A Novel Labeling Scheme for Neural Belief Propagation in Polar Codes
IWCMC(2023)
摘要
Recently, deep learning has been adopted to improve the performance of the belief propagation algorithm in polar codes, namely, the neural belief propagation decoder. By attaching trainable parameters to each edge on a tanner graph, this decoder effectively weakens the effect of short cycles. In this paper, we will show that the decoder can be further improved with our newly-designed labeling scheme. Instead of ground-truth transmitted codewords, our scheme utilizes codewords generated from a high-performance decoder (i.e., noise-aided belief propagation list decoder) to construct loss functions. Besides, to reduce the labeling complexity, the ground-truth transmitted codewords are integrated into the decoder as a list and compared with the other lists with the principle of minimum Euclidean distance. Numerical simulation results demonstrate that the proposed labeling scheme can improve the decoding performance by up to 0.2 dB with the same run time complexity and model size.
更多查看译文
关键词
Polar codes,deep learning,belief propagation algorithm
AI 理解论文
溯源树
样例
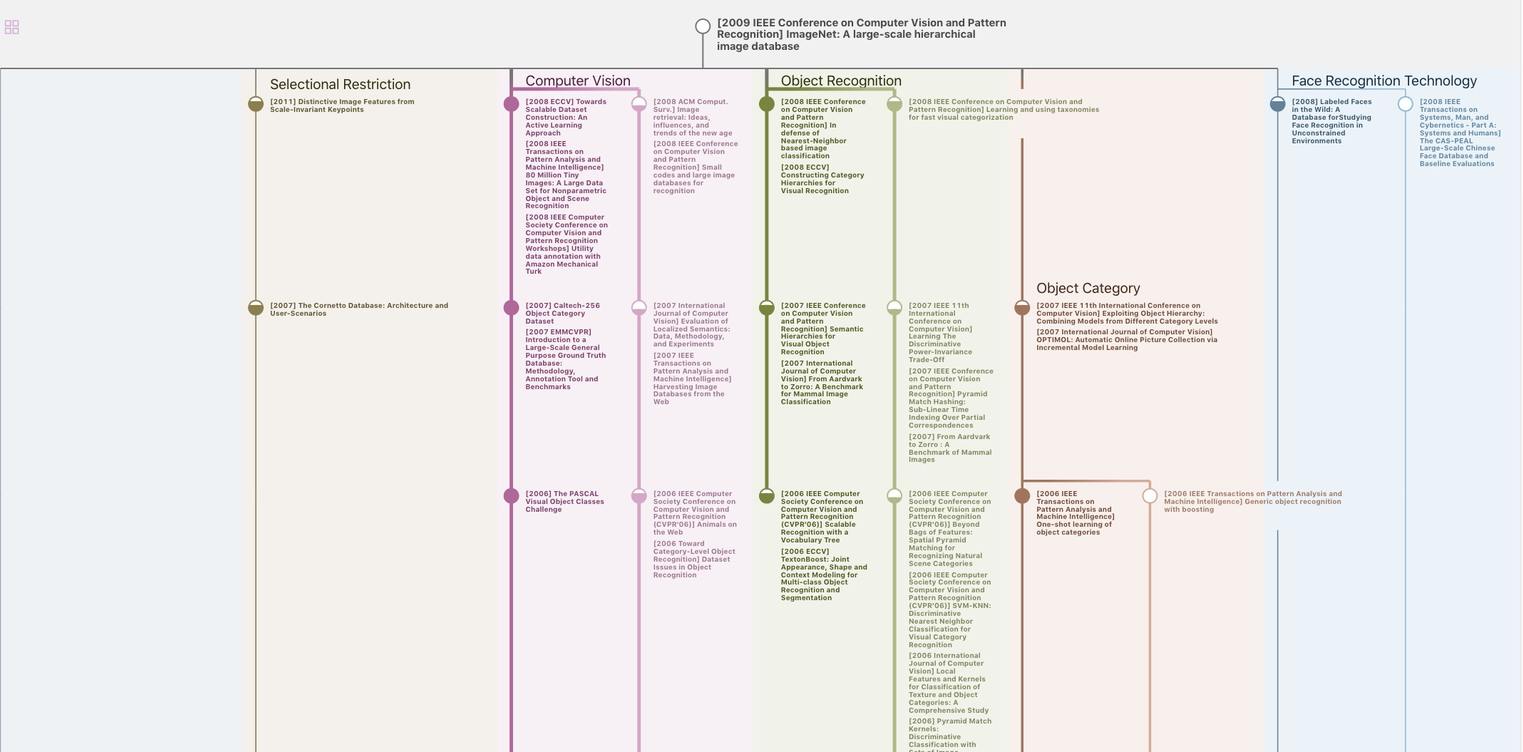
生成溯源树,研究论文发展脉络
Chat Paper
正在生成论文摘要