Bipartite Graph based Multi-view Clustering (Extended Abstract).
ICDE(2023)
摘要
In existing graph-based multi-view clustering algorithms, consensus cluster structures are explored by constructing similarity graphs of multiple views and then fusing them into a unified superior graph. However, they overlook consensus information when learning each graph independently, resulting in the undesirable unified graph with biases. To this end, we proposed a framework named bipartite graph based multi-view clustering (BIGMC) in [1] to tackle this challenge. To summarize, the key idea of BIGMC is to employ a small number of uniform anchors to represent the consensus information across views. In this way, BIGMC creates a bipartite graph between data points and anchors for each view, which are then fused to generate a unified bipartite graph. The unified graph would in turn improve each view bipartite graph and the anchor set. Finally, the clusters are formed directly using the unified graph. In this extended abstract, we also summarize the effectiveness of BIGMC as shown in experimental results originally presented in [1].
更多查看译文
关键词
Multi-view clustering,consensus information,bipartite graph
AI 理解论文
溯源树
样例
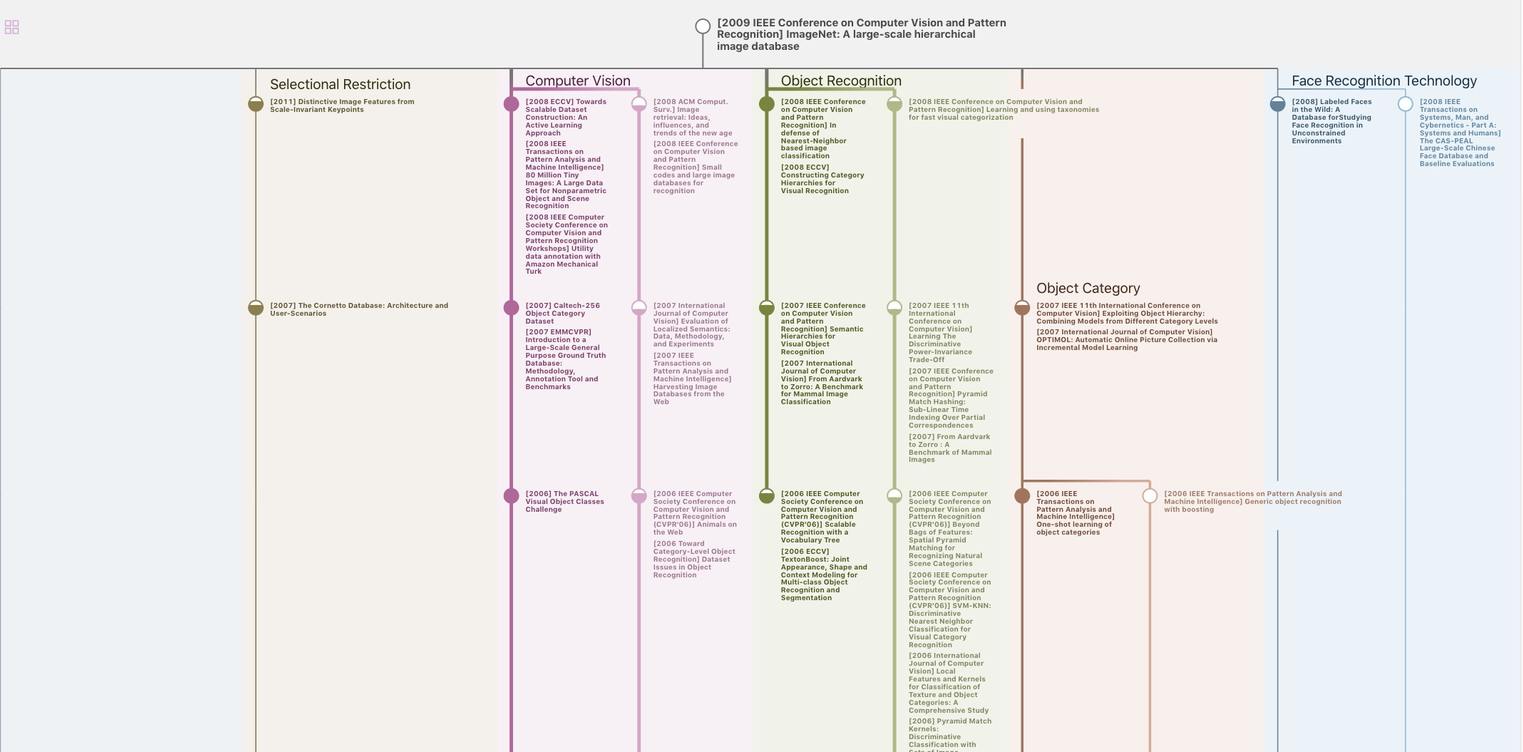
生成溯源树,研究论文发展脉络
Chat Paper
正在生成论文摘要