An Atomic Retrospective Learning Bare Bone Particle Swarm Optimization.
ICSI (1)(2023)
摘要
In order to increase the diversity of bare-bone particle swarm optimization (BBPSO) population search range, enhance the ability to jump out of local optimum, we propose an atomic retrospective learning bare-bone particle swarm optimization (ARBBPSO) algorithm based on BBPSO. Different from the renewal strategy of BBPSO, inspired by electron motion around protons in ARBBPSO, we use a strategy of motion around nuclei to increase population diversity. At the same time, the retrospective learning strategy is used to allow the proton particles to have a chance to correct errors during the process of updating, thus allowing the population to have a chance to evade falling into a local optimum. To verify the performance of the proposed algorithm, 29 benchmark functions of CEC2017 are chosen to compare with four well-known BBPSO-based algorithms. The experimental results indicate that ARBBPSO is superior to several other algorithms for improving BBPSO from a comprehensive consideration.
更多查看译文
关键词
particle swarm optimization,bone
AI 理解论文
溯源树
样例
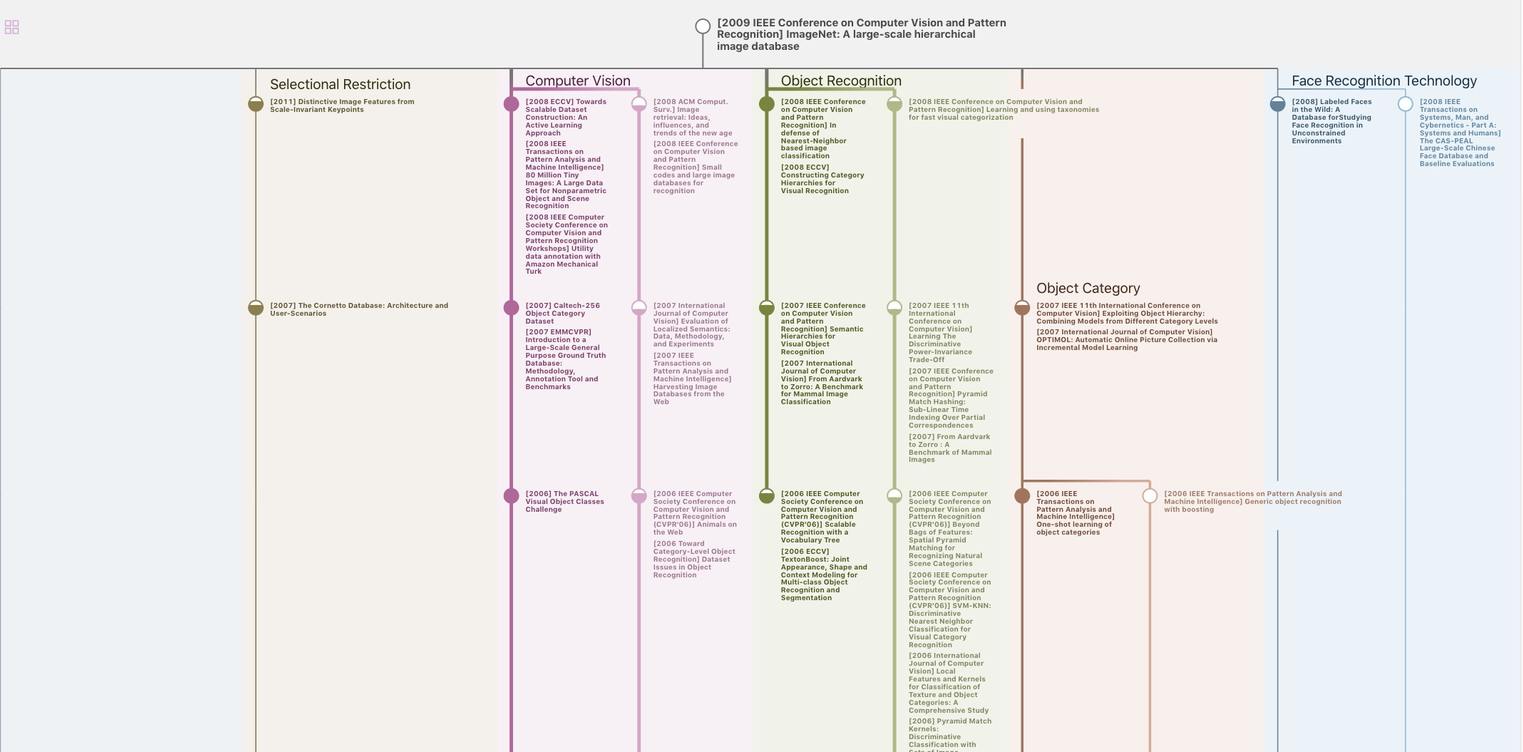
生成溯源树,研究论文发展脉络
Chat Paper
正在生成论文摘要