In-network Latency Nowcast Using Data Stream Learning Models
IWCMC(2023)
摘要
Predicting network metrics with high accuracy is a challenging task. Network Latency prediction allows Network Operators (NO), Internet Service Providers (ISP), and Over The Top (OTT) Service Providers to optimize their performance in almost real-time. Moreover, applications can make decisions based on latency predictions, improving the quality of the provided services. In recent years, many works proposed machine learning based techniques to predict network metrics, especially using Recurrent Neural Network (RNN) techniques, such as Long Short-Term Memory (LSTM) Networks. Nevertheless, despite the good results achieved, the computational cost of training and keeping a model updated makes adopting those techniques unfeasible in some scenarios. In this work, we propose the usage of Data Stream Learning techniques to predict Round-Trip Time (RTT) and One-Way Delay (OWD) metrics using real-world data. The experiment results demonstrated a prediction performance similar to LSTM networks using only a fraction of the computational resources used by LSTM.
更多查看译文
关键词
Data Stream Learning,Machine Learning,One-Way Delay (OWD) prediction,Online Learning,Round-Trip Time (RTT) Prediction
AI 理解论文
溯源树
样例
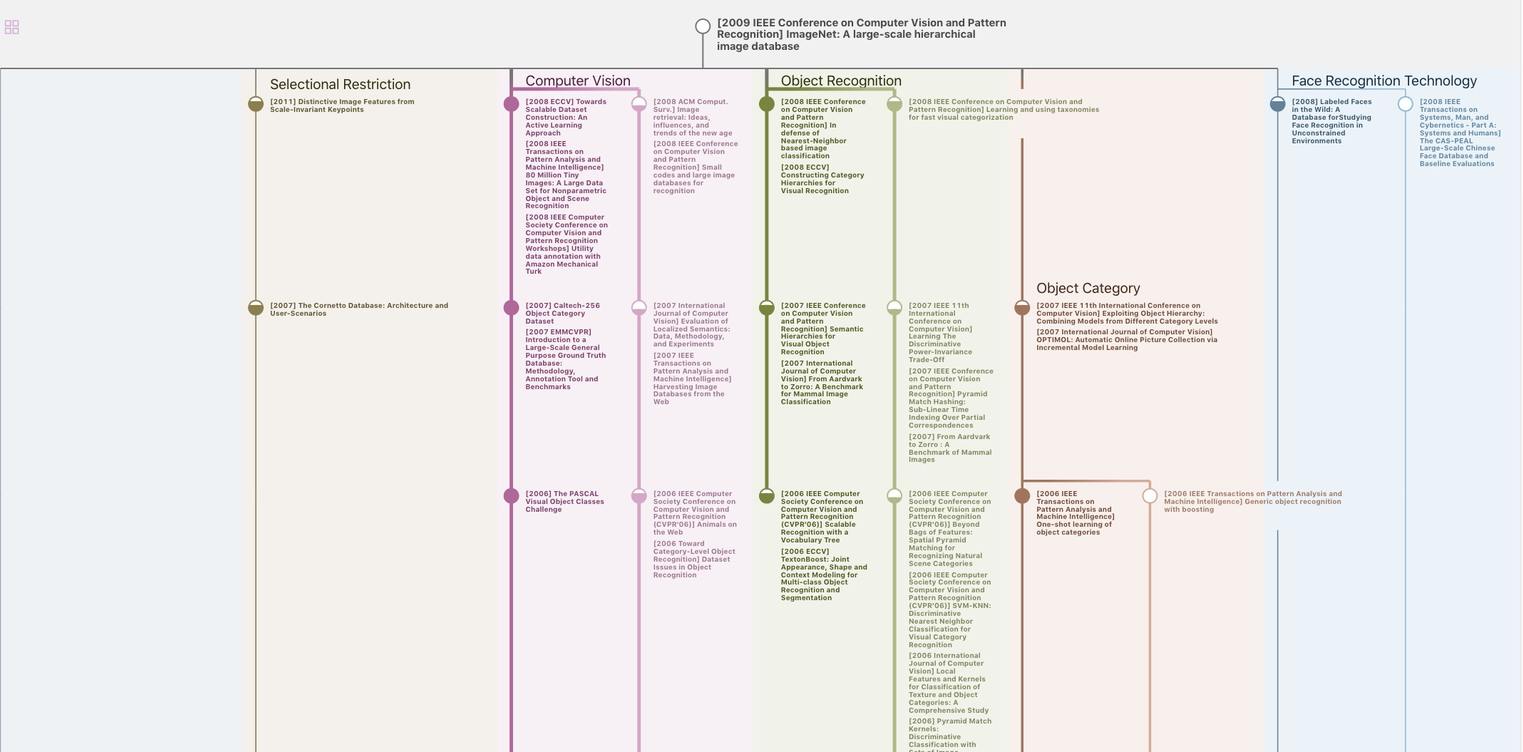
生成溯源树,研究论文发展脉络
Chat Paper
正在生成论文摘要