A Holistic Approach for Answering Logical Queries on Knowledge Graphs
ICDE(2023)
摘要
Logical queries on Knowledge Graphs (KGs) is a fundamental sub-task of knowledge graph reasoning. A promising paradigm for answering logical queries, recently, has been proposed based on versatile deep learning techniques. In this line, the query is first broken down into a series of first-order logical predicates, and then both the query and knowledge graph entities are jointly encoded in the same embedding space. Some approaches are able to support the full range of traditional First-Order Logic (FOL) operations for complex queries in real-world scenarios, while others have attempted to create a new combination of FOL operations by replacing the negation operation with the difference operation due to the poor performance of the negation operation. Our empirical observations show that the difference operator is more effective for multi-hop reasoning, while the negation operator is better suited for use as the final operation in the query, particularly in single-hop settings. In addition, other fundamental limitations such as linear transformation assumption for negation operator and the fixed-lossy problem for difference operator further degrade the performance of these methods. In light of these, we propose the HaLk, a holistic approach for answering logical queries that, to our knowledge, is the first to support a full set of logical operators in a unified end-to-end framework. In this approach, we propose specific neural models for each operator by considering their own intrinsic properties, based on which HaLk effectively mitigates the cascading error of projection and negation operators as well as delicately provides closed-formed solutions for difference operator. Extensive experimental results on three datasets demonstrate that HaLk outperforms all competitors and achieves up to 32% improvement in accuracy.
更多查看译文
关键词
Logical queries,knowledge graph,geometry deep learning
AI 理解论文
溯源树
样例
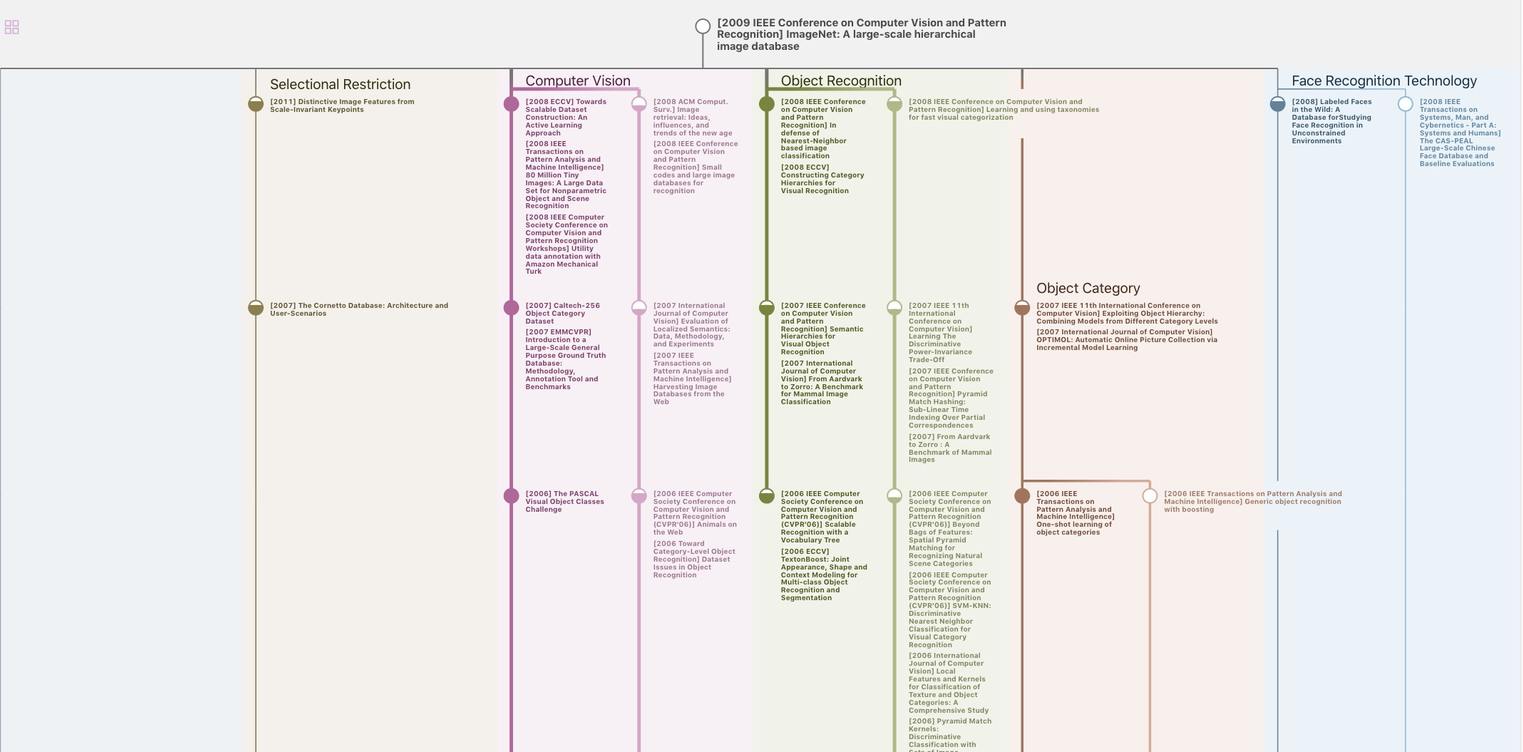
生成溯源树,研究论文发展脉络
Chat Paper
正在生成论文摘要