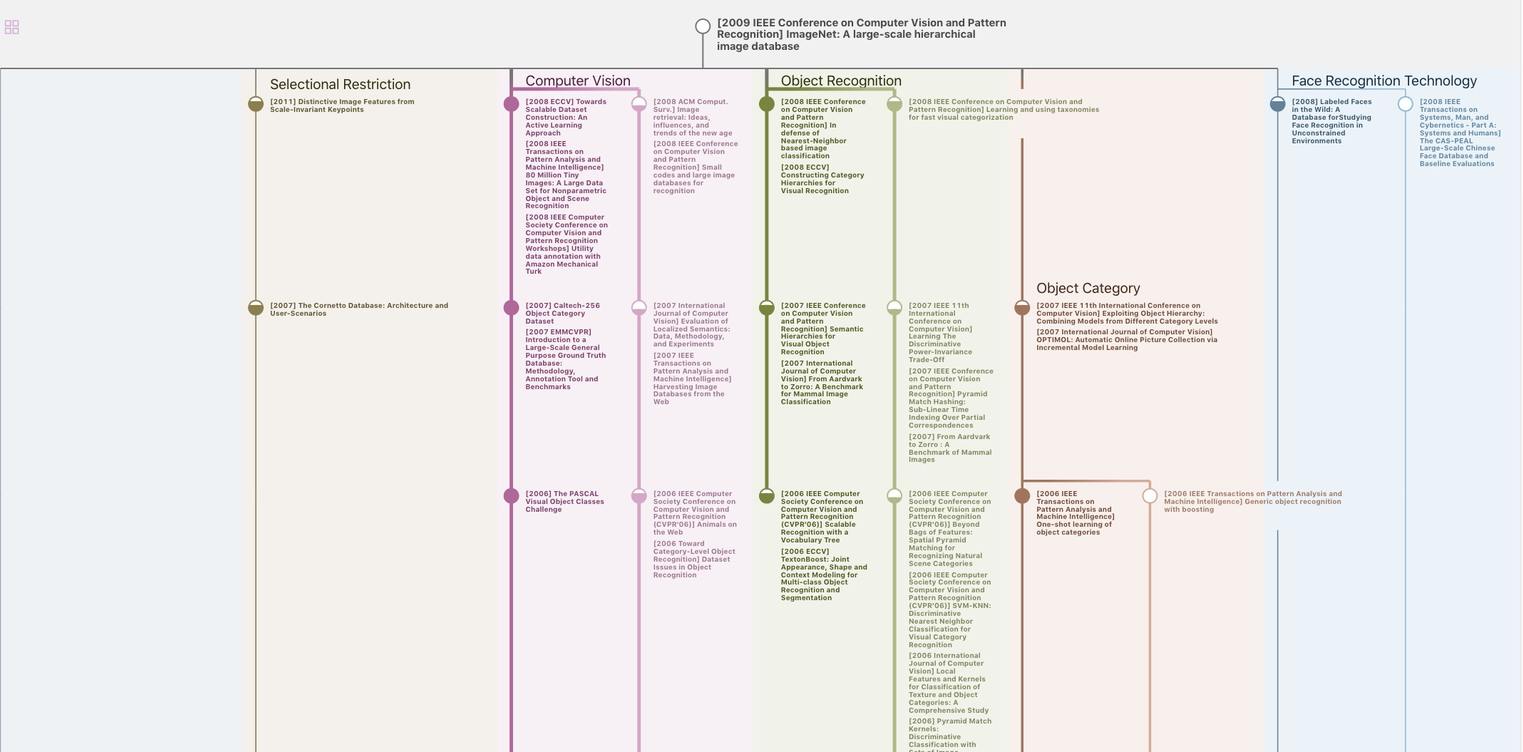
Generate MRT to find the research sequence of this paper
Data Disclaimer
The page data are from open Internet sources, cooperative publishers and automatic analysis results through AI technology. We do not make any commitments and guarantees for the validity, accuracy, correctness, reliability, completeness and timeliness of the page data. If you have any questions, please contact us by email: report@aminer.cn
Chat Paper